Effectiveness of a voice analysis technique in the assessment of depression status of individuals from Ho Chi Minh City, Viet Nam: A cross-sectional study
Volume 9, Issue 4, Page No 73-78, 2024
Author’s Name: Le Truong Vinh Phuc 1,2, Mituteru Nakamura3, Masakazu Higuchi3, Shinichi Tokuno1,3
View Affiliations
1Graduate School of Health Innovation, Kanagawa University of Human Services, Kanagawa, 241-0815, Japan.
2University of Medicine and Pharmacy at Ho Chi Minh City, Ho Chi Minh City, 749000, Vietnam.
3Voice Analysis and Measurement of Pathophysiology, Department of Bioengineering, Graduate School of Engineering, The University of Tokyo, Tokyo, 113-0033, Japan.
a)whom correspondence should be addressed. E-mail: vinhphuc0104@gmail.com
Adv. Sci. Technol. Eng. Syst. J. 9(4), 73-78 (2024); DOI: 10.25046/aj090408
Keywords: Mind Monitoring System, Mental health assessment, Depression, Voice analysis, Cross-sectional study
Export Citations
The Mind Monitoring System (MIMOSYS) is a novel voice analysis technique for mental health assessment that has been validated in some languages; however, no research has been conducted on the Vietnamese yet. This study aimed to examine the ability of the Vitality score extracted from the MIMOSYS system to assess depression status based on the standards of the Patient Health Questionnaire-9 items (PHQ-9) and Beck’s Depression Inventory (BDI) questionnaire using the Vietnamese language. In this cross-sectional study conducted between August 1, 2022 and September 4, 2022, students and staff from a university, and patients from a hospital were recruited. Participants were asked to complete the self-administered depression questionnaires (PHQ-9 and BDI), and their voice data was collected by reading designated sentences. MIMOSYS extracted the Vitality score from the participant’s voice data. One hundred and twenty-two participants with a mean age of 31 years were included in the study; 72.4% of them were female. After adjusting for age and sex, negative correlations between the Vitality score and psychological test scores were found. For discriminating individuals with a high risk of depression, using the BDI score as a standard, the area under the curve of the Vitality score was 0.72. Sensitivity, specificity, and accuracy evaluations also reported a moderate discrimination ability of the Vitality score on the risk of depression by the BDI. In conclusion, voice analysis can be a viable technique for depression assessment in Vietnamese; however, further investigations are necessary to confirm our findings.
Received: 04 June 2024, Revised: 29 July 2024, Accepted: 01 August 2024, Published Online: 03 August 2024
1. Introduction
Despite the fact that mental health disorders are recognized as an important global public health problem, mental health care remains a low priority in low- and middle-income countries [1], [2]. The Vietnamese Ministry of Health reported that the prevalence of depression and anxiety in the population is 2.8% and 2.6%, respectively [3]. Conventional methods, such as the use of self-administered questionnaires, are still effective for assessing..depression [4] and have served as the gold standard for evaluating mood and emotional issues [5]. However, since patients can provide incorrect information in interviews and questionnaires, objective indicators are perceived to be more useful for diagnostic support [6]. Voice analysis was gradually applied to health monitoring as a non-invasive technique, which requires no specialized equipment and can be performed easily and remotely. In several languages, such as Japanese, English, and German, physical characteristics of voice such as pitch rate, jitter, shimmer, and Harmony to Noise Ratio were examined for their capability to discriminate between healthy individuals and those with mental disorders [7].
Recently, a voice analysis system named the Mind Monitoring System (MIMOSYS) is in practical use to assess and monitor an individual’s mental health status, particularly in Japan [8], [9], [10]. The significant difference in mean Vitality score, the short-term indicator of MIMOSYS, between the high-risk and low-risk depression groups was also confirmed by research on the Romanian and Russian languages (p-value < 0.05) [11]. Therefore, this voice analysis technique can be a potential tool to assess depression because it has the advantages of overcoming the barrier of languages, eliminating the bias of psychological tests, and being simple for implementation and management, which may help reduce the burden on the screening and diagnostic process. To our knowledge, no similar research has been conducted on the Vietnamese language yet. This study aimed to 1) examine the correlation between the Vitality score extracted from the MIMOSYS system and the depression scores measured by the Patient Health Questionnaire-9 items (PHQ-9), and the Beck’s Depression Inventory (BDI), using the Vietnamese language, and 2) evaluate the performance of the Vitality score in discriminating individuals with a high risk of depression using the thresholds of the PHQ-9 and BDI as standards.
2. Methods
2.1. Ethics statements
The study protocol was approved by the Ethics Committee of the Graduate School of Health Innovation, Kanagawa University of Human Services (No. SHI-03), and by the Ethics Committee in Biomedical Research of the University of Medicine and Pharmacy at Ho Chi Minh City, Viet Nam (No. 397/HDDD-DHYD). All methods were performed in accordance with the guidelines stipulated in the Declaration of Helsinki. Written informed consent was obtained from each participant before data collection. The STROBE guidelines were followed in reporting the study.
2.2. Participants
This cross-sectional study was conducted from August 1, 2022 to September 4, 2022 and recruited individuals from the Faculty of Public Health, University of Medicine and Pharmacy at Ho Chi Minh City, and outpatients visiting the Department of Clinical Psychology, Le Van Thinh Hospital in Ho Chi Minh City, Viet Nam. To be eligible for study inclusion, individuals must be 18 years or older at the time of the study, have Vietnamese nationality, and use Vietnamese as their native language. Additionally, at the hospital, outpatients had to be diagnosed with major depressive disorder according to the Diagnostic and Statistical Manual of Mental Disorders, Fourth Edition (DSM-IV) criteria by a psychologist. Individuals with speech problems, such as apraxia, dysarthria, orofacial myofunctional disorders, stuttering, or aphasia, those with congenital hearing impairment, or those with confirmed organic brain diseases, were excluded from our study. Data was collected in a separate room, with one participant interviewed each time.
Altogether, 125 potential eligible individuals were approached, including 100 students and staff from the school and 23 depressive patients from the hospital. Two patients were younger than 18 years old; thus, they were excluded from our study. A total of 123 participants were finally included in our analysis (Figure 1).
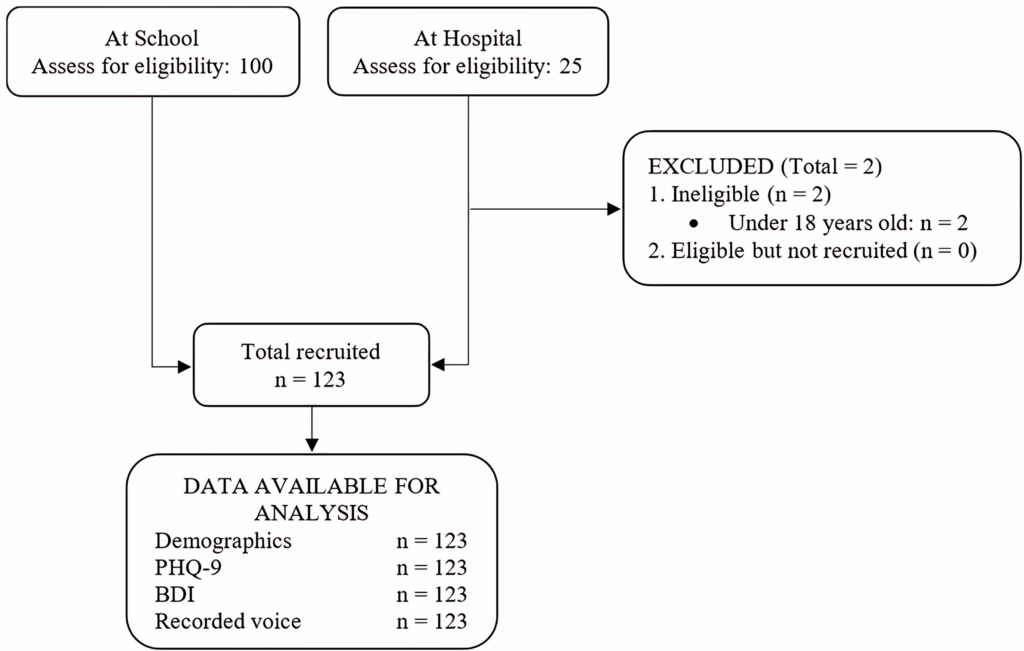
2.3. Voice data
A sheet of sixteen designated phrases used for recording voice was provided. The participant’s voice was recorded by reading out loud the phrases one by one that followed the signal of the data collector. Phrases were read in a constant order for all participants. Phrases used in this study were conventionally translated into Vietnamese based on the meaning of the original Japanese phrases and in comparison with the English version of the phrases. Phrases were also categorized into two groups: reading phrases (phrases with full sentences) and vowel sounds (/Ah/, /Eh/, and /Uh/ phrases). Participant’s study ID number was also included in the recording to match their response in paper-based questionnaires.
Voice recordings were acquired at 24-bit and 96 kHz resolution using a TASCAM DR-100MK3 portable digital audio recorder (Tokyo, Japan) and an Olympus ME52W lavalier microphone (Tokyo, Japan). For the speech analysis, we used MIMOSYS (PST Inc., Kanagawa, Japan), a system used for monitoring health status through voice based on the sensibility technology [12]. For the requirements of the MIMOSYS data input format, the recorded speech was converted into 11 kHz, 16-bit audio data for analysis. The Adobe® Audition™ software version 1.5 (Adobe Inc., San Jose, California) was used for the audio conversion.
In brief, using MIMOSYS, the degree of intensity of each of the four emotional components (“calmness”, “anger”, “joy”, and “sorrow”) is firstly calculated from the voiced speech on an eleven-point scale of zero to 10. On a ten-point scale of one to ten, MIMOSYS also calculates the intensity of “excitement.” The intensity of these five variables is then used to calculate “vivacity” and “relaxation”. “vivacity” is determined by the components of “joy” and “sorrow”, whereas “relaxation” is determined by the components of “calmness” and “excitement”. Finally, “vivacity” and “relaxation” are used to calculate “Vitality” [7]. The data calculation flow of MIMOSYS is presented in Figure 2 [8]. The output, Vitality score, is a real numeric value ranging from zero to one. A higher Vitality score indicates a better mental state. In addition, the mean and variation in Vitality over two weeks can be used to generate the “Mental Activity” indicator [13].
In this study, the Vitality was characterized as a scale on which individuals suffering from depression got a lower score, and healthy individuals got a higher score. The Vitality score of each phrase and each combination of phrases was used to examine the study objectives. The combination that provided the overall best results was reported.
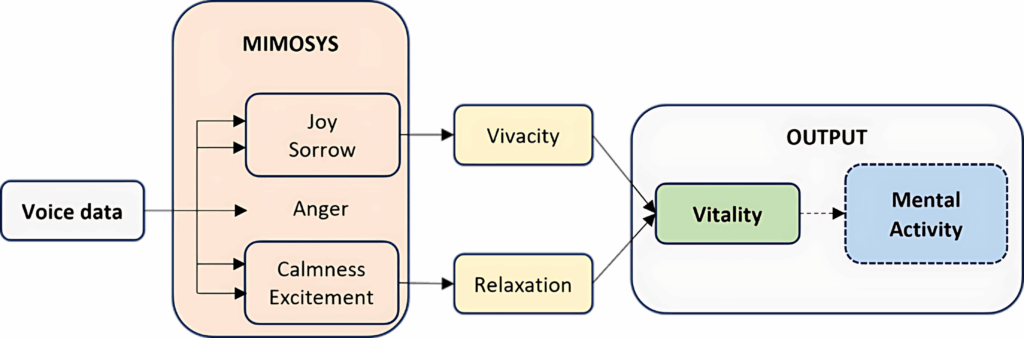
2.4. Self-administered questionnaires
Participants were asked to complete self-administered questionnaires to determine their depressive symptoms. The questionnaires used were the PHQ-9 and BDI in Vietnamese. These questionnaires are frequently used assessment instruments for depression, freely accessible, and the Vietnamese version of these tools is also available [14], [15]. The sum scores of the PHQ-9 and BDI tests were included in our analysis as dependent variables. The score ranges from 0 to 27 for the PHQ-9 and 0 to 63 for the BDI; a lower score indicates a milder depression status. The PHQ-9 and BDI cutoff scores of 12 [8] and 19 [15], respectively, were used to define an individual with a high risk of depression. The factors considered as potential effect modifiers were age and sex.
2.5. Data analysis
Pearson’s correlation analysis was applied to evaluate the correlation between the Vitality score and PHQ-9 or BDI score. The strength of the correlation was categorized as follows based on the absolute correlation coefficient: 0.5–1, strong correlation; 0.3–<0.5, moderate correlation; and <0.3, weak correlation. T-test analysis was applied to evaluate the difference in Vitality score between the low-risk and high-risk groups of depression categorized by the PHQ-9 or BDI sum score.
The area under the curve (AUC) from the receiver-operating characteristics (ROC) model using logistic regression was applied to examine the classification performance of the Vitality score. The performance was judged according to the AUC value: 0.5–< 0.7 as low accuracy, 0.7–< 0.9 as moderate accuracy, and 0.9–1 as high accuracy [6]. Our ROC models used a BDI score threshold of 19 and a PHQ-9 score threshold of 12. The ROC curve was plotted with sensitivity as the vertical axis and 1–specificity as the horizontal axis by altering the threshold of the Vitality score.
The sensitivity, specificity, and accuracy of the optimal Vitality cutoff score using the PHQ-9 or BDI as the standard were also reported. The optimal cutoff point for the Vitality score was specified with 1) a sensitivity value greater than 0.5, 2) a specificity value greater than 0.5, and 3) the value of Youden’s J index (sensitivity + specificity – 1) being the highest.
All the statistical tests were considered significant at α level of 5%. The online version of the SAS program was used to analyze and visualize the data.
3. Results
The participants’ mean age was 31 years, and 89 of them were female (72%). Most of the participants lived in Ho Chi Minh City (93%) (Table 1).
Table 1: Paritipants’ baseline characteristics (n=123)
Characteristics | Results |
Age (years), mean ± sd | 31 ± 12 |
Sex (female), n (%) | 89 (72) |
Residence (Ho Chi Minh City), n (%) | 114 (93) |
History of depression (Yes), n (%) | 30 (24) |
sd: standard deviation
The average scores in the PHQ-9 and the BDI tests among the participants were 6.9 ± 5.5 and 10.5 ± 9.4 points, respectively. The number of participants at high risk of depression was 19 and 20 based on the PHQ-9 cut and BDI cutoff scores (Table 2).
Table 2: Results of the PHQ-9 and BDI tests (n=123)
Psychological tests | Results |
PHQ-9 score, mean ± sd | 6.9 ± 5.5 |
High risk of depression, n (%) | 19 (15.5) |
BDI score, mean ± sd | 10.5 ± 9.4 |
High risk of depression, n (%) | 20 (16.3) |
sd: standard deviation
3.1. Correlation between the Vitality and test scores
The vitality score and correlation coefficient of each phrase are presented in Table 3. Mean Vitality score ranged from 0.36 to 0.56, Not all phrases had a negative correlation and/or significant result with the psychological tests (Table 3).
Table 3: Vitaltiy score and correlation of each phrase (n=123)
Phrase |
Vitality mean (sd) |
Correlation | |
PHQ-9 | BDI | ||
A B C D E F G | 0.36 (0.12) | −0.049 | −0.099 |
Alpha beta gamma delta | 0.44 (0.13) | −0.021 | −0.011 |
It is a sunny day today | 0.46 (0.16) | −0.020 | 0.005 |
Once upon a time | 0.42 (0.13) | −0.038 | 0.012 |
Galapagos Islands | 0.46 (0.14) | −0.049 | −0.092 |
I’m very fine | 0.46 (0.15) | −0.189* | −0.173 |
Yesterday I had a good sleep | 0.47 (0.18) | −0.107 | −0.218* |
I have an appetite | 0.52 (0.19) | −0.200* | −0.184* |
I feel calm | 0.48 (0.15) | 0.082 | 0.042 |
I feel irritable | 0.49 (0.17) | 0.023 | 0.011 |
I feel tired and exhausted | 0.56 (0.17) | 0.116 | 0.168 |
I always looked ahead | 0.40 (0.15) | −0.078 | −0.129 |
Come on, myself! | 0.39 (0.14) | −0.108 | −0.149 |
/Ah/ sound | 0.41 (0.15) | −0.179* | −0.193* |
/Eh/ sound | 0.38 (0.13) | −0.061 | −0.111 |
/Uh/ sound | 0.38 (0.13) | −0.138 | −0.208* |
*: p-value < 0.05
Vitality scores from 65,519 phrase combinations were also examined for the study objectives. Among them, the Vitality score from a combination of six phrases showed the highest overall results and was chosen for the report.
The correlation between the Vitality and test scores, adjusted for age and sex, is shown in Figure 2. The adjusted correlation between the Vitality and BDI scores was negative with a value of −0.272 and was significant (p-value = 0.003) (Figure 3). Similar result of correlation was found between the Vitality and PHQ-9 score (r = −0.215, p-value = 0.018) (Figure 4).
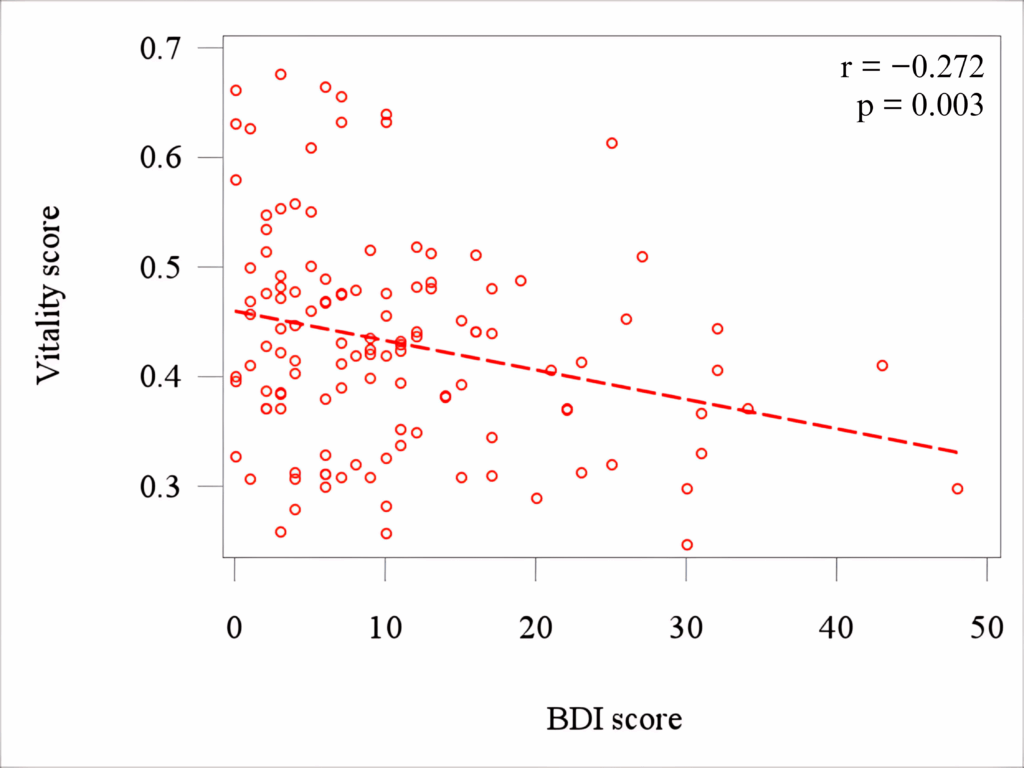
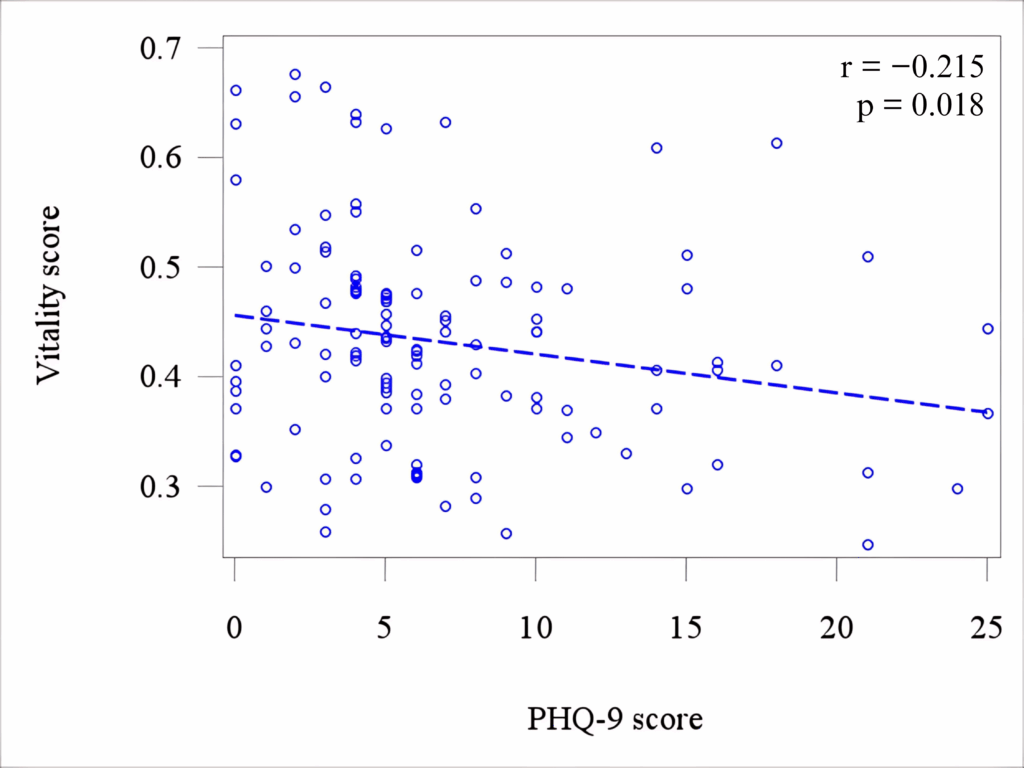
The box and whisker plots of the Vitality scores among those with a low and a high risk of depression are shown in Figure 5. When comparing the participants categorized according to their BDI score, the high-risk group showed a significantly lower Vitality score than the low-risk group (p-value at 0.024). However, there was no significant difference in the Vitality score between the groups categorized according to the PHQ-9 score (Figure 6).
3.2. MIMOSYS performance in discriminating individuals with a high risk of depression
Using the BDI as a standard, the AUC of the Vitality score controlled for age and sex was 0.717, and the ROC model is significant (p-value = 0.001) (Figure 7). Using the PHQ-9 as standard, the AUC was 0.623; however, the ROC model is not significant (p-value > 0.05) (Figure 8). The sensitivity, specificity, and accuracy of the “optimal cutoff point” in discriminating individuals with a low and a high risk of depression were reported in Table 4. A comparison regarding the effectiveness of the Vitality score in identifying depressive disorder between this study and previous studies was additionally shown in Table 5.
Table 4: Discriminating performance of the Vitality score (n=123)
Vitality cutoff = 0.4139 |
PHQ-9 score cutoff = 12 |
Sen. | Spec. | Acc. | |
High (n) | Low (n) | ||||
High risk | 13 | 42 | 0.68 | 0.60 | 0.61 |
Low risk | 6 | 62 | |||
Vitality cutoff = 0.4139 |
BDI score cutoff = 19 |
Sen. | Spec. | Acc. | |
High (n) | Low (n) | ||||
High risk | 15 | 40 | 0.75 | 0.61 | 0.63 |
Low risk | 5 | 63 |
Sen.: Sensitivity; Spec.: Specificity; Acc.: Accuracy
Table 5: Performance of the Vitality score in different studies using BDI (n=123)
Author | Hagiwara [8] | Higuchi [16] | Shinohara [17] | This study |
Year | 2017 | 2017 | 2017 | 2023 |
Language | Jap. | Jap. | Jap. | Vie. |
AUC | 0.78 | 0.64 | 0.80 | 0.72 |
Sensitivity | 0.80 | NR | 0.94 | 0.75 |
Specificity | 0.64 | NR | 0.64 | 0.61 |
Accuracy | 0.66 | NR | NR | 0.63 |
Jap.: Japanese; Vie.: Vietnamese; NR: not reported
4. Discussion
Our study evaluated the effectiveness of the Vitality, a biomarker retrieved from voice, to assess the depression status of Vietnamese individuals. A lower Vitality score denotes a more severe state of depression [17], while the PHQ-9 and the BDI questionnaires interpret a lower score as milder depressive status. In other words, the Vitality score and these psychological test scores are expected to have a negative correlation.
In this study, voice data were divided into utterances; each utterance corresponded to a voice uttered for a designated phrase. It is worth noting that not all of the recorded phrases showed a negative correlation with psychological tests; a similar issue was also reported in the research conducted in the Japanese language [10]. Among all combinations of phrases generated and examined from our study, the Vitality score from the combination of six utterances was chosen for analyzing the study objectives. The combination consists of Phrase 1 (A-B-C-D-E-F-G), Phrase 6 (I am very fine), Phrase 7 (Yesterday I had a good sleep), Phrase 8 (I have an appetite), Phrase 12 (I always look ahead), and Phrase 13 (Come on, myself!). The vitality score extracted from each of these phrases was negatively correlated with both the PHQ-9 score and the BDI score (as shown in Table 3). Our proposed combination also met the minimum number of utterances recommended for the MIMOSYS to achieve sufficient accuracy of the Vitality score [9].
4.1. Correlation between the Vitality score and self-administered psychological test scores
In our study, a weak negative correlation was found between the Vitality and the PHQ-9 score as well as the BDI score. A similar trend of correlation was also reported in a previous study using the BDI test and voice data recorded via phone, with a smaller magnitude of 0.208 [8]. The difference in results can possibly be due to the difference in the way the voice data was collected. In our study, the participant’s voice was recorded by a specialized recorder, in a noise-controlled environment. Since the interference of noise from the surrounding environment may decrease the precision of utterance detection, it can lead to an unproperly analysis of the Vitality score [18] Another factor that may affect the result is whether the voice data is reading speech or spontaneous speech. As the Vitality score is calculated based on changes in emotion, it is likely that reading a fixed sentence would generate less variance in emotional expression than, for example, a phone conversation, which resulted in a lower value of the Vitality score [8].
Regarding the difference in the Vitality score between groups categorized by the test’s cutoff, a significant result was found only when using the BDI test. Previous studies using the same questionnaire in other languages such as Japanese, Romanian, or Russian also reported similar results [11], [17]. Result using the PHQ-9 test, however, showed no difference in Vitality score between the low-risk group and high-risk group. Since the PHQ-9 includes 9 items with a relatively narrow sum score, it does not necessarily reflect an individual’s personal depression experiences in their life [19], [20]. The questionnaire is also commonly used to measure outcomes in primary care rather than being used as a diagnostic tool [21].
4.2. Performance in discriminating individuals with high risk of depression
Using the BDI test as a standard, a moderate accuracy AUC of Vitality score was found in our study. Our result was higher than a similar study conducted on Japanese seniors [22], but lower than the study involving Japanese employees [8]. The result of the AUC may be interfered by other un-examined factors such as the homogeneity of the study population, number of participants, or the voice data collecting method.
Results on the sensitivity, specificity, and accuracy from our study were relatively close to, but lower than the report numbers from the previous studies conducted in Japanese [8], [17]. Enhancing the performance of Vitality, especially the specificity result, remains a future research issue for the applicability of this voice analysis technique in Vietnamese. This result also suggests the potential effect of acoustic differences between the Japanese and Vietnamese. Further studies focused on addressing this difference may help clarify the mechanism of pathophysiological voice analysis in different languages.
4.3. Limitations
Due to the small sample size and convenient sampling method, the generalizability of our findings was limited. Compared to the Vitality score, the MIMOSYS’s Mental Activity score, which was not generated and evaluated in our study, was reported to have a higher performance both in the correlation and discrimination evaluations with the BDI test [8]. Besides the emotion-based technique, voice data can be analyzed by its acoustical characteristics such as shimmer, jitter, or harmonic-to-noise Ratio. However, this technique was not included and parallel examined in our study; thus, the performance of MIMOSYS may not have been comprehensively compared yet. Spontaneous or free speech is reported to better classify individuals with a major depressive disorder than reading designated sentences [7]. Therefore, further comparison of the results from different types of voice data in the Vietnamese language is necessary.
5. Conclusion
Although our findings may support the use of the voice analysis technique in the Vietnamese language by its acceptable results, further investigations are necessary to confirm and enhance the performance of depression assessment of this method.
Conflict of Interest
The authors declare no conflict of interest.
Acknowledgment
We would like to express our sincere appreciation to the Faculty of Public Health, University of Medicine and Pharmacy at Ho Chi Minh City, and to the Department of Clinical Psychology, Le Van Thinh Hospital, Ho Chi Minh City for their substantial support in conducting the research. We also acknowledge Enago (www.enago.com) for English language review.
- T. Alloh, P. Regmi, I. Onche, E.v. Teijlingen, S. Trenoweth, “Mental Health in low-and middle income countries (LMICs): Going beyond the need for funding,” Health Prospect, 17(Special Issue), 12–17, 2018, doi:10.3126/hprospect.v17i1.20351.
- World Health Organization, World Mental Health Report; Transforming Mental Health for all. WHO: Geneva, 2022.
- A. Vuong, E.v. Ginneken, J. Morris, S.T. Ha, R. Busse, “Mental health in Vietnam: burden of disease and availability of services,” Asian J Psychiatr, 4(1), 65–70, 2011, doi:10.1016/j.ajp.2011.01.005.
- Shinohara, H. Toda, M. Nakamura, Y. Omiya, M. Higuchi, T. Takano, T. Saito, M. Tanichi, S. Boku, S. Mitsuyoshi, et al., “Evaluation of emotional arousal level and depression severity using voice-derived sound pressure change acceleration,” Scientific Reports, 11(1), 13615, 2021, doi:10.1101/2020.08.19.20177048.
- K. Patel, L. Nivethitha, A. Mooventhan, “Effect of a yoga based meditation technique on emotional regulation, self-compassion and mindfulness in college students,” Explore, 14(6), 443–447, 2018, doi:10.1016/j.explore.2018.06.008.
- Omiya, T. Takano, T. Uraguchi, M. Nakamura, M. Higuchi, S. Shinohara, S. Mitsuyoshi, M. So, S. Tokuno, An attempt to estimate depressive status from voice – in book: Pervasive Computing Paradigms for Mental Health, Springer, 2019.
- Shinohara, Y. Omiya, M. Nakamura, N. Hagiwara, M. Higuchi, S. Mitsuyoshi, S. Tokuno, “Multilingual evaluation of voice disability index using pitch rate,” Advances in Science, Technology and Engineering Systems Journal, 2(3), 765–772. 2017, doi:10.25046/aj020397.
- Hagiwara, Y. Omiya, S. Shinohara, M. Nakamura, M. Higuchi, S. Mitsuyoshi, H. Yasunaga, S. Tokuno, “Validity of Mind Monitoring System as a mental health indicator using voice,” Advances in Science Technology and Engineering Systems, 2(3), 338–344, 2017, doi:10.25046/aj020343.
- Higuchi, M. Nakamura, S. Shinohara, Y. Omiya, T. Takano, S. Mitsuyoshi, S. Tokuno, “Effectiveness of a voice-based mental health evaluation system for mobile devices: prospective study,” JMIR Formative Research, 4(7), e16455, 2020, doi:10.2196/16455.
- Higuchi, N. Sonota, M. Nakamura, K. Miyazaki, S. Shinohara, Y. Omiya, T. Takano, S. Mitsuyoshi, S. Tokuno, “Performance evaluation of a voice-based depression assessment system considering the number and type of input utterances,” Sensors, 22(67), 1–12, 2022, doi:10.3390/s22010067.
- Uraguchi, S. Shinohara, M. Taicu, G. Savoiu, Y. Omiya, M. Nakamura, M. Higuchi, T. Takano, N. Hagiwara, S. Mitsuyoshi, et al., “Evaluation of Mind Monitoring System (MIMOSYS) by subjects with Romanian and Russian as their native language,” in 2018 IEEE International Conference in Medicine and Biology Society (EMBC2018).
- Mitsuyoshi, F. Ren, Y. Tanaka, S Kuroiwa, “Non-verbal voice emotion analysis system,” International journal of innovative computing, information & control, 2(4), 819–830, 2006.
- Shinohara, M. Nakamura, Y. Omiya, M. Higuchi, N. Hagiwara, S. Mitsuyoshi, H. Toda, T. Saito, M. Tanichi, A. Yoshino, et al., “Mental health assessment method based on emotion level derived from voice,” Preprints, 2020, doi:10.21203/rs.2.18354/v1.
- H.B. An, N.T.T. Ngoc, V.Q. Nghia, H.T.N. Ngoc, B.C. Minh, L.V. My, C.T. Nha, N.H. Dung, “Application of the Beck Depression Inventory – II, Zung Self-Rating Anxiety Scale and Pittsburgh Sleep Quality Index on student of School of Medicine – Vietnam National University Ho Chi Minh City: a pilot study,” (article in Vietnamese with an abstract in English), Journal of Science and Technology Development – Health Sciences, 2(2), 323–329, 2021, doi:10.32508/stdjhs.v2i2.490.
- T. Nguyen, P.L. An, N.D.P. Tien, “Reliability and validity of Vietnamese version of patient health questionnaire 9 items (PHQ-9) among UMP medical freshmen,” in 8th International Conference on the Development of Biomedical Engineering in Vietnam, 901–923, 2019, doi:10.1007/978-3-030-75506-5_72.
- Higuchi, S. Shinohara, M. Nakamura, Y. Omiya, N. Hagiwara, S. Mitsuyoshi, S. Tokuno, “Study on depression evaluation indicator in the elderly using sensibility technology,” in 3rd International Conference on Information and Communication Technologies for Ageing Well and e-Health, 70–77, 2017, doi:10.5220/0006316700700077.
- Shinohara, Y. Omiya, N. Hagiwara, M. Nakamura, M. Higuchi, T. Kirita, T. Takano, S. Mitsuyoshi, S. Tokuno, “Case studies of utilization of the Mind Monitoring System (MIMOSYS) using voice and its future prospects,” Econophysics, Sociophysics & other Multidisciplinary Sciences Journal, 7, 7–12, 2017.
- Higuchi, S. Shinohara, M. Nakamura, Y. Omiya, N. Hagiwara, T. Takano, S. Mitsuyoshi, S. Tokuno, “Accuracy evaluation for mental health indicator based on vocal analysis in noisy environments,” Journal of Information and Communication Engineering, 4(1), 217–222 2018, doi:10.5281/zenodo.4300086.
- Dowrick, G.M. Leydon, A. McBride, A. Howe, H. Burgess, P. Clarke, S. Maisey, T. Kendrick, “Patients’ and doctors’ views on depression severity questionnaires incentivised in UK quality and outcomes framework: Qualitative study,” British Medical Journal, 338(b663), 2009, doi:10.1136/bmj.b663.
- Malpass, A. Shaw, D. Kessler, D. Sharp, “Concordance between PHQ-9 scores and patients’ experiences of depression: a mixed methods study,” British Journal of General Practice, 60(575), e231–e238, 2010, doi:10.3399/bjgp10X502119.
- Ford, F. Thomas, R. Byng, R. McCabe, “Use of the Patient Health Questionnaire (PHQ-9) in practice: interactions between patients and physicians,” Qualitative Health Research, 30(13), 2146–2159, 2020, doi:10.1177/1049732320924625.
- Y. Omiya, N. Hagiwara, M. Nakamura, S. Shinohara, M. Higuchi, T. Takano, “音声を用いたメンタルヘルス状態計測における年齢及び性別の影響の検証,” Journal of Industrial Hygiene, 60(Special Issue), 487, 2018.
No. of Downloads Per Month