Revolutionizing Robo-Advisors: Unveiling Global Financial Markets, AI-Driven Innovations, and Technological Landscapes for Enhanced Investment Decisions
Volume 9, Issue 2, Page No 33-44, 2024
Author’s Name: Marco I. Bonelli1, a), Jiahao Liu2
View Affiliations
1 Dr. Vishwanath Karad MIT World Peace University, Pune, 411038, India
2 Judge Business School, University of Cambridge, Cambridge, CB2 1AG, United Kingdom
a)whom correspondence should be addressed. E-mail: Mibonelli6@gmail.com
Adv. Sci. Technol. Eng. Syst. J. 9(2), 34-44 (2024); DOI: 10.25046/aj090205
Keywords: Robo-advisors, Full-scale optimization, Digital twin, Natural language processing, Artificial intelligence
Export Citations
Robo-advisors, fundamental to the financial services sector, have undergone substantial technological metamorphosis. Innovations in artificial intelligence, blockchain, cloud technology, augmented reality, and virtual reality have reshaped the financial industry’s landscape. As automated investment solutions, robo-advisors are on the brink of further technological evolution. This comprehensive research amalgamates historical data, behavioral insights, and emerging market trends to provide technology-centric recommendations for the robo-advisory industry. Emphasizing the significance of a global perspective, the study explores the adoption of full-scale optimization in portfolio construction and the integration of digital twin capabilities. It delves into the burgeoning realm of Natural Language Processing facilitated by AI-driven chatbots in financial technology companies. These recommendations stand as pivotal pillars for steering the ongoing technological advancements of robo-advisors in the ever-evolving landscape of the financial sector.
Received: 05 November 2023, Revised: 05 March 2024, Accepted: 16 March 2024, Published Online: 20 April 2024
1. Introduction
This paper extends work originally presented in 2023 at the 9th International Conference on Virtual Reality (ICVR 2023), held on May 14th, 2023, in Xianyang, China. Investors today leverage robo-advisors as powerful tools for efficient portfolio management. This is particularly beneficial for small investors navigating the complexities of crafting tailored investment strategies. Operating on sophisticated algorithms, robo-advisors autonomously oversee investment portfolios, increasing the likelihood of attaining financial goals.
Beyond portfolio management, these digital platforms extend their support to investors by providing a spectrum of financial planning services, ranging from budgeting and debt management to comprehensive retirement planning. This holistic approach empowers businesses to cultivate sustainable financial models, fortifying their overall financial health.
The emergence of robo-advisors signifies a transformative shift in investment advisory, offering a cost-effective alternative to traditional human advisors. Originating as fintech startups post the global financial crisis, robo-advisors have surged in popularity, with established financial institutions like Charles Schwab and BlackRock integrating robo-advisory services into their portfolios. Major players, including Bank of America and Wells Fargo, have also introduced their automated advisory services.
The United States dominates the robo-advisory market, hosting over 200 robo-advisors and commanding 57% of global robo-advisory investments as of 2017. With assets exceeding $400 billion in 2018 and an impressive annual growth rate of approximately 30%, projections indicate that by 2023, assets overseen by robo-advisors in the US will approach $1.5 trillion. Vanguard, Schwab Intelligent Portfolios, and Betterment stand out as major players by assets under management [1]. While the US leads, robo-advisory services are gaining traction globally. Europe hosts over 70 robo-advisors, with five managing assets exceeding €100 million. In Asia, particularly in developing markets, the proliferation of robo-advisors is propelled by a growing middle class and robust technological connectivity.
This study navigates through the industry’s history, emerging trends, strengths, and weaknesses, pinpointing potential areas for growth. It posits three key predictions: a shift from Modern Portfolio Theory towards Full-Scale Optimization, the integration of Digital Twin capabilities to harness user data, and the development of Natural Language Processing to elevate the user experience. These insights encapsulate the evolving landscape of robo-advisory services, positioning them for sustained global growth and innovation.
2. Literature Review
In recent years, the landscape of the financial services sector has been marked by a profound and dynamic transformation propelled by the integration of cutting-edge technologies, notably artificial intelligence (AI) [2-5]. This technological wave has seen the rise of robo-advisors as a pivotal force, fundamentally reshaping investment management and financial planning services, as highlighted by a study by Deloitte [6].
Nevertheless, while the preceding introduction offers a glimpse into this transformative landscape, it only scratches the surface of a multidimensional tableau. This study sets sail to delve into the complexities of robo-advisors on a global scale, focusing on their evolutionary journey and technological advancements. In this exploration, it aims to shed light on the disparities observed in the worldwide automated investment advisory sphere while acknowledging, for example an emerging market like India’s with significantly higher growth, anticipated to reach a notable 8.5% annually. [1].
Guided by a central research question resonating across scholarly discourse—”Amid the AI surge, how can robo-advisors ascend to new frontiers, and what fault lines underlie the landscape of robo-advisory services globally?”[4], [7-11]—this intellectual exploration aspires to unearth insights that shed light on potential advancements and bridge the gaps awaiting in the realm of AI-augmented financial guidance and strategy.
2.1. Global Landscape of Robo-Advisors and AI Technology
Robo-advisors have garnered swift global recognition, primarily for their capacity to provide automated and personalized investment solutions, a facet reaffirmed in longitudinal studies [12]. These platforms, driven by intricate algorithms, consider individual risk profiles and financial objectives when creating and managing portfolios [13], [14] even extending to crypto asset allocation [15]. AI, a linchpin of robo-advisory technology, empowers comprehensive data analysis, pattern recognition, and predictive modeling [1]. AI-fueled robo-advisors, both from established institutions and startups, possess the potential to reshape the financial sector, making investment opportunities more accessible and cost-efficient [4], [6], [8], [15], [16]. Notably, robo-advisors provide the capability to incorporate global environmental constraints into stock forecasts [4]. A prevalent challenge in the adoption of advanced AI-powered robo-advisor technology is the lack of trust, a pivotal topic in customer studies globally and within regional markets [3], [8], [10], [11].
2.2. Advancing Technology Through AI
The AI explosion has ushered in new horizons for enhancing robo-advisory capabilities [4], [8]. The incorporation of AI into robo-advisors has unlocked the potential for more precise predictions, streamlined risk assessment, and optimized portfolio management [17]. Techniques such as machine learning, deep learning, and natural language processing have contributed to the refinement of investment strategies and the enhancement of the customer experience [11]. By harnessing the capabilities of AI, robo-advisors stand poised to offer more resilient and personalized solutions, ultimately elevating investor satisfaction and engagement [10].
3. Applications, Advantages, and Insights from Behavioral Studies
3.1. Efficient Functioning of Robo-Advisors
Robo-advisors adopt a personalized approach to shaping investment decisions, initiating the creation of individualized investment plans tailored to specific goals and risk preferences. Prospective clients undergo inquiries regarding their investment purpose, horizon, and readiness to endure market fluctuations, enabling robo-advisors to propose plans for various objectives, such as significant expenditures, retirement, income generation, or emergency fund building [18].
To ensure cost-effectiveness, robo-advisors employ online questionnaires to assess clients’ needs, investment goals, and risk profiles. Automated algorithms utilize this information to recommend asset allocations across different types of assets, with portfolio optimization typically grounded in Modern Portfolio Theory [19]. The entire process, often completed online without human interaction, ensures efficient portfolio management.
3.2. Cost Optimization and Returns
Retail investors utilizing robo-advisors benefit from significantly lower management fees, typically ranging from 20% to 30% of fees charged by human financial advisors due to automation and scalability [20, 21]. Robo-advisors aim to reduce costs, discouraging fee-inducing practices like excessive trading. Studies indicate a substantial reduction in average retail investor expense ratios, leading to significant savings and reinvestment opportunities [22].
Utilizing passively managed ETFs, robo-advisors offer less expensive and transparent fee structures, empowering clients to determine costs easily. Research indicates that robo-advisors in the US have outperformed popular mutual funds and major equity indices in value-weighted returns between 2016 and 2019, emphasizing their cost-effectiveness and potential for better risk-adjusted returns [24].
3.3. Behavioral Impact on Retail Investors
Robo-advisors play a crucial role in mitigating behavioral biases, such as under-diversification, overtrading, and herd mentality, prevalent among retail investors [25-27]. These platforms, driven by algorithms, craft portfolios tailored to individual objectives and risk tolerance, effectively addressing concerns related to behavioral biases [28].
Research highlights that robo-advisors effectively counter under-diversification, reducing portfolio volatility and enhancing performance. The services act as a shield against behavioral biases associated with DIY investment approaches, providing a more disciplined investment strategy. While not entirely immune to biases, robo-advisors contribute to countering behavioral pitfalls such as the disposition effect, rank effect, and trend-chasing, ensuring more rational investment decisions [29].”
3.4. Current State of the Industry
Robo-advisory services are poised for a robust Compound Annual Growth Rate (CAGR) of 40% from 2021 to 2026, emphasizing the steady expansion of this sector. The industry is witnessing a shift toward advanced portfolio strategies, integrating artificial intelligence (AI) and machine learning to align with the ongoing wave of financial digitization [30, 31]. Hybrid robo-advisors, combining human and automated advice, dominate the sector, reflecting the enduring demand for human involvement in portfolio management [32].
The Covid-19 pandemic underscored the resilience of robo-advisors, with certain platforms doubling their client base during lockdowns. Platforms equipped with advanced technology showcased their ability to withstand market shocks, attracting a larger clientele [30]. Notable performers during volatile market conditions included Wealth Front, Morgan Stanley Inflation Conscious, and Personal Capital, emphasizing the appeal of robo-advisory services in navigating challenging economic landscapes.
4. Research Study
The Web-Based Research Design study revolves around gathering data from the Internet concerning global financial indicators. Information regarding financial markets was systematically collected using platforms like statista.com. Additionally, the study involved employing Google Trends to gauge the level of interest in financial technology, as outlined by Vasylchenko and Bonelli in 2023 [33]-[36]. The data collection process also encompassed obtaining comprehensive insights into the most popular global Robo-Advisors and AI-driven solutions.
4.1. Analysis Key Aspects:
During our analysis, we focused on evaluating the following key aspects:
- Obtaining a comprehensive global perspective of the financial markets, both on a global scale.
- Investigating the status of technology within the global landscape of Robo-Advisors.
- Exploring opportunities for enhancing technology through the implementation of AI-driven solutions.
- Exploring the status of technology within the global landscape of Robo-Advisors.
Statista [7], the publisher of financial data, allowed us to collect data about the global perspective of the financial market within the context of India. That includes key points of financial market development, actual financial data about market volume worldwide, and forecasts for India until 2030.
In the first stage, information about the most frequently mentioned robo-advisors online was filtered [37-45]. About 70 international Robo-Advisors (Advisors who work worldwide, in many countries) lists were formed. For each Robo-Advisor, data about user count, capitalization of assets managed by, and interest data about search requests about Advisors were collected.
The Betterment Robo-Advisor, serving 775,000 customers with assets totaling $36 billion, was chosen as the benchmark for comparing relative measures with other advisors. Operating globally, with its headquarters in the US, this data was utilized to compute the relative interest in other advisors worldwide, incorporating the average Google Trend values for Robo-Advisors [36]. The tools advisors use to help investors through Finance Advisors, Robo Advisors, and AI trading were classified into the next categories.
Table 1: Advisor tools
Tool Category | Definition |
Risk Assessment and Profiling | Robo-advisors collect information about an investor’s risk tolerance, investment goals, and financial situation to create personalized investment profiles. |
Portfolio Creation | Based on the investor’s profile, robo-advisors automatically build diversified portfolios using various asset classes like stocks, bonds, and alternative investments. |
Asset Allocation | Robo-advisors use algorithms to determine the optimal distribution of assets in the portfolio based on the investor’s risk profile and market conditions. |
Rebalancing | Robo-advisors monitor the portfolio and periodically adjust the allocation to maintain the desired balance as market conditions change. |
Tax Efficiency | Some robo-advisors optimize investment decisions for tax efficiency, including strategies like tax-loss harvesting to minimize capital gains taxes. |
Goal-Based Investing | Robo-advisors help investors set specific financial goals, such as retirement or buying a home, and create investment strategies tailored to those goals. |
Automated Trading | Many robo-advisors automatically execute trades based on pre-defined investment strategies and market conditions. |
Cost Optimization | Robo-advisors often build portfolios using low-cost exchange-traded funds (ETFs) or index funds, aiming to minimize investment expenses. |
Diversification | Robo-advisors ensure that portfolios are well-diversified across various assets to reduce risk and enhance returns. |
Continuous Monitoring | They continuously monitor the portfolio’s performance and provide updates and notifications to investors. |
Education and Insights | Robo-advisors offer educational resources and insights to help investors understand their investments and make informed decisions. |
User-Friendly Interfaces | Many robo-advisors provide easy-to-use online platforms and mobile apps for investors to track their portfolios, make changes, and access information. |
Savings and Investing Automation | Some robo-advisors allow users to automate regular contributions to their investment accounts, helping investors save and invest consistently over time. |
Accessibility | Robo-advisors make investing accessible by offering lower account minimums compared to traditional financial advisors. |
Personalized Recommendations | They provide recommendations for additional investments or adjustments based on changing market conditions and the investor’s goals. |
Behavioral Finance Strategies | Certain robo-advisors incorporate behavioral finance principles to help investors stay disciplined and avoid emotional decisions. |
Financial Planning | Some robo-advisors offer comprehensive financial planning services, including retirement planning, estate planning, and goal tracking. |
Transparency | Many robo-advisors provide transparent fee structures, allowing investors to see exactly how much they are paying for the service. |
Customer Support | Although automated, robo-advisors often offer customer support through various channels to assist with account-related queries. |
4.2. Exploring opportunities for enhancing technology through the implementation of AI-driven solutions.
Advisor tools and AI Enhancement options were collected in the next table [37-50]. The next conjoint of these papers was summarized in Table 2 [36].
Table 2: AI Enhancement
AI Enhancement | Description |
Advanced-Data Analysis |
AI analyzes extensive financial data from various sources to identify patterns and insights for informed investment strategies. |
Predictive Modeling |
AI creates predictive models using historical data to estimate future market movements, enabling more accurate investment decisions. |
Natural Language Processing (NLP) |
NLP technology helps robo-advisors understand text-based information, such as news and social media, to assess market sentiment. |
Personalized Recommendations |
AI analyzes individual investor profiles, preferences, and risk tolerance to provide highly tailored investment recommendations. |
Dynamic Asset Allocation |
AI algorithms adjust asset allocations in real time based on changing market conditions to keep portfolios aligned with goals. |
Behavioral Finance Analysis |
AI studies investor behavior patterns and biases, offering strategies to avoid behavioral pitfalls and make rational decisions. |
Automated Tax Optimization |
AI optimizes tax-related decisions, like tax-loss harvesting, to minimize tax liabilities and enhance after-tax returns. |
Risk Assessment and Management |
AI performs advanced risk analysis beyond traditional metrics to provide accurate risk assessments and effective risk management. |
Alternative Data Utilization |
AI processes unconventional data sources to gain insights into potential investment opportunities, enhancing decision-making. |
Portfolio Stress Testing |
AI simulates the impact of market scenarios on portfolios, helping investors understand potential performance under different conditions. |
Market Timing |
AI identifies potential entry and exit points by analyzing technical indicators and historical trends to enhance investment timing. |
Real-time Alerts |
AI generates real-time alerts for significant market events or changes, keeping investors informed of potential adjustments. |
Natural Language Interaction | AI-enabled interfaces offer conversational interactions, allowing users to engage with the platform using natural language. |
Fraud Detection | AI identifies unusual patterns in account activity, detecting potentially fraudulent activities and enhancing security. |
Continuous Learning | AI systems learn from interactions and continuously improve recommendations and strategies based on feedback and outcomes. |
Market Sentiment Analysis | AI analyzes social media and news to gauge market sentiment, incorporating sentiment analysis into investment decisions. |
Deep Learning for Complex Analysis |
AI techniques like deep learning analyze intricate financial models and predict complex outcomes for more accurate decisions. |
Cognitive Computing |
AI processes unstructured data, making sense of diverse information sources and enhancing decision-making capabilities. |
Enhanced User Experience |
AI-driven interfaces offer personalized dashboards, interactive visualizations, and user-centric features, enhancing the investor experience. |
Google Trends used search engine data to determine interest in finding interesting information during the last year. Developer reports were used to determine the actual number of users and assets under management. Sometimes, if user data was not accessible, Google Play market data were used to determine the current count of users for applications.
These are the worldwide robo-advisor list used for this study: Capital One Investing, Schwab Intelligent Portfolios, Etoro, Marcus Invest, TD Ameritrade Essential Portfolios, XM Group, Ally Invest, Fidelity Go, SoFi Invest, E*TRADE Core Portfolios, Acorns, Charles Schwab Intelligent Portfolios, DBS, FxPro, Morgan Stanley Access Investing, Betterment, Interactive Brokers, Wealthfront, M1 Finance, Fundrise, Plus500, Nutmeg, Ellevest, Syfe, StashAway, OCBCBank, Merrill Guided Investing, Kristal.AI, Titan Invest, SigFig, CitiWealthBuilder, Modern Advisor, BMO Smartfolio, MSCI, FutureAdvisor, FP Markets, Qtrade Guided Portfolios, Empower/Personal Capital, Endowus, CI Direct, and Axos Invest [36].
4.3. Research Methodology and Evaluation
In our pursuit of understanding the global robo-advisor landscape, we used Google Trends and developers’ reports to assess user interest and metrics. The study, anchored in a worldwide robo-advisor list featuring major players like Capital One Investing and Schwab Intelligent Portfolios, formed the basis of our approach. Our analysis covered 94.7% of the global robo-advisor market, excluding smaller Indian players. We curated robo-advisor lists, explored AI roles, and utilized Google Trends for global and Indian insights on popularity and enhancements [36].
4.4. Interest Measures
To complete our quantitative analysis, we took advantage of the power of Google Trends, recognizing its dominance as the predominant search engine globally, capturing approximately 96-98% of search requests. This expansive coverage allowed us to gain insights into the worldwide interest trends related to robo-advisors, offering a perspective on the evolving landscape of financial technology.
Google Trends used search engine data to determine interest in finding interesting information during the last year. Developer reports were used to determine the actual number of users and assets under management. Sometimes, if user data was not accessible, Google Play market data were used to determine the current count of users for applications.
These are the worldwide robo-advisor list used for this study: Capital One Investing, Schwab Intelligent Portfolios, Etoro, Marcus Invest, TD Ameritrade Essential Portfolios, XM Group, Ally Invest, Fidelity Go, SoFi Invest, E*TRADE Core Portfolios, Acorns, Charles Schwab Intelligent Portfolios, DBS, FxPro, Morgan Stanley Access Investing, Betterment, Interactive Brokers, Wealthfront, M1 Finance, Fundrise, Plus500, Nutmeg, Ellevest, Syfe, StashAway, OCBCBank, Merrill Guided Investing, Kristal.AI, Titan Invest, SigFig, CitiWealthBuilder, Modern Advisor, BMO Smartfolio, MSCI, FutureAdvisor, FP Markets, Qtrade Guided Portfolios, Empower/Personal Capital, Endowus, CI Direct, and Axos Invest [36].
4.3. Research Methodology and Evaluation
In our pursuit of understanding the global robo-advisor landscape, we used Google Trends and developers’ reports to assess user interest and metrics. The study, anchored in a worldwide robo-advisor list featuring major players like Capital One Investing and Schwab Intelligent Portfolios, formed the basis of our approach. Our analysis covered 94.7% of the global robo-advisor market, excluding smaller Indian players. We curated robo-advisor lists, explored AI roles, and utilized Google Trends for global and Indian insights on popularity and enhancements [36].
4.4. Interest Measures
To complete our quantitative analysis, we took advantage of the power of Google Trends, recognizing its dominance as the predominant search engine globally, capturing approximately 96-98% of search requests. This expansive coverage allowed us to gain insights into the worldwide interest trends related to robo-advisors, offering a perspective on the evolving landscape of financial technology.
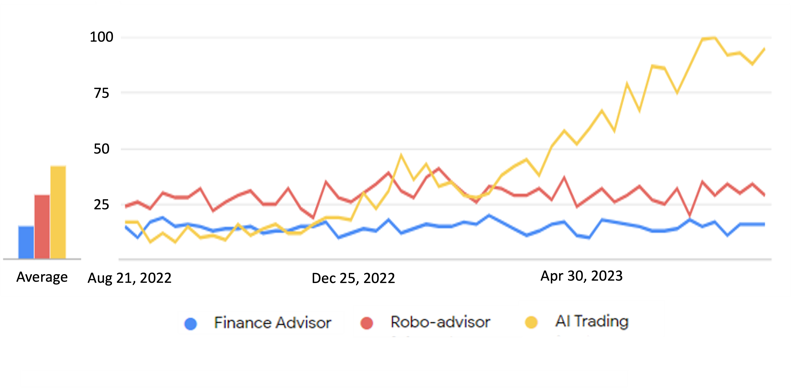
Figure 1, titled “Interest Over Time,” illustrates the frequency of searches for key terms such as “Finance Advisor,” “Robo Advisor,” and “AI Trading” over the past year.
The data derived from Google Trends serves as a valuable tool for monitoring and comparing the popularity of specific search queries, enabling a dynamic understanding of user preferences and interests in the financial technology domain [36].
5. Findings
5.1. Global perspective of the financial market
In Figure 2, we present a comprehensive overview of the global financial services sector, revealing a projected market value of around $31 billion based on insights from a report by BLinC Invest [51]. This figure encapsulates the expansive scope and economic significance of the financial services industry on a global scale. The forecasted trajectory of the market points towards a notable growth trend, with an anticipated annual growth rate of 6.8%. Notable is the growth in emerging markets like India, which is over double the rate compared to the average overall growth rate [51]. This projection underscores the sector’s resilience and its capacity to evolve, signaling opportunities for expansion and innovation within the financial services domain. Analyzing these projected values provides crucial insights into the dynamic nature of the global financial landscape, setting the stage for strategic considerations and potential advancements in the industry.
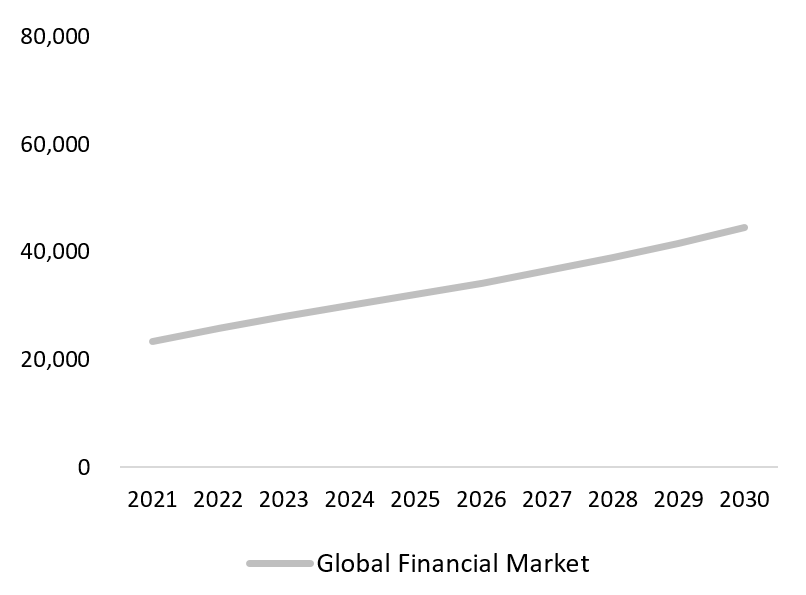
5.2. Investigating the status of technology within the global landscape of Robo-Advisors
Our investigation reveals a global landscape of robo-advisors, where the most influential players collectively command 94.7% of the world’s assets. To discern their prominence, we utilized assets under management as the benchmark for ranking these advisors. This criterion was selected to accommodate the diverse nature of advisors catering to a myriad of customer segments.
Figure 3, as depicted in our research [36], visually underscores the dominance of these major global advisors and serves as a key reference point for understanding their significance in the industry.
The diverse clientele of these Robo-Advisors spans a wide spectrum of needs and preferences, encompassing various categories such as Individual Investors, Quick Traders, International Funds, Banks and Institutions, Retirement Planners, Novice Investors, Ethical and Socially Responsible Investors, High-Net-Worth Individuals, Young Investors, Passive Investors, Digital Natives, Small Businesses, Educational Savings, Real Estate Investors, Customized Portfolios, Tax-Efficient Investors, Conservative Investors, Alternative Investments.
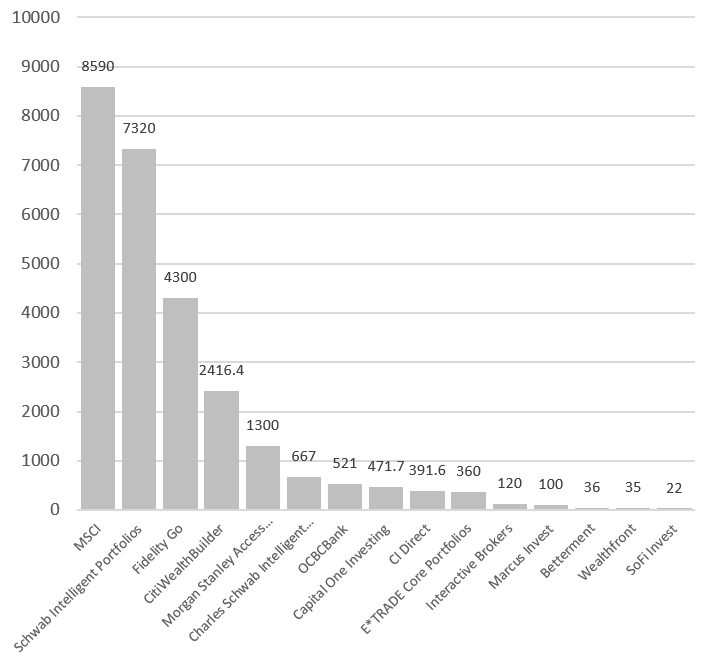
5.3. Exploring Opportunities for Enhancing Technology through AI-Driven Solutions
In our quest to advance technology within the financial sector, we conducted a thorough examination of AI-driven solutions, shedding light on significant trends and user preferences. Figure 4 visually represents the evolving landscape of AI trading and its intersection with user interests, serving as a compelling testament to the comprehensive analysis [36].
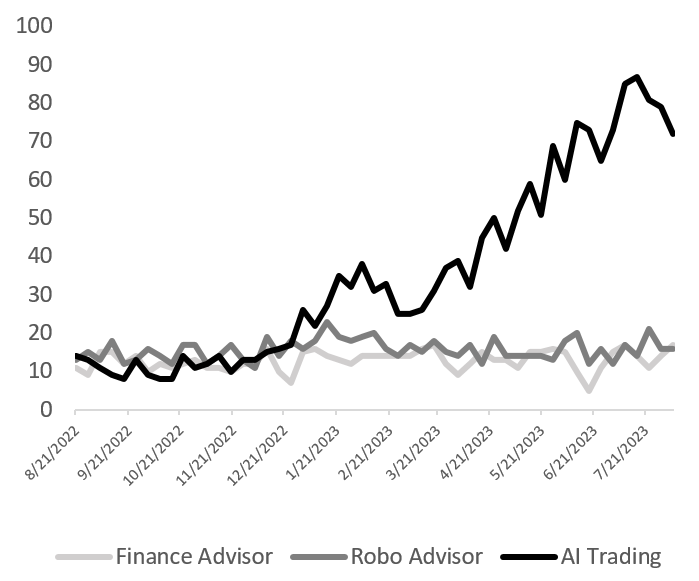
Figure 4 offers valuable insights into the dynamic preferences surrounding AI trading and financial advisory services. The depicted global trends showcase a consistent pattern; however, a closer examination reveals intriguing nuances.
The data indicates a noticeable divergence in the level of interest between Finance Advisors and Robo-Advisors. Finance Advisors command a notable interest score of 12.8 points, while Robo-Advisors register at 15.6 points. This suggests a preference for the automated and technologically driven approach of Robo-Advisors, even when faced with the typically higher costs associated with traditional Finance Advisors[36].
A noteworthy disparity emerges when specifically examining the interest in AI trading. Globally, AI trading garners a considerable interest score of 9.3 points, surpassing the interest in Robo-Advisors at 3 points. This signifies a distinct global enthusiasm for AI-driven trading solutions, potentially fueled by the allure of sophisticated algorithms and data-driven decision-making processes.
In summary, the trends illustrated in Figure 4 underscore the increasing prominence of AI trading in the financial technology landscape. The global preference for Robo-Advisors over traditional Finance Advisors, combined with the growing interest in AI trading, indicates a notable shift toward technologically advanced solutions in the financial advisory realm [36]. These insights not only mirror current user preferences but also provide valuable foresight into the trajectory of AI-driven financial services in the future (see Fig. 4 for illustration)
6. Development of Robo-Advisory Services
6.1. Full-Scale Optimization
The initial research focus revolves around the investment strategies employed by robo-advisors to serve their clients. Given the saturation within this sub-sector, the remaining robo-advisors face the imperative of distinguishing themselves from their competitors to both attract assets and maintain customer loyalty, thus heralding the anticipation of evolving investment methodologies [29].
Modern Portfolio Theory (MPT), initially embraced within this subsector, offered a well-established, Nobel Prize-backed approach known for its safety and appeal to investors seeking automated investment solutions. MPT is grounded in mean-variance analysis, striving to maximize expected returns while minimizing associated risks [52].
At its core, MPT employs mean-variance analysis, an approach that aims to strike a balance between maximizing expected returns and minimizing associated risks. Mathematically, it is represented as follows:
Here, Rp represents the expected portfolio return, wi signifies the weight of each asset in the portfolio, Ri is the expected return of each asset, σi denotes the standard deviation of each asset, ρij reflects the correlation between assets i and j, and σp is the standard deviation of the portfolio.
While MPT has been a valuable tool, the robo-advisory landscape has evolved, revealing its limitations. These limitations include an overreliance on data inputs, an undue concentration in specific asset classes, and the assumption of normal asset return distributions, which can be problematic in the presence of extreme returns.
Rather than shifting towards entirely new strategies, numerous firms are instead choosing to enhance the existing MPT investment framework, thus giving rise to the phenomenon known as the “Multidimensional Improvement of Modern Portfolio Theory” [29].
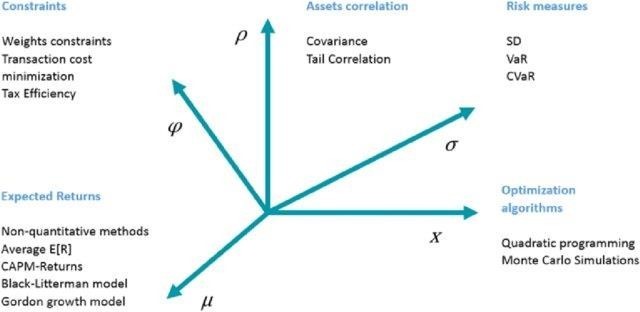
Figure 5 offers a summary of the multidimensional improvement of Modern Portfolio Theory (MPT), although it does not encompass all the methodologies currently being implemented by robo-advisors [29].
To overcome the limitations inherent in Modern Portfolio Theory (MPT), an advanced approach known as the “Multidimensional Improvement of Modern Portfolio Theory” (MPT+) has emerged. MPT+ goes beyond the traditional MPT framework by considering a broader array of factors, inputs, methods, and considerations in portfolio models. This extended framework is designed to overcome some of the constraints associated with MPT [53].
The MPT+ introduces an alternative formula for portfolio optimization:
In this formula, Ai represents alternative risk measures, and λ is a risk aversion parameter. By incorporating these additional risk measures, MPT+ offers a more nuanced approach to portfolio optimization, effectively addressing the limitations of traditional MPT [53]
As the robo-advisory landscape evolves, a growing disparity emerges between conventional investment strategies and newer, academically validated approaches. Modern Portfolio Theory (MPT), while widely adopted, reveals limitations such as data reliance, estimation errors, and over-concentration in specific asset classes within portfolios. It assumes normal asset return distributions, neglecting “fat tails” observed in empirical evidence, and overlooks crucial factors like time horizon and diverse financial circumstances [54-55].
MPT’s reliance on quadratic utility presents further challenges, assuming uniform emotions towards market volatility and an inaccurate representation of investor utility changes with wealth accumulation [4]. Alternative utility functions have been proposed, acknowledging that wealth benefits increase with growth, investors prefer upward market volatility, and relative risk aversion remains constant. Despite advantages, some argue these functions may oversimplify preferences, suggesting S-shaped or kinked utility functions for a more accurate representation [54-55].
Addressing these limitations, advanced strategies like Full-Scale Optimization, Risk Parity, Scenario Optimization, and Risk Parity with Skewness Risk have been introduced. Schwab Intelligent Portfolios has already implemented Full-Scale Optimization, showcasing practical advancements in the robo-advisory sector. Research suggests firms adopting these methodologies attract higher client investment levels [56].
As the robo-advisory sector evolves, these advanced strategies are poised to play a crucial role in meeting diverse investor preferences and optimizing portfolio performance. Particularly, the Multidimensional Improvement of Modern Portfolio Theory (MPT+) has gained traction, providing a more comprehensive and adaptable approach compared to traditional MPT, incorporating various risk measures, and accommodating different asset return distributions [57].
6.2. Digital Twin
Within the evolving landscape of robo-advisory, a new frontier emerges with the exploration of Digital Twin capabilities (Figure 6). This concept introduces the prospect of creating a virtual replica of the user’s environment, a digital twin that serves as the foundation for personalized advice delivered by robo-advisors [58]. It should be underscored that this approach remains speculative, warranting thorough regulatory examination to establish trust in the responsible use of consumer data.
The envisioned integration of Digital Twin capabilities into robo-advisor software marks a theoretical leap. Data extracted within the virtual environment shapes a digital replica. This conceptual duplicate becomes a tool for robo-advisors to tailor advice, utilizing insights gleaned from the user’s simulated surroundings [42].
The process can be summarized as:
Where DT represents the digital twin, and Data signifies the data collected from the user’s environment.
Digital twins hold promise in delivering more personalized recommendations compared to current robo-advisors. These replicas dynamically adapt guidance based on evolving client circumstances. For instance, if a client publicly shares on social media that they’ve become a parent, the robo-advisor could promptly suggest a college savings plan. As the software accumulates data, the digital twin’s understanding of the client’s financial situation becomes more comprehensive, enabling adjustments to their financial strategies [58]. The recommendations can be quantified as:
where recommendations denote advice from the digital twin, and Client Data represents data from the client’s virtual environment.
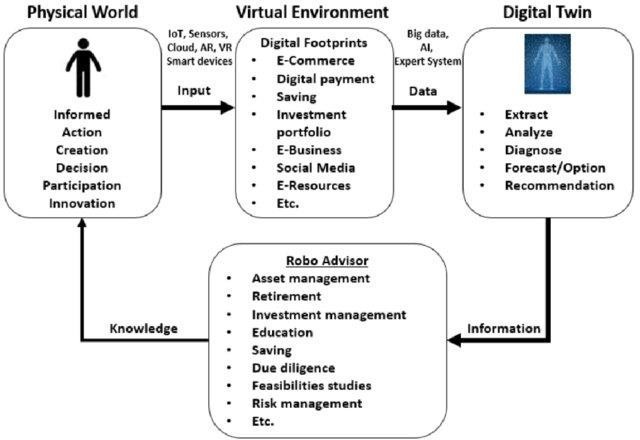
Leveraging insights from the digital twin, the robo-advisor can offer a spectrum of recommendations, including adjusting the client’s portfolio, providing guidance on insurance, suggesting new accounts, and delivering insights on significant purchases. Additionally, the robo-advisor can anticipate both near-term and long-term scenarios and recommend appropriate actions.
The digital nature of robo-advisors provides higher accessibility for clients with internet connectivity, a notable advantage over traditional human advisors. However, integrating digital twins into robo-advisory services poses challenges and risks, requiring robust data security measures to handle substantial volumes of sensitive client information and build trust in the technology[58].
6.3. Natural Language Processing
Natural Language Processing (NLP), a cornerstone of Artificial Intelligence (AI), is a crucial force bridging human language and machine comprehension. Rooted in Computational Linguistics, NLP enables machines to understand and manipulate human language, evolving beyond traditional predefined instructions [59].
The rise of Machine Learning and AI signifies a transformative era, empowering machines to make nuanced decisions based on real-time contextual information. This shift is evident in technologies like voice-activated virtual assistants (e.g., Alexa, Google Home), showcasing NLP’s role in enhancing human-machine interactions [59].
Figure 7 illustrates NLP’s profound impact on the financial landscape. Sourced from Analytics Vidhya, it exemplifies NLP’s transformative role in refining communication between users and robo-advisors, shaping the financial services landscape.
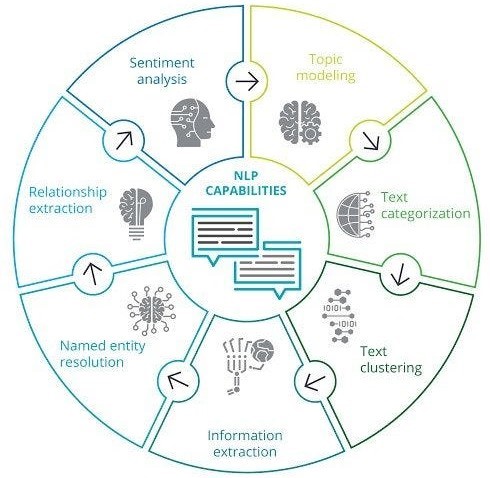
In the financial realm, grappling with time-consuming methods for document retrieval and extracting insights from vast, unstructured data has been a persistent challenge for decades. The intricate nature of unstructured data makes analysis and deciphering a formidable task. However, the emergence of Natural Language Processing (NLP) revolutionized this landscape, delivering unprecedented efficiency and accuracy [60].
NLP, prized for its innate ability to discern patterns within unstructured data, provides a profound advantage to financial institutions and, more recently, robo-advisers. Its applications span text classification, information retrieval, natural language generation, and natural language understanding. These capabilities collectively yield streamlined and powerful results, establishing NLP as an indispensable tool for automating numerous processes within the financial sector, particularly benefiting robo-advisers [61].
Robo-advisors in finance leverage Natural Language Processing (NLP) for significant enhancements:
- Personalized Customer Care: NLP-powered chatbots serve as 24/7 personal clerks for clients. They efficiently manage repetitive tasks and provide financial advice, aiding clients in saving, on-time payments, and overdraft prevention.
- Automated Trading Platforms: NLP is vital for high-frequency trading in robo-advisors. It enables the analysis of data, including news and press releases, to identify trading patterns. These tools operate almost in real-time, aiding brokers in validating trading decisions.
- Monitor Investor’s Sentiment: NLP tools track mentions of companies and assess sentiment in news coverage. Understanding the tone of news articles provides valuable insights into investor perceptions.
- Upgrade Quality of Analyst Reporting: NLP enhances analyst reporting by swiftly accessing relevant information. This enables analysts to create more detailed reports and offer superior advice.
- Easier Data Retrieval: Robo-advisors use NLP to implement conversational interfaces, allowing effortless searches within vast internal databases, even for complex queries.
These advancements in robo-advisors are powered by the capabilities of NLP, contributing to efficiency and effectiveness in various financial processes. That can be quantified as follows:
Let:
- PC: Personalized Customer Care efficiency.
- ATP: Efficiency in Automated Trading Platforms.
- MCS: Monitoring Company Sentiment.
- UQAR: Upgrade in Quality of Analyst Reporting.
- EDR: Easier Data Retrieval.
And:
- Total Benefits (TB) = PC + ATP + MCS + UQAR
+ EDR
Quantitative Representation:
TB = PC + ATP + MCS + UQAR + EDRbg
Where:
- PC is measured as the percentage increase in the efficiency of customer care and financial advice provided.
- ATP is quantified as the percentage improvement in trading efficiency.
- MCS is evaluated based on the accuracy of sentiment analysis and its impact on investment decisions.
- UQAR is expressed as the percentage increase in the quality of analyst reporting.
- EDR is assessed as the percentage reduction in the time required for data retrieval and its impact on decision-making processes.
By assigning values to each of these components, the overall benefits of implementing NLP in their robo-advisory services can be accurately quantified.
In [62], the authors underscores the potential of reciprocal conversations between human and robo advisors through AI chatbots to foster trust and enhance the perceived relationship between clients and RA firms. By investing in NLP technology on their platforms, RA companies can significantly enhance the user experience and cultivate customer loyalty. AI chatbots bring a conversational element that can potentially increase the likelihood of clients adhering to the portfolio guidance provided by robo-advisors, improve investors’ perceptions of the investment firm, and create a more positive and engaging experience for customers [62].
Furthermore, AI chatbots enable investors to obtain more personalized portfolio recommendations based on customer data. However, the preference for conversationalist RAs over non-conversational ones does raise potential ethical implications, as investors might be inclined to follow suboptimal investment decisions [62]. In [56], the athors delves into the advantages of AI-powered consultants for enhancing decision-making in businesses. It highlights the introduction of psychological barriers and emphasizes the potential risks associated with imbuing robo-counselors with social design elements to make them appear more human-like.
7. Regulating Robo-Advisors: Navigating Legal and Regulatory Frontiers
The rise of robo-advisors, internet-based advisory services driven by algorithms, has revolutionized the financial landscape, offering cost-effective solutions compared to traditional advisors. However, the unique client-machine interaction raises intricate legal questions, challenging regulators globally. This section explores the regulatory aspects surrounding robo-advisors, emphasizing their distinctive nature and the responses of major regulatory bodies [63].
Regulators, especially in major jurisdictions, grapple with adapting existing frameworks to address the peculiarities of robo-advisory services. While the European Union’s financial intermediary framework is seen as broadly applicable, the challenge lies in interpreting these rules without stifling innovation. Globally, regulators are cautious, often opting for a conservative stance. For instance, the U.S. Securities and Exchange Commission (SEC) issued guidance cautioning investors against sole reliance on robo-advisors, highlighting the need for individual research. Meanwhile, the Financial Industry Regulatory Authority (FINRA) in the U.S. expressed concerns about the adequacy of robo-advisor advice, emphasizing the importance of human intervention [64].
7.1. Elements of Robo-Advisory Regulation:
- Ongoing Duties, Compliance, and Authorization: The regulation of robo-advisors involves authorization by national regulators, aligning with the traditional framework for financial intermediaries. However, the challenge lies in ensuring that robo-advisors comply with ongoing duties and standards of care, particularly concerning communication with clients. Regulatory bodies globally, including the U.S. SEC and the Australian Securities and Investments Commission (ASIC), have issued guidance emphasizing the need for disclosure and investor protection [64].
- Collective Fallibility and Market Failure: Concerns surrounding market stability and systemic risks due to the collective decision-making processes of robo-advisors have been raised. While robo-advisors are yet to achieve a market share that poses a threat to systemic stability, regulators must be vigilant. The potential for instantaneous reactions during market crises and the risk of market distortions due to uniform advice patterns are areas where future regulatory focus may be warranted.
This section addresses concerns about the effectiveness of communication between robo-advisors and clients and delves into the need for a standard of investor protection equivalent to that for human advisors. It emphasizes that while robo-advisors need not be flawless, they should provide a similar level of investor protection as their human counterparts. The importance of recourse options for clients in case of flawed advice and the role of regulatory mechanisms in preventing or responding to such instances are underscored.
As robo-advisors continue to gain prominence, the regulatory landscape must evolve to accommodate their unique characteristics. While current frameworks offer a foundation, thoughtful interpretation, and adjustments are crucial to ensure a level playing field. Balancing innovation with investor protection remains a key challenge for regulators globally, urging ongoing dialogue and adaptability in the face of a dynamic financial landscape [63].
In conclusion, the regulatory framework for robo-advisors requires careful calibration to meet the evolving dynamics of the financial landscape. This section advocates for an investor protection standard akin to that applied to human advisors, recognizing the importance of addressing communication concerns and ensuring recourse options for clients. As the prominence of robo-advisors continues to grow, a nuanced and adaptable regulatory approach is paramount. Striking a balance between fostering innovation and safeguarding investor interests stands as an ongoing challenge, urging regulators to engage in continuous dialogue and adaptability. This proactive stance will contribute to the sustainable development of robo-advisory services in a rapidly changing financial ecosystem.
8. Pros and Cons of Robo-advisors
8.1. Pros
- Lower Costs Robo-advisors tend to have lower costs than traditional financial advisors because they operate more efficiently, reducing the workload of human advisors.
- Automated Investing Robo-advisors can automatically manage the investment portfolios of businesses, streamlining the investment process for enterprises and saving time.
- Efficient Financial Planning Robo-advisors can offer a more efficient financial planning process, aiding businesses in achieving their financial goals.
8.2. Cons
- Lack of Personalization Robo-advisors may not offer services as personalized as human consultants, potentially falling short of fully meeting the specific needs of businesses.
- Risk Management Robo- advisors may not provide sufficient expertise in risk management strategies for enterprises, necessitating additional support in this domain.
- Lack of Emotional Connection Robo- advisors may struggle to establish the same level of emotional connection as human consultants, which may result in a diminished human element in the consulting services offered to businesses.
As a result, robo-advisors can undoubtedly assist investors in constructing a more efficient business model, particularly in areas such as financial planning, investment, and risk management. However, they may encounter limitations when it comes to addressing their specific needs, and the absence of the human factor can potentially reduce the emotional connection in the consulting services provided.
9. Conclusive Remarks
In conclusion, this comprehensive analysis, rooted in the exploration of technological advancements within the robo-advisory sector, integrates insights from academia, industry reports, and emerging trends. The primary goal is to offer a technically grounded perspective that informs practical decision-making in a competitive landscape.
The recommendations emerging from this study herald a new era in robo-advisory technology. First and foremost, the adoption of advanced portfolio management methodologies, like Full-Scale Optimization (FSO), is pivotal for robo-advisory firms to gain a distinctive edge. Second, the incorporation of digital twin capabilities offers a future where ultra-personalized services are tailored to each unique client. Lastly, the infusion of Natural Language Processing (NLP) into robo-advisor software promises substantial enhancements in customer satisfaction, portfolio guidance adherence, and client-advisor relationships.
These implications reverberate across the spectrum of industry stakeholders, guiding firms, regulators, and investors. Firms can employ these technical recommendations to initiate conversations and explore the potential of integrating these technologies. Regulators responsible for oversight, must vigilantly monitor the development of these trends as robo-advisors evolve. For investors, this research provides a valuable framework to assess prospective platforms.
In the realm of research, our web-based design incorporated data gathered from platforms like statista.com, Google Trends, and developer reports. This study delved into key aspects, encompassing a global perspective of financial markets, an investigation into the status of technology within the global landscape of Robo-Advisors, and an exploration of opportunities for enhancing technology through the implementation of AI-driven solutions.
The pathway for future research extends towards delving into alternative investment strategies like Risk Parity, Scenario Optimization, and Risk Parity with Skewness Risk, as well as their adaptability within the robo-advisory sub-sector. Further studies can delve into the enduring impact of AI chatbots on the intricacies of the client-robo-advisor relationship, particularly as the younger generation inherits wealth and navigates its utilization.
While this study aims to offer technical insights, it encounters certain limitations. The availability of pertinent data points and the relatively nascent nature of the robo-advisory sector may constrain the breadth of current academic research. Nevertheless, this research sets the stage for technical innovation and paves the way for future explorations and advancements within the robo-advisory sub-sector.
Conflict of Interest
The authors declare no conflict of interest.
- Statista, Finance – Value and Forecasts, 2022. https://www.statista.com/outlook/dmo/app/finance/india [on 05.08.2023]
- G. Bayramoglu, An Overview of the Artificial Intelligence Applications in Fintech and Regtech, 291–298, 2021, doi:10.1007/978-981-33-6811-8 15.
- D. Belanche, L. V. Casal, C. Flavi ́an, “Artificial Intelligence in FinTech: understanding robo-advisors adoption among customers,” Industrial Management Data Systems, 119, 1411–1430, 2019, doi:10.1108/IMDS-08-2018-0368.
- M. I. Bonelli, E. S. Dongul, “Robo-Advisors in the Financial Services Industry: Recommendations for Full-Scale Optimization, Digital Twin Integration and Leveraging Natural Language Processing Trends,” 268–275, IEEE, 2023, doi:10.1109/ICVR57957.2023.10169615.
- L. D. Bernardis, ROBO ADVISORY Artificial intelligence applied to finance: An industry-specific analysis, comparing incumbents to startups: the Money-Farm vs YellowAdvice, LAP LAMBERT Academic Publishing, 2021.
- Deloitte, “2021 investment management outlook,” https://www2.deloitte.com/jp/en/pages/financial-services/articles/im/2021- investment-management-outlook.html, 1–26, 2021.
- K. Wahabi, Lakhchini, Rachid Wahabi, “Artificial Intelligence Machine Learning in Finance: A literature review,” International Journal of Accounting, Finance, Auditing, Management and Economics, 3, 437–455, 2022, doi: 10.5281/zenodo.7454232.
- A. Bhatia, A. Chandani, R. Atiq, M. Mehta, R. Divekar, “Artificial intelligence in financial services: a qualitative research to discover robo-advisory services,” Qualitative Research in Financial Markets, 13, 632–654, 2021, doi:10.1108/QRFM-10-2020-0199.
- V. Joshi, Dr. Manjari Sharma, “Artificial Intelligence and Financial Decision Making – Review of efficacy in Usage within Financial Organizations in India,” Turkish Online Journal of Qualitative Inquiry (TOJQI), 12, 12138–12160, 2021.
- R. Manrai, K. P. Gupta, “Investor’s perceptions on artificial intelligence (AI) technology adoption in investment services in India,” Journal of Financial Services Marketing, 28, 1–14, 2023, doi:10.1057/s41264-021-00134-9.
- Nain, S. Rajan, “Algorithms for better decision-making: a qualitative study exploring the landscape of robo-advisors in India,” Managerial Finance, 49, 1750–1761, 2023, doi:10.1108/MF-01-2023-0055.
- M. Shanmuganathan, “Behavioural finance in an era of artificial intelligence: Longitudinal case study of robo-advisors in investment decisions,” Journal of Behavioral and Experimental Finance, 27, 100297, 2020, doi:10.1016/j.jbef.2020.100297.
- M. Sarea, A. H. Elsayed, S. A. Bin-Nashwan, Artificial Intelligence and Islamic Finance, Routledge, 2021, doi:10.4324/9781003171638.
- W. L. Shih, C. Wei, H. Lin, P. Chang, “RETRACTED: Selecting and balancing market portfolio using artificial intelligence and fuzzy Mult objective decision-making model,” Concurrent Engineering, 30, 382–398, 2022, doi:10.1177/1063293X221115768.
- G. Babaei, P. Giudici, E. Raffinetti, “Explainable artificial intelligence for crypto asset allocation,” Finance Research Letters, 47, 102941, 2022, doi: 10.1016/j.frl.2022.102941.
- S. Deo, “The Under-appreciated Regulatory Challenges posed by Algorithms in FinTech. Understanding interactions among users, firms, algorithm decision systems regulators,” 1–299, 2022, doi:10.48462/opus4-4288.
- Burnmark, “Digital Wealth,” 2017. https://www.burnmark.com/uploads/reports/Burnmark_Report_Apr17_ D igital_Wealth.pdf (accessed on 1/16/2023).
- W. Lam, “Robo-Advisors: A Portfolio Management Perspective,” 1–105, 2016.: https://economics.yale.edu/sites/default/files/2023- 01/Jonathan_Lam_Senior%20Essay%20Revised.pdf
- Bjerknes, A. Vukovic, A. A. Gaivoronski, “Automated advice: A portfolio management perspective on robo-advisors,” 2017.
- Backend Benchmarking, “The Robo Report and Robo Ranking. The Robo Report.” 2022. https://www.theroboreport.com/ (accessed on 01/18/ 2023)
- S. Utkus, A. Rossi, “Who Benefits from Robo-advising? Evidence from Machine Learning,” 2020.: https://papers.ssrn.com/sol3/papers.cfm?abstract_id=3552671.
- D. Jung, V. Dorner, F. Glaser, S. Morana, “Robo-Advisory,” Business Information Systems Engineering, 60, 81–86, 2018, doi:10.1007/s12599-018-0521-9.
- R. Tao, C. Su, Y. Xiao, K. Dai, F. Khalid, “Robo advisors, algorithmic trading and investment management: Wonders of fourth industrial revolution in financial markets,” Technological Forecasting and Social Change, 163, 120421, 2021, doi:10.1016/j.techfore.2020.120421.
- B. Barber, Y. Lee, Y. Liu, T. Odean, “Just How Much Do Individual Investors Lose by Trading?” Review of Financial Studies, 22, 609–632, 2009, doi:10.1093/rfs/hhn046.
- W. N. Goetzmann, A. Kumar, “Equity Portfolio Diversification*,” Review of Finance, 12, 433–463, 2008, doi:10.1093/rof/rfn005.
- FROOT, D. Scharfstein, J. C. Stein, “Herd on the Street: Informational Inefficiencies in a Market with Short-Term Speculation,” The Journal of Finance, 47, 1461–1484, 1992, doi:10.1111/j.1540-6261.1992.tb04665.x.
- S. Chandler, “Robo-advisors offer automated investing services at a low cost —here’s how to tell if they’re right for you,” 2020: https://www.businessinsider.com/personal-finance/what-is-a-robo- advisor (accessed on 1/17/2023).
- F. D’Acunto, N. Prabhala, A. G. Rossi, “The Promises and Pitfalls of Robo-Advising,” The Review of Financial Studies, 32, 1983–2020, 2019, doi:10.1093/rfs/hhz014.
- Research, Markets, “Robo-advisory Services Market – Growth, Trends, COVID-19 Impact, and Forecasts (2022 – 2027),” 2022.: https://www.researchandmarkets.com/reports/5120011/roboadvisory- services-market-growth-trends (accessed on 01/18/ 2023).
- R. Dive, “The Use of Digital Technology and Automation in Wealth Management are creating Growth Opportunities for the Robo Advisory Market Globally,” 2022.
- J. P. Costa, “Vanguard research Quantifying the investor’s view on the value of human and robo-advice,” 2022. :https://advisors.vanguard.com/iwe/pdf/ISGHVD.pdf (accessed on 02/18/ 2023).
- B. Benchmarking, “The Robo Report and Robo Ranking.” 2022. : https://www.theroboreport.com/ (accessed on 02/20/ 2023)
- Beketov, K. Lehmann, M. Wittke, “Robo Advisors: quantitative methods inside the robots,” Journal of Asset Management, 19, 363–370, 2018, doi:10.1057/s41260-018-0092-9.
- Anshari, M. N. Almunawar, M. Masri, “Digital Twin: Financial Technology’s Next Frontier of Robo-Advisor,” Journal of Risk and Financial Management, 15, 163, 2022, doi:10.3390/jrfm15040163.
- M. B. A. Vasylchenko, “Web research and Google Trend data for Robo-Advisors study,” 2023.
- M. L. Sette, “Best Robo-Advisors. Investopedia. Reviewed by S. Silberstein. Fact checked by M. M. Klammer,” 2023. https://www.investopedia.com/best-robo-advisors-4693125
- Benson, “12 Best Robo-Advisors of August 2023,” 2023.
- T. Tepper, “5 Best Robo-Advisors Of August 2023. Forbes Advisor,” 2023. https://www.nerdwallet.com/best/investing/robo-advisors
- M. Frankel, “Best Robo-Advisors for August 2023,”. https://www.fool.com/the-ascent/buying-stocks/best-robo-advisors/
- E. Gravier, “The 5 Best Robo-Advisors When You Want to Be Hands Off with Your Investments. CNBC,” 2023. https://www.cnbc.com/select/best-robo-advisors/
- D. Sherarrow, “Best Robo Advisors in the U.S. August 2023,” 2023. https://www.investing.com/brokers/robo-advisors/
- I. Pino, “The Top Robo-Advisor Offerings for 2023.” 2023. https://fortune.com/recommends/investing/best-robo-advisors/
- M. C. Zinn, “Best Robo-Advisors for Investing for August 2023,” 2023. https://www.cnet.com/personal-finance/investing/advice/best-robo-advisor/
- J. Fell, “Best Robo Advisory: Top 6 Robo Advisors (2023),” 2023. https://www.entrepreneur.com/guide/investing/robo-advisors
- F. Allen, G. Xian, “A survey of fintech research and policy discussion,” 2021, doi:10.21799/frbp.wp.2020.21.
- M. I. Bonelli, “Macroeconomic Factors and SENSEX Performance in India: Unveiling the Post-Liberalization Era (1980-2020),” Indian Journal of Research in Capital Markets, Forthcoming, 2023.
- L. D. Bernardis, “Artificial intelligence applied to finance: Robo advisory: An industry-specific analysis, comparing incumbents to startups in business model sustainability: MoneyFarm vs YellowAdvice,” 2020.
- Deloitte, “2021 investment management outlook,”: https://www2.deloitte.com/content/dam/Deloitte/tr/Documents/financial-services/2021-investment-management-outlook.pdf.
- X. Cheng, F. Guo, J. Chen, K. Li, Y. Zhang, P. Gao, “Exploring the Trust Influencing Mechanism of Robo-Advisor Service: A Mixed Method Approach,” Sustainability, 11, 4917, 2019, doi:10.3390/su11184917.
- BLNC, “BLnC Invest,”. https://www.blincinvest.com
- T. Idzorek, A step-by-step guide to the Black-Litterman model, 17–38, Elsevier, 2007, doi:10.1016/B978-075068321-0.50003-0.
- R. O. Michaud, “The Markowitz Optimization Enigma: Is ‘Optimized’ Optimal?” Financial Analysts Journal, 45, 31–42, 1989, doi:10.2469/faj.v45.n1.31.
- M. Best, R. Grauer, “On the Sensitivity of Mean-Variance-Efficient Portfolios to Changes in Asset Means: Some Analytical and Computational Results,” Review of Financial Studies, 4, 315–342, 1991, doi:10.1093/rfs/4.2.315.
- T. Adler, M. Kritzman, “Mean-variance versus full-scale optimization: In and out of sample,” Journal of Asset Management, 7, 302–311, 2007, doi: 10.1057/palgrave.jam.2250042.
- C. Back, S. Morana, M. Spann, “When do robo-advisors make us better investors? The impact of social design elements on investor behavior,” Journal of Behavioral and Experimental Economics, 103, 101984, 2023, doi:10.1016/j.socec.2023.101984.
- M. Beketov, K. Lehmann, M. Wittke, “Robo Advisors: quantitative methods inside the robots,” Journal of Asset Management, 19, 363–370, 2018, doi:10.1057/s41260-018-0092-9.
- M. Anshari, M. N. Almunawar, M. Masri, “Digital Twin: Financial Technology’s Next Frontier of Robo-Advisor,” Journal of Risk and Financial Management, 15, 163, 2022, doi:10.3390/jrfm15040163.
- B. Mishra R. Agrawal, “Natural Language Processing in Artificial Intelligence,” Apple Academic Press, 2019.
- T. Rouzbeh A. Shirvani, Y. Keneshloo, “Natural Language Processing Advancements By Deep Learning: A Survey,” https://arxiv.org/, 2021, doi:10.48550/arXiv.2003.01200.
- R. Kliman, B. Arinze, Cognitive Computing: Impacts on Financial Advice in Wealth Management, 11–23, 2019, doi:10.1007/978-3-319-93299-6 2.
- C. Hildebrand, A. Bergner, “Conversational robo advisors as surrogates of trust: onboarding experience, firm perception, and consumer financial decision making,” Journal of the Academy of Marketing Science, 49, 659–676, 2021, doi:10.1007/s11747-020-00753-z.
- Maume, “Regulating Robo-Advisory,” SSRN Electronic Journal, 2018, doi:10.2139/ssrn.3167137.
- M. L. Fein, Regulation of Robo-Advisers in the United States, 105–131, 2021, doi:10.1007/978-3-030-40818-3 7.
- R. Steennot, “Robo-advisory services and investor protection,” Law and Financial Markets Review, 15, 262–277, 2021, doi:10.1080/17521440.2022.2153610.