Efficacy of multiple anticancer therapies may depend on host immune response
Volume 2, Issue 3, Page No 773-787, 2017
Author’s Name: Kritika Karri1, 2, a), Dhundy R Bastola1
View Affiliations
1University of Nebraska at Omaha, College of Information Science and Technology, 68182, USA
2Boston University, Bioinformatics Graduate Program, 02215, USA
a)Author to whom correspondence should be addressed. E-mail: kkarri@unomaha.edu
Adv. Sci. Technol. Eng. Syst. J. 2(3), 773-787 (2017); DOI: 10.25046/aj020398
Keywords: Biomarker, Immune response, Progressive Disease, Complete Remission, Lung adenocarcinoma, Colon adenocarcinoma, Alkylating agents, Antimetabolite, Mitotic inhibitors, Anti-angiogenesis, GSEA
Export Citations
The host immune system is a key player in anticancer therapy response and resistance. Although the impact of host immune response in the ‘war against cancer’ has been studied and it has been the basis for immunotherapy, understanding of its role in attenuating the action of conventional anticancer therapies is an area that has not been fully explored. In spite of advances in systemic therapy, the 5-year survival rate for adenocarcinoma is still a mere 13% and the primary reason for treatment failure is believed to be due to acquired resistance to therapy. Hence, there is a need for identifying reliable biomarkers for guided treatment of lung and colon adenocarcinoma and to better predict the outcomes of specific anticancer therapies. In this work, gene expression data were analyzed using public resources and this study shows how host immune competence underscores the efficacy of various anticancer therapies. Additionally, the result provides insight on the regulation of certain biochemical pathways relating to the immune system, and suggests that smart chemotherapeutic intervention strategies could be based on a patient’s immune profile.
Received: 05 April 2017, Accepted: 16 May 2017, Published Online: 16 June 2017
1. Introduction
Cancer immunology has been studied and researched over many years to unravel the key mechanisms that determine therapeutic synergy or antagonism for cancer patients based on individual host immune environments. Traditional chemotherapy and other targeted therapies have been effective in combating many types of cancer. However, the efficacy of these interventions is determined by off-target effects within the host immune system [1]. Review of the literature shows that host immune response is key to the success of anticancer therapies through a process known as immune-surveillance [2].
Studies have shown that type-1 interferon can convert tumor associated macrophages (TAMs) into tumor-antagonizing macrophages by up-regulating the expression of dendritic cells in breast cancer. This results in increased CD8+ T cell population with reduced tumor growth and metastasis. As such, the combination of chemotherapy together with drugs that repolarize TAMs may be exploited to achieve greater patient responses and prevent resistance mechanisms within the immune system [3]. Some chemotherapeutics skew the polarization of macrophages directly, or indirectly via regulating cancer cell secreted factors [4]. FOXP3 expressing regulatory T cells (Tregs) are well-known for their immunosuppressive function in tumors [5]. They are a subset of CD4+ T cell population, which are involved with adaptive immune response. Tregs are known to be sensitive to cyclophosphamide and their depletion improves the potency of platinum-containing chemotherapeutic drugs against lung adenocarcinoma [6].
Host immune response is known to significantly regulate the response of anti-angiogenic therapies [7]. Studies suggests that TAMs counteract the potency of anti-angiogenic agents; one such example is anti-angiopoietin 2 (ANGPT2), which decreases the blood vessel density of tumor and promote its remission. Interestingly, it also prevents the TIE2-expressing macrophages from associating with endothelial cells [8]. Together, these findings support the rationale for combining anti-angiogenic drugs with macrophage-targeting strategies to increase the efficacy of the former, particularly in tumors that develop resistance to anti-VEGF therapy. Despite the scientific progress in the field, a complete understanding of the role of the host immune system’s response in improving the efficacy of anticancer therapies is greatly needed. There has been no systematic analysis carried out to evaluate the efficacy of different anticancer treatments based on host immune profile and subsequent application of this knowledge to develop reliable biomarkers to predict how well patients will respond to a treatment interventions.Another study has shown that Interleukin-17 (IL-17) producing CD4+ T cells limit the ability of 5-Flouro Uracil (5-FU) to delay the growth of subcutaneous thymoma cells. In contrast, IL-17 elevates the therapeutic efficacy of doxorubicin in sarcoma. Hence, the mode of action of these CD4+ T cell is paradoxical and depends widely on the cancer type [6]. However, their manipulation may be a useful strategy to determine the efficacy of chemotherapy.
Lung cancer continues to be the leading cause of death in industrialized countries and most commonly occurring disease among smokers. It can be broadly categorized into small cell lung cancer (SCLC) and non-small cell lung cancer (NSCLC). About 80-89% of patients suffer from the latter with most common being lung adenocarcinoma (LUAD), which has the average survival rate of 12-15% [9]. It develops on the outer parts of the lung or ‘in-situ’ and tends to grow slower than other types of cancer resulting in late-stage detection and a paucity of late stage treatments.
Colon adenocarcinoma (COAD) is the third most common cause of cancer mortality worldwide and fourth in the United States. Even though the 5-year survival rates have improved over the last 3 decades with ~95% in stage-I, it still remains low for stage III (~61%) and reaches only 8% for patients in stage IV. The treatment regimen involves mainly two classes of chemotherapy- alkylating agents and antimetabolites. However, the therapy is often insufficiently effective, with some patients exhibiting strong chemo-resistance. Therefore, host immune-competency plays a vital role to promote immunosurvelliance and disease regression [10].
Chemotherapy is one of the most common interventions for cancer care. It uses certain drugs to kill cancer cells or to stop them from growing and spreading to other parts of the body. This therapy works by damaging the DNA inside the nucleus of the transformed cells. While some drugs damage cells at the point of cell division, others damage the cells during DNA replication prior to division. Based on these differences in the mechanism of action, the chemo drugs can be classified into three main therapy types [11]:
(i) Alkylating agents: This class of drug directly targets DNA to repress a cell from reproducing. These drugs are not cell cycle specific and can be used for wide range of cancers.
(ii) Antimetabolites: This class of drug interferes with DNA and RNA synthesis by substituting for the normal building blocks of nucleotides. These agents damage cells during the S phase of cell cycle, when the cell’s chromosomes are being copied.
(iii) Mitotic inhibitors: This class of drug is primarily derived from plant alkaloids and other natural products, and they operate by stopping mitosis in the M phase of the cell cycle by disrupting microtubule formation.
(iv) Anti-Angiogenesis: This class of drug is most widely used in the domain of vascular-mediated therapy [12], which is another class of treatment strategy that involves the impairment of tumor nutrition by targeting tumor blood vessel.
The single gene analysis method has been instrumental in our understanding of cell-biological processes, especially in elucidating the correlation between mutation and cancer. However, with increased understanding of the disease etiology, we have come to realize that it is not usually a single gene but a set that contribute to the clinical manifestation of cancer. Hence, it is more relevant to study the changes initiated by a set of genes, which can dramatically alter various cell biological and metabolic pathways. Along this vein the commonly used approaches to analyze a geneset are by over representation or aggregate score calculation. The latter is more reliable as it calculates the aggregate score for each geneset based on the gene-specific scores for that geneset and overcomes the limitations of over-representation analysis, which relies on the cutoff used in generating the geneset and can vary considerably depending on the gene list [13].
High throughput data like RNA-seq and microarray have fueled knowledge-based approaches like Gene Set Enrichment Analysis (GSEA), with access to publically available datasets for gene expression and clinical outcomes from TCGA. GSEA is widely used in studies that compare and contrast two conditions (for example disease vs. normal states) and usually focuses on a handful of genes that are either up or down regulated in the process. Therefore, in the present study we used GSEA to extract significant information at the level of functionally related geneset between the two clinical conditions (Fig 1.).
While GSEA provides a ‘bird’s eye view’ of interactions between the drug treatment and the geneset significantly overrepresented in the phenotype being compared, not all the members in the geneset contribute equally to attain significant enrichment. As described by Subramanian et al., there are leading edge subsets of genes within the set that appear in the ranked-list before the point at which the running sum reaches its maximum deviation from zero [14]. These sets of genes are called ‘leading edge genes’ as they contribute more to the enrichment score of a geneset during geneset enrichment analysis. A gene that is in many of the leading edge subsets is more likely to be of higher significance or interest compared to other genes. called ‘leading edge genes’ as they contribute more to the enrichment score of a geneset during geneset enrichment analysis. A gene that is in many of the leading edge subsets is more likely to be of higher significance or interest compared to other genes.
In the current study, we used publically available datasets from TCGA for lung and colon adenocarcinoma samples along with the therapy regimen and response type – Progressive Disease (PD) (ineffective therapy with continued growth and spreading of cancer), and Complete Remission (CR) (state of disappearance of all signs of cancer in response to a treatment regimen) – to come up with putative biomarkers specific for both cancer types and different anticancer therapies using GSEA. Finally, to determine the prognostic ability of these biomarkers, we performed survival analysis.
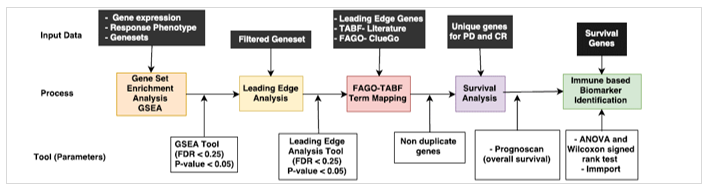
2. Material and Methods
2.1. Data Access and Processing
The Cancer Genome Atlas (TCGA) public resource was used to download RNAseq gene expression datasets (level 3) for lung adenocarcinoma (LUAD) and colon adenocarcinoma (COAD). The expression set was quantified using RSEM method (RNA-seq by expectation maximization). Additionally, the genesets were obtained from MsigDB, which was explicitly integrated with the GSEA tool [15]. Five geneset categories, namely, hallmark, canonical pathways (CP), GO genesets (C5), oncogenic signatures (C6) and immunologic signatures (C7) were selected from the MsigDB collection, which included genes involved in cancer, immunology and biochemical pathways. The information relating to therapeutic interventions including drug name and response type for these patients was obtained from Nationwide Children’s Hospital, which serves as the Biospecimen Core Resource (BCR) for TCGA.
The clinical data for lung adenocarcinoma consisted of four therapies discussed in the previous section, whereas alkylating agents and antimetabolites were the most preferred intervention for colon adenocarcinoma [16]. The drug information and patient response were then mapped with gene expression data using the TCGA ID as the primary identifier as shown in Table 1.
Table 1. Four therapy-classes were identified based on the information obtained from BCR for TCGA- LUAD tumor samples. Each named-drug used on the patients are listed under respective therapy-class.
2 GSEA
A Java based GSEA tool [14] that incorporated genesets from MsigDB was used with 3 required inputs, namely- (a) expression file (.txt format), (b) phenotype files, which provided therapy response (PD and CR) (.cls format) and (c) genesets obtained from MsigDB (.gmt format). A geneset permutation with the default value of 1000 was performed along with other parameters set to default for the analysis. The result of the analysis consisted of enriched genesets in PD and CR with their corresponding enrichment score (ES), p-value and FDR value. The genesets with p-value < 0.05 and FDR value < 0.25 (both PD and CR) were chosen for the leading edge analysis as shown in Figure 1.
2.3 Leading Edge Genes
We performed leading edge analysis on the enrichment results from GSEA, to identify genes that were significantly enriched across the PD vs. CR in LUAD and COAD. The leading edge analysis was carried out for genesets that were significantly enriched in the GSEA (FDR < 0.25, p-value < 0.05) across different geneset categories (C5, CP, hallmark, C7 and C6) and for all anticancer therapies. The leading edge genes obtained from the analysis were merged for each geneset category into the PD upregulated and CR upregulated groups.
2.4 Functional Association of Leading Edge Genes with GO terms (FAGO)
In order to extract functional annotation for the leading edge genes we used ClueGo, a Cytoscape plugin [17] that gives biological interpretation and systematic organization for a large list of genes. The list of leading edges genes for each anticancer therapy (for both PD and CR separately) was provided to ClueGo to obtain a list of gene-clusters that are functionally associated with a specific GO functional categories including biological processes (BP), cellular component (CC), molecular function (MF) and immune based terms. From a large list of GO terms, the terms that had significant p-value and high percentage term association were extracted and labeled as ‘functionally associated GO terms’ (FAGO). There were several repetitive terms which performed similar function. Merging of all such FAGO terms helped us in downstream analysis.
2.5 Therapy Associated Biological Functions (TABF)
Each anticancer therapy has a different mechanism of action to stop the cancer cells from growing; each mechanism evokes perturbations in a complex system with several immunological processes and biological pathways involved. To evaluate the efficacy of each intervention, a comprehensive understanding of the mode of drug-action and the processes/pathways affected is necessary. Therefore, we searched the literature for concepts related to action mechanism in each drug category which were known to affect the efficacy of the therapy. These concepts/terms were labeled as ‘therapy associated biological functions’ (TABF).
2.6 Mapping of TABF with FAGO terms
To identify relevant biological function affected by a therapy, we integrated TABF term for each therapy with related FAGO terms. While TABF term provided experimental evidence through the literature, FAGO defined concepts used to describe gene function. For instance, STAT3 signaling is known to be involved with tumorigenesis in all chemotherapies [18]. Here ‘STAT3 Signaling’ is TABF and it is mapped with all the related GO terms (FAGO terms) present in PD.
2.7 Extraction of Unique Genes and Survival Analysis
The genes associated with mapped FAGO–TABF terms were retrieved for both disease outcome phenotype (PD and CR) as well as for all therapy groups. The duplicate gene entries were discarded and the list of genes from each therapy were compared to determine common and mutually exclusive genes between all therapies in both phenotypes (PD and CR). Survival analysis was performed for genes unique to each therapy using PrognoScan – a survival prediction tool with a collection of publicly available cancer survival data [19]. A list of genes specific to each treatment and phenotype was provided as input for the PrognoScan tool [20]. The survival performance was evaluated based on p-value, where a value < 0.05 indicated good overall survival while a greater value indicated poor survival. The genes obtained from survival analysis were further evaluated for immune related function using Immport which is a comprehensive list of immune related genes [21].
2.8 Study of Expression Variance for Biomarker Prediction
We performed statistical tests (ANOVA and Wilcoxon signed rank test) to detect putative biomarker genes whose expression significantly varied across the two phenotypes (PD vs CR) for each anticancer therapy in LUAD and COAD samples.
3. Results
3.1. Data
As shown in Table 1. 129 samples along with therapy information were obtained from BCR-TCGA for LUAD. Alkylating agents had the highest number of samples (60) due to its wide drug target spectrum, whereas 42 samples were divided amongst alkylating agents and antimetabolite therapy for COAD with clinical outcome (PD vs. CR) as shown in Table 1 with almost equal number of samples.
3.2. Gene Set Enrichment Analysis (GSEA)
Gene sets were filtered based on the p-value cutoff (<0.05) and FDR (<0.25), and we found significantly higher enrichment for complete remission in all four therapies for LUAD as reported in Table 2. High enrichment of oncogenic signatures (C6) in CR phenotype were reported for antimetabolite (PD: 0, CR: ~53%), mitotic inhibitor (PD: ~5%, CR: ~24%) and anti-angiogenesis (PD: 0, CR: ~58%). No significant enrichment was recorded for canonical pathway (CP) in both PD and CR for alkylating agents. However, in COAD oncogenic signature geneset (C6), PD phenotype was highly enriched in both therapies and a similar trend was observed for the hallmark geneset category as shown in Table 2. That said, the C7 category (immunogenic signatures) was equally enriched in PD and in CR for alkylating agents. Between lung and colon adenocarcinomas, we found higher overall enrichment for PD in COAD as opposed to LUAD.
Table 1. Distribution of LUAD and COAD samples based on therapy and outcome (PD vs CR) obtained from BCR-TCGA.
Table 2. Enrichment percentage for significantly enriched (p< 0.05, FDR < 0.25) genesets from GSEA across PD vs. CR in each anticancer therapy for LUAD and COAD samples.
3.3. Leading Edge Genes
The next step following the GSEA analysis was to identify a subset of the genesets that accounted for the enrichment signal. This was the leading-edge analysis, which resulted in identifying high scoring genesets that contributed to the enrichment signal. The numbers above each of the bars in Figure 2 represent the sum of such core genes from all 5 geneset categories that resulted from the leading edge analysis. These results show a high number of genes in the geneset that contribute to the enrichment score. In case of LUAD, fewer leading edge genes were enriched across CR compared to PD in alkylating agents as opposed to the other three therapies. The highest numbers of leading edge genes were recorded for anti-angiogenesis in CR (4688), where the numbers were almost comparable in mitotic inhibitor (4115) and antimetabolite (4232). Antimetabolite therapy had the least number of leading edge genes in PD (91). This observation is in agreement with the geneset enrichment result shown in Table 2, where the overall enrichment percentage for antimetabolite therapy for CR in each geneset category was higher as opposed to the PD phenotype.
This trend was reversed in the case of COAD, where large number of leading edge genes enriched across PD for both the alkylating agents (PD:4495, CR:214) and antimetabolite therapies (PD:4907, CR:765) as shown in Figure 2. This result is consistent with the GSEA results shown in Table 2.
3.4. TABF-FAGO term mapping
We next sought to identify the functions associated with the genes within various enriched genesets. The Functionally Associated GO-terms (FAGO) for all the genesets were obtained, using ClueGO analysis. The number of genes associated with each of the GO terms is shown in Table 3. Majority of the FAGO terms belong to biological processes (BP) or immune-related processes for both LUAD and COAD. The TABF terms and mapped FAGO terms are reported in Tables 1-12 in Appendix I section and have been explained in detail in the discussion section.
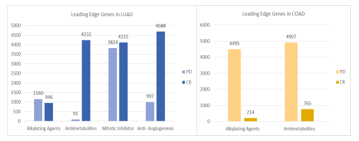
3.5. Survival analysis and Biomarker Discovery
The set of non-repetitive genes from each TABF-FAGO term mapping was extracted for survival analysis. However, due to the interest in seeking specific biomarkers for each therapy, only genes that were unique and non-overlapping between the anticancer therapies were extracted. The result showed 120 common leading genes across all four therapies for CR and 0 genes common across PD in LUAD. Similar to the analysis of genes unique to the therapy, analysis of these 120 common genes was also completed as shown in Figure 3. However with COAD data, both alkylating agents and antimetabolite therapies target the cell cycle and are involved in its disruption, which explains the large number of overlapping genes for both PD (103 genes) and CR (l107 genes) as shown in Figure 3.
Table 3. The number of FAGO terms mapped with TABF terms in each GO term category for all the anticancer therapies across PD and CR for LUAD and COAD samples.
The unique list of genes from each anticancer therapy from PD and CR were analyzed for overall survival for LUAD and COAD datasets using PrognoScan based on the p-value threshold (0.05) for good and poor survival as shown in Figure 4.
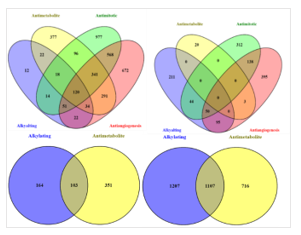
Simultaneously, the number of immune-related genes in each case using a comprehensive list of known immune related genes from Immport was evaluated. Results show a good percentage of immune related genes. Of all these total genes involved in good and poor survival, ~39% genes (in CR) ~48% genes (in PD) for LUAD, and ~42% genes (in PD) and ~14.6% (in CR) for COAD were related to immune response as shown in Table 4.
3.6. Expression Variance
The gene expression data was used in statistically analyzing expression variance across the PD vs. CR for all survival genes using ANOVA and Wilcoxon ranked test. The immune-related genes, which showed significant expression variance (p-value < 0.05), are presented in Table 5 for LUAD and Table 6 for COAD. In lung adenocarcinoma, the data resulted in 2 immune-related biomarkers for alkylating agents and 6 for antimetabolites, 17 gene biomarkers for mitotic inhibitors, and 33 gene biomarkers
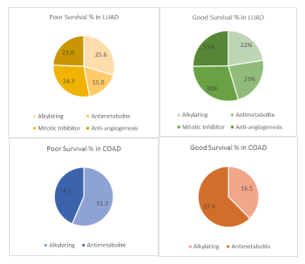
for anti-angiogenesis, as shown in Table 5. For colon adenocarcinoma, 5 biomarkers for alkylating agents and 8 expression biomarkers for antimetabolites were seen in Table 6. The expression biomarkers are represented as boxplots in Figure 5 and Figure 6, where a clear distinction between PD upregulation compared to CR for DEF126 in alkylating genes are seen for LUAD, while JUNB has very high overall expression with high variance for PD as compared to CR. In antimetabolites, we found a good differentiation in expression for two groups: PD vs. CR response phenotype, for all the 6 genes including SIVA1, DNM1 and TNFRSF14, which shows a significant variance with high expression for CR as shown in Figure 5. The highest number of gene expression biomarkers were found in mitotic inhibitors, with a mixture of PD and CR upregulated genes with immune-based function. SRGN, MAPT, ABR, NPBL, STXPB1, NFE2L1, AATF, and VEZF1 were genes with striking difference in expression across PD and CR. The anti-angiogenesis therapy had 17 genes with striking expression variance, with 3 genes upregulated for PD and 14 for CR. A significant expression variance was seen across PRAKAA2, F13A1, NUPR1, RAPGEF4 and STK38L in PD compared to CR.
In COAD, we found 35 significant immune-related biomarkers – NPPB, S100A3, PPBP, LCNL1, PRDX2, TNFRSF11A, S100G, LEFTY1, IL13RA2, CXCR1, S100A12, FAM3C, CCK, PSMD14, PROK2, IL20RB, S100A9, WNT5A, TNFRSF11B, INHBC, APLN, MALT1, IFNE, RLN2, KLRC2, NCR1, KLRC3, PSPN, CRLF3, IL15, CDH1, COLEC10, GAST, SP1, and CRABP2 were upregulated for PD while two genes, IL18RAP and IL24, were significantly upregulated for CR in alkylating agents. In antimetabolite therapy, 8 immune-related biomarkers were indicated: EIF2AK2, FABP5, FCN2, HRAS, IL10, NAMPT, SEMA4G, and TAP2 were upregulated for PD, and three genes, IGF2R, NOV, and IL17C, were upregulated for CR as shown in Figure 6.
Table 4. The number of survival analysis genes with known immune function for PD and CR in all the therapies for LUAD and COAD.
Table 5. List of putative biomarker genes related to immune response unique to each therapy for LUAD. The % of immune based putative biomarker with significant expression variance is shown.
Table 6. Putative biomarker genes related to immune response unique to each therapy for COAD. The % of immune based putative biomarker on the basis of the variance is shown.
4. Discussion
4.1. GSEA and Leading Edge Analysis
In this work, we used the public dataset from TCGA for lung and colon adenocarcinoma patients who were treated with different anticancer drugs. Due to the lack of sufficient pre-cancerous samples, this study investigated the efficacy of the treatment outcomes, which were PD and CR. Since alkylating agents were the most commonly used drug therapy for non-small cell lung cancer (NSCLC) [22] compared to other therapeutic agents, we obtained a high number of study samples for patients treated with drugs in this therapeutic category. Anti-angiogenesis therapy is relatively new in contrast to the other three therapies [23], and consists of around 20 compounds being tested – only a few of them have been FDA-approved for commercial use, which explains the relatively small sample size for this therapy class. As for colon cancer, chemotherapy (alkylating agents and antimetabolite drugs) is the preferred choice of treatment.
A geneset enrichment analysis on two datasets to evaluate pathway level differences between the two clinical responses, i.e. PD vs. CR, is presented in this study. In case of LUAD, higher enrichment for CR samples was seen as opposed to PD in all five geneset categories. Since the disease under study is cancer, the result explains the large number of genesets enriched for C6, which is an oncogenic signature category based on MsigDB as shown in Table 2. Also this pattern was consistent across the leading edge analysis, where we selected the genes that significantly contributed towards the enrichment signal of a geneset. Overall enrichment for CR was higher than PD for LUAD as shown in Figure 2.
This pattern was reversed for COAD where PD samples were more enriched than CR, as presented in Table 2, and reflects upon the chemoresistant nature of this cancer type. This result was translated further to explain the higher leading edge genes enriched for PD as compared to CR as shown in Figure 2. Moreover, oncogenic signatures were highly enriched compared to other geneset categories. However, unlike LUAD, a significantly higher enrichment percentage for immunogenic signatures (C7) was also observed, which suggests a more immuno-active behavior of COAD. This is supported by the report from Tougeron et al., [24] which described the immune response as vital factor in the progression of colon cancer, and there exists a close association between the prognosis of the disease and the rate of tumor-infiltrating lymphocytes. These results are concordant with a number of TABF-FAGO terms related to immune response function, as shown in Table 3.
4.2 TABF- FAGO term mapping
The results from Table 3 show the number of FAGO terms obtained from ClueGO analysis and mapped with TABF terms for both complete remission (CR) and progressive disease (PD). In this section we will try to comprehend these associations and their biological interpretations.
- Alkylating agents – Complete Remission
- Lung adenocarcinoma
The TABF-FAGO term mapping results for alkylating agents can be comprehended from its mechanism of action. Cytotoxicity is one of the most highly correlated topics between cancer and immune system. It is a process involved in cell toxication which trigger processes like apoptosis, necrosis, and cell lysis. Alkylating agents, as described earlier, are cytotoxic agents that target the process of cell division. The host immune system has its own set of agents that cause cytotoxicity. The main ones are lymphocytes, cytotoxicity T-cells and natural killer cells. The recent review from Kulawik [25] explains the role of cytotoxic T-lymphocytes in antitumor response in lung adenocarcinoma. The host immune system also plays a crucial role in the antitumor response with a population of CD8+ and CD4 + lymphocytes [26], neutrophils [27], NK-T, B and T cells triggering the process of apoptosis [28]. The process is often amplified with the help of macrophages and dendritic cells which contributes towards antigen presentation for a successful cytolytic attack [29]. Therefore, the TABF terms found from the literature, were categorized as: cell cytotoxicity, macrophage activation, immune response and myeloid cell activation, regulation of T cell and lymphocyte activation, and B cell proliferation and activation. These terms are known to contribute synergistically towards the efficacy of alkylating therapy and are known to show tumor remission. This explains FAGO terms related to cytotoxicity in complete remission and TABF-FAGO term mappings involved with activation of T-cell, B-cell, macrophage activation, and immune response as shown in Table 1- appendix.
Targeting some signaling pathways can prevent DNA damage response and increase the efficacy of chemotherapy. While signaling pathways are essential for normal stem cells, their dysregulation can lead to tumorigenesis [30]. They form a complex interconnected network and can be crucial in regulating the cell structure, apoptosis and macrophage polarity [31]. This explains signaling pathway related term mapping, upregulated in complete remission for alkylating agents. The process of leukocyte activation and differentiation is connected with inflammatory response, which is beneficial in normal cells, and leukocytic adhesion to endothelial tissues marks the beginning of the inflammation process. But as much as these processes are important in the normal cell functioning, they can be the reason of poor survival in cancer and their regulation is crucial for improved efficacy of chemotherapy specifically alkylating agents [32]. The negative regulation of tumor promoting leukocytes is therefore one of the most important processes in improving the efficacy of this intervention. The role of leukocytes and lymphocytes is paradoxical and their balance mediates the process of tumor regression or promotion [33].
Cytokines are secretory proteins that are involved in the regulation and activation of immune cell. The role of host-derived cytokines is insightful in understanding the cytokine-tumor cell interactions in the inhibition of cancer development. According to the review by Dranoff [34], host-derived cytokines can suppress tumor formation by controlling infection, inflammation and immunity. Our results were consistent with cytokine-associated FAGO terms upregulated in CR for alkylating therapy. The review also talks about IL-12 cytokine production by macrophages and dendritic cells inhibiting chemical carcinogenesis. We found chemical carcinogenesis pathway enriched in progressive disease while cytokine-related FAGO terms were upregulated to the opposite response (CR), which further validates and justifies our results. The host immune response can be categorized into two broad categories: innate and adaptive, where the former is the first line of defense and involves the activation of macrophages, neutrophils, granulocytes etc. while the latter activates B and T cell response and production of immunoglobulins [35]. The activation of adaptive immune response and immunoglobulin production with the process involving somatic recombination have shown to sensitize tumors to alkylating drugs and increasing the drug efficacy [36] which further explains TABF-FAGO term mapping in CR for LUAD.
b. Colon adenocarcinoma
Bracci et al., [37] discussed in-depth the relevance of host immune-competency for improved efficacy of chemotherapy. There are evidences of enhanced T and B lymphocytes in the spleen of animal model with colon cancer showing synergistic association with chemotherapy drugs like oxaliplatin, gemcitabine and 5-FU which explains the upregulation of B and T lymphocyte activation, proliferation and other terms related to the activation of immune system in CR for both alkylating agents and antimetabolite therapy. Mitochondria organization is a key player in release of cytochrome-c with subsequent activation of caspase-9 and -3 which reduces cell cycle progression and carcinogen activation, as well as promoting immunosurvelliance [38]. Furthermore, non-coding RNA processing is often associated with improved response to chemotherapy in colon cancer and are directly involved in DNA methylation, histone modification and gene silencing. In turn, they are epigenetically targeted for repression of cancer and improved drug therapy [39]. All these biological processes explain the upregulation of FAGO terms in Table 2- appendix.
4.2.2 Alkylating Agents – Progressive Disease
- a) Lung adenocarcinoma
We obtained 18 FAGO terms that were successfully mapped with the TABF terms as shown in Table 3-appendix. Holland-Feir Cancer Medicine (6th ed.) [40] presents a complete review on alkylating agents and the factors affecting its efficacy. Alkylating agents induce cell cytotoxicity but its potency is determined by the host system. One such finding is the host response to alkylation damage by arresting the cell in the G2 phase of the cell cycle which in turn activates the DNA repair process, where cells enter the mitotic phase despite alkylation damage, which leads to therapy resistance. A human tumor cell line has been shown to exhibit G2 arrest in response to alkylating damage, resulting in resistance to nitrogen mustard [41] which clearly explains the upregulation of FAGO term: regulation of cell cycle G1/S phase transition in PD for lung cancer. In some cases, transcription factors involved in DNA transcription initiation are released in response to genotoxic stress (agents involved in DNA damage), which stimulate RNA polymerase and promote RNA binding. This eventually leads to mRNA synthesis and the process is called gene activation, further stimulating DNA repair mechanisms by the host system and promoting defense against alkylating agents [42]. We found related FAGO terms upregulated in PD as shown in Table 3- appendix, which strongly correlates with the findings discussed above.
The relationship between inflammatory response and cancer progression was first discovered in the 19th century by Rudolf Virchow and is one of the most widely studied phenomena. There exists an established connection between leukocyte chemotaxis and inflammation. The movement of leukocytes from blood to tissue in response to inflammatory stimuli was observed by Metchnikoff in 1891. During inflammation, leukocytes migrate to the site of inflammation to fulfill their role in defense response [43]. Pathways involved in inflammation and wound healing have shown to be associated with promoting tumorigenesis, with increased resistance to anticancer treatments. Interestingly, there also exists a strong link between complement activation and inflammation. Generally, the complement cascade system is activated to defend a host against microbes during an inflammatory response. The activation of complement and its role in tumor progression is evident in studies conducted for different cancer types, including lung adenocarcinoma [44], breast [45] and thyroid cancer [46]. Consequently, we observed similar processes from ClueGO results where genes involved in inflammation, complement activation and defense response were activated in PD alongside genes responsible for positive regulation of leukocyte chemotaxis.
- b) Colon adenocarcinoma
Colon cancer is strongly linked with inflammatory microenvironment composed of intestinal epithelial cells, immune cells, etc. which determine the fate of the intervention. Moreover, cytokines acts as a mediator between the host immune response and malignant cells that activate oncogenic transcription factor STAT3 which is a major activator in cancer inflammation and promotes cell cycle progression [47]. Holohan et al., explains the role of JAK-STAT signaling pathway in cell proliferation, tumor progression, and chemo-resistance [48]. We found the upregulation of several interleukin factors, e.g. IL-1, 2, 4, 6, and 10, in progressive disease for colon cancer. IL-6 is a major player in inflammatory response in COAD that interacts with the membrane-associated gp130 subunit and activates Janus kinases (JAKs) with downstream effects on STAT3 [49], which explains their role in tumor progression as shown in Table 4-appendix.
IL-6 can increase resistance to various chemotherapeutic drugs [50, 51] by preventing apoptosis and downstream signaling of MAPK cascade [52]. Moreover, the release angiogenesis growth factors greatly influence the drug response in solid tumors and evidence suggests their involvement in cancer cell survival in the presence of chemo drugs [53]. Furthermore, IL-1b is a pro-inflammatory cytokine which can modulate the response of different drug transports [54]. Tumor necrosis factor is a major inflammatory cytokine and is well known for its role in tumor progression and drug resistance. Therefore, the upregulation of this term in the PD category for alkylating agents is well explained [55]. Mccubre et al., discusses the effect of the ERK-signaling cascade in promoting tumorigenesis and resistance to doxorubicin treatment [56]. All these points support our results from TABF-FAGO term mapping for PD in alkylating agents in Table 4-appendix [57].
4.2.3 Antimetabolite – Complete Remission
- a) Lung adenocarcinoma
Antimetabolites are another class of chemotherapeutic drug that inhibit the action of metabolites and interfere with DNA and RNA growth [58]. They replace the normal compounds in the cell, resulting in disrupted cell division and specifically targeting the synthetic phase of cell cycle [59]. Since antimetabolites disrupt cell division and DNA damage, the TABF and FAGO terms upregulated for complete remission were very similar to alkylating agents. We found FAGO terms related to cell cytotoxicity upregulated in CR, and the majority of the FAGO terms were similar to alkylating agents.
Naïve CD4+ T cells can be categorized into four main lineage: T helper cells -1, 2, 17 and Treg cells [60]. Th2 promotes tumor growth and Th1 produce cytokines like interferon gamma, which causes cytotoxic T lymphocytes (CTL) proliferation, resulting in tumor elimination and potentiating the action of chemotherapy [61].The host immune milieu is rich in cytokine and chemokines which can produce immune-stimulatory effects. For instance, type-I IFNs can enhance the stimulation of dendritic cells which activate Th1 and inhibits Treg activity [62]. Therefore, we saw FAGO terms associated with Th cell activation and cytokines in Table 5-appendix.
Signaling pathways are popular targets and critical to the success of an anticancer therapy [63]. For instance, activated PI3K/Akt/mTOR pathways can regulate cell proliferation, growth, and survival, and are important targets for the development of potential antitumor agents [64]. According to Wang et al [65], the activation of signal transduction pathways enhances the cellular response to drug treatment. Hence, we found various signaling pathways terms associated with CR.
The link between skeletal system development and the immune system has been recognized recently with an interdisciplinary field studying and embracing the relationship under the name of “osteoimmunology”. Studies have also shown that osteoimmunology can be a potential target for anticancer interventions as several systems that are related to host immune response are actually related to skeletal function. Such information has been useful to understand the improved efficacy of anticancer drugs, especially antimetabolites [66]. Receptor activator of NF-kB (RANK)/RANK Ligand (RANKL)/Osteoprotegerin (OPG) system is involved in the regulation of osteoclastogenesis, which influences immune, cardiovascular, endocrine, and nervous systems. They stimulate the antigen-specific immune response and are involved in dendritic cell maturation and survival [67, 68]. Hence, this clearly explains the upregulation of TABF-FAGO association in Table 5-appendix.
- b) Colon Adenocarcinoma
We observed the upregulation of terms related to T cell lymphocyte activation, proliferation and positive regulation of immune response, a consistent pattern for CR category in all chemotherapies for both lung and colon cancer. Additionally, we found histone modification terms enriched in CR. Histone modifications are crucial to gene regulation processes by compacting DNA. Histones are often involved in maintaining the balance between euchromatin and heterochromatin by undergoing post-translational modification at the amino terminal ends. These modifications, e.g. methylation, acetylation, ubiquitination, etc. [69] are correlated with the efficacy of chemo drugs in numerous types of cancer (prostate, breast, colorectal, lung) and hematological malignancies. All these TABF terms with their mapped FAGO terms are presented in Table 6-appendix.
4.2.4 Antimetabolites – Progressive Disease
- a) Lung adenocarcinoma
Almost 90% of genomic DNA is transcribed into RNA, but only 2% of this RNA is protein-coding; the remaining “dark matter” genomic content is transcribed into non-coding RNA (ncRNA) and has been implicated with biological significance particularly in cancer. According to Liu et al. [70], ncRNAs play a crucial role in tumor progression and resistance to chemotherapy. Expression of metastasis-associated lung adenocarcinoma transcript 1 (MALAT1), a long non-coding RNA (lncRNA) is associated with resistance to chemotherapy and poor prognosis in patients in non-small cell lung cancer [71]. Another interesting study was conducted by Mader and colleagues [72] that showed increased expression of thymidylate synthase (TS) resulted in resistance to antimetabolite drug –5 fluorouracil (5-FU), to incorporate with RNA. Our results for progressive disease were in consensus with the discussion as shown in Table 7 -appendix.
- b) Colon adenocarcinoma
The upregulated terms in the PD category for antimetabolite therapies were similar to alkylating agents – IL1 production, tumor necrosis factor production, inflammation, and ERK cascade. This is explained by their both being chemotherapy drugs with similar modes of action. Slow growth of tumors or G0-G1 cell cycle promotes resistance against antimetabolite drugs, which explains the connection between the upregulation of G1/s , G2/M phase transition and the progression of disease in Table 8 -appendix. Furthermore, literature based study shows TNF-alpha increase post-drug recovery and tumor survival in colon cancer from 5-FU (antimetabolite drug) by obstructing S phase entry [73].
- Mitotic Inhibitors – Complete remission
Mitotic inhibitors are derived from natural plant alkaloids. They disrupt microtubules involved in mitotic cell division process [74]. Even though the mechanism of action for this therapy is very similar to alkylating agents, it was interesting to see that the immune response varied with some unique FAGO –TABF term mapping. The terms that were similar to alkylating agents were: T cell and lymphocyte activation, B cell activation, positive regulation of immune response and cell signaling. The terms similar to antimetabolite therapies were related to system development and KEGG signaling pathways. Interestingly, we found some striking differences. Firstly, innate immune response was more active as compared to adaptive when compared to alkylating agents and antimetabolites. Secondly, we found significant upregulation of FAGO terms related to cyclic adenosine monophosphate (cAMP) processes, which is attributed to the fact that cAMP plays a fundamental role in cellular response. Regulation of cAMP pathways is associated with ion metabolism and apoptosis [75]. They are also known to intermingle with other signaling pathways like calcium [76], JAK/STAT inhibitors [77], and RAK-mediated MAPK kinases to modulate cell processes [78]. The upregulation of cAMP-related pathways is favorable for the action of antimitotic drugs [79] which justifies the upregulated FAGO terms in CR as shown in Table 9 -appendix.
Another paradoxical phenomenon that was observed in antimitotic agents was the upregulation of an inflammatory response in CR unlike the other therapies. Even though inflammatory response is often associated with tumor regression, in some cases it can enhance the action of antimitotic agents. Host macrophages secreting inflammatory cytokines have been shown to induce the expression of inducible nitric oxide synthase (iNOS). This potentiated the effect of paclitaxel to regress cancer in solid tumors and promoted apoptosis [80]. Since inflammatory response is a critical stage in the wound healing process [81], we could see that wound healing was upregulated alongside inflammatory response in CR as shown in Table 9 –appendix.
Another contradictory observation was upregulation of interleukin-10 (IL-10) which is an anti-inflammatory cytokine that downregulates cytokine production. The effect of host IL-10 in tumor progression or regression has been debated over a long time. While studies have shown that regulatory T cells (Treg) produce IL-10 which downregulates immune response [82], this is inconsistent with Mumm et al’s [83] claim that IL-10 reduces tumor development by inducing cytolytic molecules in CD8+ T cells and IFN- gamma dependent mechanisms. The review by Teng et al. [84] talks about the significance of stable IL-10, which can be beneficial in the regressing tumor. So far, there has not been any strong evidence showing a positive correlation between host IL-10 expression and survival of cancer patients. Our results in Table 9 -appendix highlights the above discussion.
4.2.6 Mitotic Inhibitors – Progressive Disease
The key highlight in the PD category was the upregulation of terms: antigen presentation and transporter-associated with antigen processing (TAP), which are known for their multidrug resistance in cancer. The experiment conducted on a human cancer cell line by Izquierdol and colleagues [85] present a strong correlation between TAP expression and resistance (~ 2 fold) to the antimitotic drugs doxorubicin and vincristine. Mitotic checkpoints and response to DNA damage have shown resistance against these drugs, as they sense microtubule attachment to kinetochore. According to Wang et al. [65], the activation or dysregulation of these mitotic checkpoints and DNA damage response leads to continued cell division and increased resistance to taxol (antimitotic drug). Table 10 -appendix shows the FAGO terms upregulated in PD for mitotic inhibitors.
4.2.7 Anti-Angiogenesis
Angiogenesis is the formation of new blood vessels and is known to play an essential role in tumor growth, as blood vessels supply oxygen and nutrients for the cancerous cell’s nourishment. Angiogenesis inhibitors bind to the vascular endothelial growth factor (VEGF) and prevent them from binding to the receptor in order to facilitate the process of vasculogenesis [86].
- a) Complete Remission
The mechanism of action for this therapy is different from the other three types of chemotherapies discussed previously. There is a strong link between host immune response and anti-angiogenesis therapy. T-cell activation and VEGF share an antagonistic relationship where the latter inhibits the production of T cells by interfering with thymus development [87]. Therefore, T-cell activation has a positive effect on anti-angiogenesis therapy. To back up this hypothesis, we have the case study of semaphorins, axon guidance molecules belonging to the class of transmembrane proteins which are known to play a role in immune response and organ morphogenesis. Class IV semophorin (Sem4A), expressed on dendritic and B-cells, enhance T-cell activation and differentiation [88]. Toyofoku and colleagues demonstrated in their experiments that mice lacking Sem4A exhibit enhanced angiogenesis in response to VEGF or inflammatory stimuli [89]. Therefore, deeming Sem4A as an inhibitor of VEGF and angiogenesis. Our results are concurrent with the above discussion and presented in Table 11- appendix.
Hypoxia is a process of oxygen depletion in cells which induce pro-angiogenic factors along with inflammatory response. According the study conducted by Brustugun [90], hypoxia caused treatment failure in lung carcinoma. Resistance to gefitinib, extensively used in EGFR‐mutated lung cancer, is also induced by hypoxia via upregulation of growth factor. Blocking chronic inflammation can prevent pro-tumor polarization and contribute to anti-angiogenesis effect. Therefore, we see the process of negative regulation of hypoxia upregulated in CR. Even though the wound healing process is connected with an inflammatory response, in the case of anti-angiogenesis therapy response wounding is seen to contribute to increased efficacy. Activated host healing response is a crucial process after the extensive surgery of solid tumors. Interleukin-6 (IL-6) can act as an inflammatory cytokine and also as an anti-inflammatory cytokine [91]. There are other cytokines (IL-20) that possess anti-angiogenesis properties [92] and their regulation enhances the efficacy of the therapy as reflected in Table 11- appendix.
- b) Progressive Disease
We observed several immune-related terms upregulated in progressive disease for anti-angiogenesis therapy. We discussed the role of VEGF and other growth factors in promoting angiogenesis, which results in tumor progression. The results in Table 12 from appendix section show the upregulation of all these pathways which induce therapy resistance. MHC and antigen presentation are associated with a multidrug resistance class of proteins especially in lung cancer; the overexpression of the transporter protein is known to induce high resistance to therapy [93]. But further investigation is needed to understand their involvement with cancer progression and resistance. The review conducted by Zaidi and Merlino [94] talks about the paradoxical role of interferon gamma in producing immune response against tumors while also producing tumorigenic effects under certain conditions. Hence, response to interferon gamma has a role in reducing drug efficacy and promoting tumor progression. Innate immunity can release angiogenic factors and promote tumor angiogenesis. Therefore, the release of inflammatory cytokines by the host innate immune system can further diminish the response to drug therapy [95]. The activated DNA repair mechanism has shown resistance to cetuximab/Erbitux (an anti-angiogenesis drug) used to treat EGFR-expressing tumors [96]. Table 12 in appendix shows upregulated FAGO terms mapped with all the biological functions discussed above.
4.3 Survival analysis and Biomarkers
The prognostic ability of genes specific to each therapy were tested using survival analysis. We found several genes with significant overall survival for both LUAD and COAD as shown in Table 4, which further validates the role of host immune response and therapy outcome. As for the common genes across different therapies, there was no complete overlap of genes for LUAD in PD; this result is obvious, as all four therapies have different modes of action. But, interestingly, there was an overlap of 120 genes for CR, shown in Figure 3, with one gene (IL4R- interleukin 4 receptor) that showed significant expression variance and survival for lung adenocarcinoma. The role of IL4R has been paradoxical in cancer immunology and has often been debated. In our results, IL4 was seen to be involved with complete remission when treated with any of the three therapies: mitotic inhibitors, anti-angiogenesis and antimetabolite. This was validated further with the experimental evidence of overexpressed IL4R contributing towards remission in lung cancer patients [97].
However, in colon cancer, the two treatments under study have similar cell cycle targets. Hence, we found more overlapping genes between alkylating agents and antimetabolites as shown in Figure 3. While we found only 4 genes (FAM3C, CRLF3, CETP, VAV3) that showed significant survival and expression variance as shown in Figure 6, the first three showed higher expression for disease progression, while VAV3 was seen as CR upregulated. We also found literature evidence of VAV3 as one of the molecular signatures for colon cancer [98]. IL-17 is often associated with tumor promotion and acts as a pro-angiogenesis factor, while in some cases it has also been regarded as tumor suppressing, as shown by experimental studies from Kryczek et al. [99]. Therefore, its role has remained undefined. However, in our results, we found IL-17 to contribute towards complete remission in antimetabolite therapy for colon cancer.
5. Conclusion
The era of translational medicine has opened new horizons for personalized therapeutic interventions and spurred the development of novel prognostic therapy-based biomarkers for each patient’s individual immune profile. It is now evident that the tumor–host immune interaction dictates the magnitude, quality and efficacy of most anticancer strategies. Despite this, in our knowledge, no systematic analysis has been performed to study expression and pathway-based differences of multiple anticancer therapies depending on patient’s immunocompetence.
In this study, we used expression based analysis to come up with biomarkers with prognostic validity for different anticancer therapies in two cancer datasets, LUAD and COAD. We found many gene biomarkers, unique to a cancer type or therapy, which were either associated with the progression or remission of the intervention. Additionally, we also found a number of overlapping genes across different therapies, of which some had a controversial status towards cancer promotion or regression. Our results predicted the outcome of therapy resistance alongside its improved efficacy to some extent. The scope of this study went further to understand some of the pathways and their mode of mechanism in-depth for different therapies in both cancer types. However, one major limitation of this study is the absence of experimental validation for these biomarkers even though the majority of the indicated genes in our results have corresponding correlations within the literature. Therefore, such information would be highly valuable for translational medicine and in making an accurate intervention choice.
This work is an extension of our previous conference paper published at BIBM IEEE 2016 [100].
Conflict of Interest
The authors declare no conflict of interest.
- S. B. Coffelt, K. E. de Visser, “Immune-mediated mechanisms influencing the efficacy of anticancer therapies” Trends in immunology., 36(4), 198–216, 2015.
- G. P. Dunn, L. J. Old, R. D. Schreiber, “The immunobiology of cancer immunosurveillance and immunoediting” Immunity., 21(2), 137–148, 2004.
- K. N. Kodumudi, K. Woan, D. L. Gilvary, E. Sahakian, S. Wei, J. Y. Djeu, “A novel chemoimmunomodulating property of docetaxel: suppression of myeloid-derived suppressor cells in tumor bearers” Clinical Cancer Research., 16(18), 4583–4594, 2010.
- E. M. Dijkgraaf, M. Heusinkveld, B. Tummers, L. T.C. Vogelpoel, R. Goedemans, V. Jha, J. W.R. Nortier, M. J.P. Welters, J. R. Kroep, S. H. van der Burg, “Chemotherapy alters monocyte differentiation to favor generation of cancer-supporting M2 macrophages in the tumor microenvironment” Cancer research., 73(8), 2480–2492, 2013.
- P. A. Savage, S. Malchow, D. S. Leventhal, “Basic principles of tumor-associated regulatory T cell biology” Trends in immunology., 34(1), 33–40, 2013.
- A.-P. Ganesan, M. Johansson, B. Ruffell, A. Yagui-Beltrán, J. Lau, D. M. Jablons, L. M. Coussens, “Correction: Tumor-infiltrating regulatory T cells inhibit endogenous cytotoxic T cell responses to lung adenocarcinoma” The Journal of Immunology., 191(10), 5319, 2013.
- C. Murdoch, M. Muthana, S. B. Coffelt, C. E. Lewis, “The role of myeloid cells in the promotion of tumour angiogenesis” Nature Reviews Cancer., 8(8), 618–631, 2008.
- R. Mazzieri, F. Pucci, D. Moi, E. Zonari, A. Ranghetti, A. Berti, L. S. Politi, B. Gentner, J. L. Brown, L. Naldini, “Targeting the ANG2/TIE2 axis inhibits tumor growth and metastasis by impairing angiogenesis and disabling rebounds of proangiogenic myeloid cells” Cancer cell., 19(4), 512–526, 2011.
- D. M. Parkin, F. Bray, J. Ferlay, P. Pisani, “Global cancer statistics, 2002” CA: a cancer journal for clinicians., 55(2), 74–108, 2005.
- S. Colak, J. P. Medema, “Cancer stem cells–important players in tumor therapy resistance” FEBS journal., 281(21), 4779–4791, 2014.
- K. L. Manasa, “E7010: Investigational anticancer agents targeting the microtubules” International Journal of Pharmaceutical Sciences and Research., 6(9), 3713, 2015.
- J. Folkman, “Clinical applications of research on angiogenesis” New England Journal of Medicine., 333(26), 1757–1763, 1995.
- M. F. Misman, S. Deris, S. Z. M. Hashim, R. Jumali, M. S. Mohamad, “Pathway-based microarray analysis for defining statistical significant phenotype-related pathways: a review of common approaches” in 2009 International Conference on Information Management and Engineering, Kuala Lumpur, Malaysia, 2009.
- A. Subramanian, P. Tamayo, V. K. Mootha, S. Mukherjee, B. L. Ebert, M. A. Gillette, A. Paulovich, S. L. Pomeroy, T. R. Golub, E. S. Lander, “Gene set enrichment analysis: a knowledge-based approach for interpreting genome-wide expression profiles” Proceedings of the National Academy of Sciences., 102(43), 15545–15550, 2005.
- A. Liberzon, A. Subramanian, R. Pinchback, H. Thorvaldsdóttir, P. Tamayo, J. P. Mesirov, “Molecular signatures database (MSigDB) 3.0” Bioinformatics., 27(12), 1739–1740, 2011.
- C. Kin, E. Kidess, G. A. Poultsides, B. C. Visser, S. S. Jeffrey, “Colorectal cancer diagnostics: biomarkers, cell-free DNA, circulating tumor cells and defining heterogeneous populations by single-cell analysis” Expert review of molecular diagnostics., 13(6), 581–599, 2013.
- G. Bindea, B. Mlecnik, H. Hackl, P. Charoentong, M. Tosolini, A. Kirilovsky, W.-H. Fridman, F. Pagès, Z. Trajanoski, J. Galon, “ClueGO: a Cytoscape plug-in to decipher functionally grouped gene ontology and pathway annotation networks” Bioinformatics., 25(8), 1091–1093, 2009.
- H. Xiong, Z.-G. Zhang, X.-Q. Tian, D.-F. Sun, Q.-C. Liang, Y.-J. Zhang, R. Lu, Y.-X. Chen, J.-Y. Fang, “Inhibition of JAK1, 2/STAT3 signaling induces apoptosis, cell cycle arrest, and reduces tumor cell invasion in colorectal cancer cells” Neoplasia., 10(3), 287–297, 2008.
- H. Mizuno, K. Kitada, K. Nakai, A. Sarai, “PrognoScan” BMC medical genomics., 2(1), 18, 2009.
- D. R. Cox, D. Oakes, “Analysis of survival data, CRC Press, 1984.
- S. Bhattacharya, S. Andorf, L. Gomes, P. Dunn, H. Schaefer, J. Pontius, P. Berger, V. Desborough, T. Smith, J. Campbell, “ImmPort: disseminating data to the public for the future of immunology” Immunologic research., 58(2-3), 234–239, 2014.
- R. J. Scheff, B. J. Schneider, “Non–Small-Cell Lung Cancer: Treatment of Late Stage Disease: Chemotherapeutics and New Frontiers” in Seminar Interventional Radiology., 30(02), 191–198, Thieme Medical Publishers, 2013.
- T. E. Stinchcombe, “Targeted therapy of advanced non-small cell lung cancer: the role of bevacizumab” Biologics: targets & therapy., 1(3), 185, 2007.
- D. Tougeron, É. Fauquembergue, J.-B. Latouche, “Réponse immunitaire et cancers colorectaux” Bulletin du Cancer., 100(3), 283–294, 2013.
- J. Domagala-Kulawik, “The role of the immune system in non-small cell lung carcinoma and potential for therapeutic intervention” Translational lung cancer research., 4(2), 177–190, 2015.
- M. Salagianni, C. N. Baxevanis, M. Papamichail, S. A. Perez, “New insights into the role of NK cells in cancer immunotherapy” Oncoimmunology., 1(2), 205–207, 2012.
- Z. Granot, J. Jablonska, “Distinct functions of neutrophil in cancer and its regulation” Mediators of inflammation., 2015, 2015.
- J. G. Aerts, J. P. Hegmans, “Tumor-specific cytotoxic T cells are crucial for efficacy of immunomodulatory antibodies in patients with lung cancer” Cancer research., 73(8), 2381–2388, 2013.
- K. Vermaelen, R. Pauwels, “Pulmonary dendritic cells” American journal of respiratory and critical care medicine., 172(5), 530–551, 2005.
- G. Leon, L. MacDonagh, S. P. Finn, S. Cuffe, M. P. Barr, “Cancer stem cells in drug resistant lung cancer:Targeting cell surface markers and signaling pathways” Pharmacology & therapeutics., 158), 71–90, 2016.
- G. S. Martin, “Cell signaling and cancer” Cancer cell., 4(3), 167–174, 2003.
- D. G. DeNardo, D. J. Brennan, E. Rexhepaj, B. Ruffell, S. L. Shiao, S. F. Madden, W. M. Gallagher, N. Wadhwani, S. D. Keil, S. A. Junaid, “Leukocyte complexity predicts breast cancer survival and functionally regulates response to chemotherapy” Cancer discovery., 1(1), 54–67, 2011.
- S. Ostrand-Rosenberg, “Immune surveillance: a balance between protumor and antitumor immunity” Current opinion in genetics & development., 18(1), 11–18, 2008.
- G. Dranoff, “Cytokines in cancer pathogenesis and cancer therapy” Nature Reviews Cancer., 4(1), 11–22, 2004.
- L. M. Weiner, R. Surana, S. Wang, “Monoclonal antibodies: versatile platforms for cancer immunotherapy” Nature Reviews Immunology., 10(5), 317–327, 2010.
- G. P. Adams, L. M. Weiner, “Monoclonal antibody therapy of cancer” Nature biotechnology., 23(9), 1147–1157, 2005.
- L. Bracci, G. Schiavoni, A. Sistigu, F. Belardelli, “Immune-based mechanisms of cytotoxic chemotherapy: implications for the design of novel and rationale-based combined treatments against cancer” Cell Death & Differentiation., 21(1), 15–25, 2014.
- L. M. Coussens, Z. Werb, “Inflammation and cancer” Nature., 420(6917), 860–867, 2002.
- C. Nervi, E. de Marinis, G. Codacci-Pisanelli, “Epigenetic treatment of solid tumours: a review of clinical trials” Clinical epigenetics., 7(1), 127, 2015.
- D. W. Kufe, J. F. Holland, “Cancer medicine 6, Decker, 2003.
- P. M. O’Connor, D. K. Ferris, G. A. White, J. Pines, T. Hunter, D. L. Longo, K. W. Kohn, “Relationships between cdc2 kinase, DNA cross-linking, and cell cycle perturbations induced by nitrogen mustard” Cell Growth Differ., 3(1), 43–52, 1992.
- M. Christmann, B. Kaina, “Transcriptional regulation of human DNA repair genes following genotoxic stress: trigger mechanisms, inducible responses and genotoxic adaptation” Nucleic acids research., 41(18), 8403–8420, 2013.
- A. Rot, “The role of leukocyte chemotaxis in inflammation, Springer Netherlands, 1992.
- D. Ajona, C. Razquin, M. D. Pastor, M. J. Pajares, J. Garcia, F. Cardenal, M. Fleischhacker, M. D. Lozano, J. J. Zulueta, B. Schmidt, “Elevated levels of the complement activation product C4d in bronchial fluids for the diagnosis of lung cancer” PloS one., 10(3), e0119878, 2015.
- F. Niculescu, H. G. Rus, M. Retegan, R. Vlaicu, “Persistent complement activation on tumor cells in breast cancer” The American journal of pathology., 140(5), 1039, 1992.
- M. Yamakawa, K. Yamada, T. Tsuge, H. Ohrui, T. Ogata, M. Dobashi, Y. Imai, “Protection of thyroid cancer cells by complement-regulatory factors” Cancer., 73(11), 2808–2817, 1994.
- J. Terzić, S. Grivennikov, E. Karin, M. Karin, “Inflammation and colon cancer” Gastroenterology., 138(6), 2101–2114, 2010.
- C. Holohan, S. van Schaeybroeck, D. B. Longley, P. G. Johnston, “Cancer drug resistance: an evolving paradigm” Nature Reviews Cancer., 13(10), 714–726, 2013.
- R. Catlett-Falcone, W. S. Dalton, R. Jove, “STAT proteins as novel targets for cancer therapy” Current opinion in oncology., 11(6), 490, 1999.
- D. R. Hodge, E. M. Hurt, W. L. Farrar, “The role of IL-6 and STAT3 in inflammation and cancer” European journal of cancer., 41(16), 2502–2512, 2005.
- A. Lichtenstein, Y. Tu, C. Fady, R. Vescio, J. Berenson, “Interleukin-6 inhibits apoptosis of malignant plasma cells” Cellular immunology., 162(2), 248–255, 1995.
- A. Ogata, D. Chauhan, G. Teoh, S. P. Treon, M. Urashima, R. L. Schlossman, K. C. Anderson, “IL-6 triggers cell growth via the Ras-dependent mitogen-activated protein kinase cascade” The Journal of Immunology., 159(5), 2212–2221, 1997.
- P. M. Navolanic, L. S. Steelman, J. A. McCubrey, “EGFR family signaling and its association with breast cancer development and resistance to chemotherapy (Review)” International journal of oncology., 22(2), 237–252, 2003.
- F. Mosaffa, F. Kalalinia, H. Lage, J. T. Afshari, J. Behravan, “Pro-inflammatory cytokines interleukin-1 beta, interleukin 6, and tumor necrosis factor-alpha alter the expression and function of ABCG2 in cervix and gastric cancer cells” Molecular and cellular biochemistry., 363(1-2), 385–393, 2012.
- F. Balkwill, “Tumour necrosis factor and cancer” Nature Reviews Cancer., 9(5), 361–371, 2009.
- J. A. McCubrey, L. S. Steelman, W. H. Chappell, S. L. Abrams, E. W.T. Wong, F. Chang, B. Lehmann, D. M. Terrian, M. Milella, A. Tafuri, “Roles of the Raf/MEK/ERK pathway in cell growth, malignant transformation and drug resistance” Biochimica et Biophysica Acta (BBA)-Molecular Cell Research., 1773(8), 1263–1284, 2007.
- P. J. Morin, “Drug resistance and the microenvironment” Drug Resistance Updates., 6(4), 169–172, 2003.
- G. J. Peters, C. L. van der Wilt, C. J. van Moorsel, Kroep, JR, Am Bergman, S. P. Ackland, “Basis for effective combination cancer chemotherapy with antimetabolites” Pharmacology & therapeutics., 87(2), 227–253, 2000.
- P. D. Cole, J. A. Zebala, B. A. Kamen, “Antimetabolites” Drug Discovery Today: Therapeutic Strategies., 2(4), 337–342, 2006.
- J. Zhu, H. Yamane, W. E. Paul, “Differentiation of effector CD4 T cell populations” Annual review of immunology., 28), 445–489, 2009.
- Z.-C. Ding, G. Zhou, “Cytotoxic chemotherapy and CD4” Clinical and Developmental Immunology., 2012, 2012.
- A. Le Bon, D. F. Tough, “Links between innate and adaptive immunity via type I interferon” Current opinion in immunology., 14(4), 432–436, 2002.
- G. Powis, “Signalling pathways as target for anticancer drug development” Pharmacology & therapeutics., 62(1-2), 57–95, 1994.
- V. Asati, D. K. Mahapatra, S. K. Bharti, “PI3K/Akt/mTOR and Ras/Raf/MEK/ERK signaling pathways inhibitors as anticancer agents” European journal of medicinal chemistry., 109), 314–341, 2016.
- X. Wang, H. W. Cheung, A. C. Chun, D.-Y. Jin, Y.-C. Wong, “Mitotic checkpoint defects in human cancers and their implications to chemotherapy” Frontiers in bioscience: a journal and virtual library., 13), 2103–2114, 2007.
- C. Criscitiello, G. Viale, L. Gelao, A. Esposito, M. de Laurentiis, S. de Placido, M. Santangelo, A. Goldhirsch, G. Curigliano, “Crosstalk between bone niche and immune system: osteoimmunology signaling as a potential target for cancer treatment” Cancer treatment reviews., 41(2), 61–68, 2015.
- M. L. Cheng, L. Fong, “Effects of RANKL-targeted therapy in immunity and cancer” Frontiers in oncology., 3), 329, 2014.
- S. H. Lee, Y. Choi, “Communication between the skeletal and immune systems” Osteoporosis and Sarcopenia., 1(2), 81–91, 2015.
- T. Kouzarides, “Chromatin modifications and their function” Cell., 128(4), 693–705, 2007.
- Q. Liu, J. Huang, N. Zhou, Z. Zhang, A. Zhang, Z. Lu, F. Wu, Y.-Y. Mo, “LncRNA loc285194 is a p53-regulated tumor suppressor” Nucleic acids research., 41(9), 4976–4987, 2013.
- Q. Ji, X. Liu, X. Fu, L. Zhang, H. Sui, L. Zhou, J. Sun, J. Cai, J. Qin, J. Ren, “Resveratrol inhibits invasion and metastasis of colorectal cancer cells via MALAT1 mediated Wnt/β-catenin signal pathway” PloS one., 8(11), e78700, 2013.
- R. M. Mader, M. Müller, G. G. Steger, “Resistance to 5-fluorouracil” General Pharmacology: The Vascular System., 31(5), 661–666, 1998.
- L.-C. Wang, C. Y. Okitsu, E. Zandi, “Tumor necrosis factor α-dependent drug resistance to purine and pyrimidine analogues in human colon tumor cells mediated through IKK” Journal of Biological Chemistry., 280(9), 7634–7644, 2005.
- K. S. Chan, C. G. Koh, H. Y. Li, “Mitosis-targeted anti-cancer therapies” Cell death & disease., 3(10), e411, 2012.
- B. Y. EARL, W. SUTHERLAND, T. W. RALL, “Fractionation and characterization of a cyclic adenine ribonucleotide formed by tissue particles, 1958.
- P. J. Rogue, J.-P. Humbert, A. Meyer, S. Freyermuth, M.-M. Krady, A. N. Malviya, “cAMP-dependent protein kinase phosphorylates and activates nuclear Ca2+-ATPase” Proceedings of the National Academy of Sciences., 95(16), 9178–9183, 1998.
- M. David, E. Petricoin, A. C. Larner, “Activation of protein kinase A inhibits interferon induction of the Jak/Stat pathway in U266 cells” Journal of Biological Chemistry., 271(9), 4585–4588, 1996.
- S. J. Cook, F. McCormick, “Inhibition by cAMP of Ras-dependent activation of Raf” SCIENCE-NEW YORK THEN WASHINGTON-., 262), 1069, 1993.
- E. K. Rowinsky, “Signal events” The Oncologist., 8(Supplement 3), 5–17, 2003.
- T. J. Mitchison, “The proliferation rate paradox in antimitotic chemotherapy” Molecular biology of the cell., 23(1), 1–6, 2012.
- S. a. Guo, L. A. DiPietro, “Factors affecting wound healing” Journal of dental research., 89(3), 219–229, 2010.
- O. Martinez-Maza, J. S. Berek, “Cancer Immunology” The Global Library of Women’s Medicine, 2009.
- J. B. Mumm, J. Emmerich, X. Zhang, I. Chan, L. Wu, S. Mauze, S. Blaisdell, B. Basham, J. Dai, J. Grein, “IL-10 elicits IFN-dependent tumor immune surveillance” Cancer cell., 20(6), 781–796, 2011.
- M. W.L. Teng, P. K. Darcy, M. J. Smyth, “Stable IL-10: a new therapeutic that promotes tumor immunity” Cancer cell., 20(6), 691–693, 2011.
- M. A. Izquierdo, J. J. Neefjes, A. E. Mathari, M. J. Flens, G. L. Scheffer, R. J. Scheper, “Overexpression of the ABC transporter TAP in multidrug-resistant human cancer cell lines” British journal of cancer., 74(12), 1961, 1996.
- S. Rafii, D. Lyden, R. Benezra, K. Hattori, B. Heissig, “Vascular and haematopoietic stem cells: novel targets for anti-angiogenesis therapy?” Nature Reviews Cancer., 2(11), 826–835, 2002.
- J. E. Ohm, D. I. Gabrilovich, G. D. Sempowski, E. Kisseleva, K. S. Parman, S. Nadaf, D. P. Carbone, “VEGF inhibits T-cell development and may contribute to tumor-induced immune suppression” Blood., 101(12), 4878–4886, 2003.
- A. Kumanogoh, S. Marukawa, K. Suzuki, N. Takegahara, C. Watanabe, E. Ch’ng, I. Ishida, H. Fujimura, S. Sakoda, K. Yoshida, “Class IV semaphorin Sema4A enhances T-cell activation and interacts with Tim-2” Nature., 419(6907), 629–633, 2002.
- T. Toyofuku, M. Yabuki, J. Kamei, M. Kamei, N. Makino, A. Kumanogoh, M. Hori, “Semaphorin-4A, an activator for T-cell Imediated immunity, suppresses angiogenesis via Plexin-D1” The EMBO journal., 26(5), 1373–1384, 2007.
- O. T. Brustugun, “Hypoxia as a cause of treatment failure in non-small cell carcinoma of the lung” Seminars in radiation oncology, 2014.
- S. A. Jones, J. Scheller, S. Rose-John, “Therapeutic strategies for the clinical blockade of IL-6/gp130 signaling” The Journal of clinical investigation., 121(9), 3375–3383, 2011.
- N. Heuzé-Vourc’h, M. Liu, H. Dalwadi, F. E. Baratelli, L. Zhu, L. Goodglick, M. Põld, S. Sharma, R. D. Ramirez, J. W. Shay, “IL-20, an anti-angiogenic cytokine that inhibits COX-2 expression” Biochemical and biophysical research communications., 333(2), 470–475, 2005.
- S. P. Cole, G. Bhardwaj, J. H. Gerlach, J. E. Mackie, C. E. Grant, K. C. Almquist, A. J. Stewart, E. U. Kurz, A. Duncan, R. G. Deeley, “Overexpression of a transporter gene in a multidrug-resistant human lung cancer cell line” SCIENCE-NEW YORK THEN WASHINGTON-., 258), 1650, 1992.
- M. R. Zaidi, G. Merlino, “The two faces of interferon-γ in cancer” Clinical Cancer Research., 17(19), 6118–6124, 2011.
- K. E. de Visser, A. Eichten, L. M. Coussens, “Paradoxical roles of the immune system during cancer development” Nature Reviews Cancer., 6(1), 24–37, 2006.
- A. Weinandy, M. D. Piroth, A. Goswami, K. Nolte, B. Sellhaus, J. Gerardo-Nava, M. Eble, S. Weinandy, C. Cornelissen, H. Clusmann, “Cetuximab induces eme1-mediated DNA repair: a novel mechanism for cetuximab resistance” Neoplasia., 16(3), 207–220, 2014.
- Z. Li, L. Chen, Z. Qin, “Paradoxical roles of IL-4 in tumor immunity” Cellular & molecular immunology., 6(6), 415, 2009.
- J. F. Ferreiro, L. Rouhigharabaei, H. Urbankova, J.-A. van der Krogt, L. Michaux, S. Shetty, L. Krenacs, T. Tousseyn, P. de Paepe, A. Uyttebroeck, “Integrative genomic and transcriptomic analysis identified candidate genes implicated in the pathogenesis of hepatosplenic T-cell lymphoma” PloS one., 9(7), e102977, 2014.
- I. Kryczek, S. Wei, W. Szeliga, L. Vatan, W. Zou, “Endogenous IL-17 contributes to reduced tumor growth and metastasis” Blood., 114(2), 357–359, 2009.
- K. Karri, D. R. Bastola, “Immune based prognostic biomarkers for multiple anticancer therapies in lung adenocarcinoma” in Bioinformatics and Biomedicine (BIBM), Shenzen,China, 2016.