Towards Optimality in Online Learning – The OLeCenT Approach
Towards Optimality in Online Learning – The OLeCenT Approach
Volume 2, Issue 3, Page No 819-838, 2017
Author’s Name: Carl Beckforda), Ezra K. Mugisa
View Affiliations
Department of Computing, University of the West Indies, Jamaica
a)Author to whom correspondence should be addressed. E-mail: carl.beckford@uwimona.edu.jm
Adv. Sci. Technol. Eng. Syst. J. 2(3), 819-838 (2017); DOI: 10.25046/aj0203103
Keywords: Learning Management Systems, Course Delivery, Learner-centric, Online Learning
Export Citations
Higher Education Institutions (HEIs) employ Learning Management Systems (LMSs) primarily for greater efficiency, profitability, technological advancement or survival. The predominantly used LMSs, Moodle and Blackboard account for in excess of 60% usage by the top HEIs. However, the individual international regions do not necessarily bear the percentages of the overall total. Gaps are identified in optimality in course delivery within online learning when one studies LMSs and their functionalities. Advanced Distributed Learning (ADL) Initiative which was established to standardize and modernize training and education management and delivery, developed and recommended usage of Sharable Content Object Reference Model (SCORM) 2004 and later versions. SCORM 2004 which provides for flexibility in sequencing and navigation for learner-centric course delivery is not supported in any version of the more prevalently used LMSs. It is believed that most people have a preferred way in processing information. We propose codifying one or more Learning Style Instruments (LSIs), diagnosing the preferred teaching approach(es) and dominant/existing learning styles within a batch of learners, then providing course delivery as a best-fit per learner. As a proof of concept, OLeCenT allows the input of one or more course learning paths with real-time learning and automatic reconfiguration of the course path where a new trend or pattern is identified. OLeCenT identified disparity in teaching-learning and provided a mechanism towards improving online learner-centric course delivery. OLeCenT also identified comparative levels of similarities among learners and instructors even where they are deemed to be of different teaching-learning styles/mechanisms.
Received: 06 April 2017, Accepted: 19 May 2017, Published Online: 19 June 2017
1. Introduction
For the formative years, the primary mode of knowledge transfer was through the face-to-face mode of delivery. Eventually knowledge was captured in writing which provided an alternative to the usual face-to-face delivery. As technology developed, new ways were found to transfer knowledge. This paper is an extension of work originally presented in Future Technologies Conference 2016 which describes a tool to enhance learning [1]. The development of technology also made available the combination of different forms of learning [2-3]. Amidst these, the need for the initial and most established mode of delivery still has its significance [4-6].
Whether through face-to-face or alternative means of course delivery, differences between the learners’ approach to learning or styles of learning and the teacher’s teaching mechanism or approaches within a batch of learners and teachers may lead to various rates of learning and a non-optimal level of learning in the overall batch of learners [7-9]. Identifying where there is a teaching-learning style mismatch and solving same can help achieve a resultant increase in the level of learning, and should free a teacher to more ably deal with special cases such as learners with special needs or develop varied course material and course delivery mechanisms to assist additional learners.
2. Teaching-Learning in Higher Education Institutions
We examine teaching-learning in Higher Education Institutions, discuss theories of teaching and learning, and analyze the usage of learning mechanisms or styles in Learning Management Systems. These are used to propose OLECENT, an approach in view of learner-centric course delivery. We describe the features, results and benefits of the derived artifact, OLeCenT, an e-Learning Tool towards optimising the teacher-learner interaction [10-11].
2.1. Teaching-Learning using Learning Management Systems
Learning Management Systems (LMSs) are used worldwide by Higher Education Institutions to assist with efficiency in course delivery or management [12-13]. There are different types of LMSs, which all work slightly differently but ultimately perform the same or similar functions and can deliver the same learning materials with different levels of efficiency [14]. Higher Education institutions tend to have one of the following three categories of LMSs in operation: off-the-shelf or proprietary, open source, or bespoke [15].
In an analysis of top universities in each international region, home-grown self-used systems accounted for about 17%, Sakai accounted for about 10% and Canvas accounted for only 5%. Usage of 40% and 21% by Moodle and Blackboard respectively confirm the dominant players in the LMS market. However, the individual regions do not necessarily bear the percentages of the overall total; for example, top universities of Latin American reflect a 100% usage of Moodle [16].
A detailed review of the four named systems was done based on features related to direct or indirect relevance to the level of learning, the finite number of human teachers, and resources or system design which may affect positively or negatively the teaching-learning process. In view of [17-30], Table 1 reflects the comparison of LMS features that are important in mitigating the problem of course delivery being static or erroneously less learner-centric.
Noteworthy are the features of Learning Management Systems that are not fully offered by any of the predominantly used learning environments. These are:
- Provide for assessment of learning and teaching styles
- Offer course delivery path/workflow to each learner to match learner’s dominant learning style
- Provide options for learner to receive course delivery in his non-dominant or other learning styles
- Allow input and usage of text unique to courses
- Allow for integration of activity simulation where practical knowledge is to be transferred
- Allow instructor to modify course path according to students’ expectations and/or indications from course beginning to end, in terms of transfer of knowledge and necessities for emphasis for repeats
- Provide for assessment which equates to or exceeds the traditional mode but matches the style of learning
- Provide for learner-centric scheduling of synchronous activities where such include online activities
Other LMSs or tools had some features but were significantly lacking in others. E.g. Intelligent Tutoring Systems (ITS) represents some of the knowledge and reasoning of good one-to-one human tutors, as it attempts to simulate a human tutor’s behaviour and guidance [31].
Table 1: Comparison of LMS Features that are important in mitigating the Problem of Course Delivery being Static or becoming less Learner-Centric
Feature | Learning Management Systems | |||
Blackboard | Canvas | Moodle | Sakai | |
Improvement for lack in the traditional with use of tools in the online environment | ||||
Provide diagnostic assessment of learning/teaching styles | ||||
Restrict and/or provide sections of course path based on pre-entered conditions or complex access criteria | √ | √ | ||
Modify course delivery path/workflow to match dominant learning style in batch of learners | √ | √ | √ | √ |
Offer course delivery path/workflow to each learner to match learner’s dominant learning mechanism / style | ||||
Provide options for learner to receive course delivery in his non-dominant or other learning styles | ||||
Automatically determine loss of learning of learners prior to assessment(s) | √ | √ | ||
Alert instructor and/or learner of loss of learning | √ | √ | √ | √ |
Allow instructor to instantly assess student comprehension | √ | √ | √ | √ |
Provide instructor with a view of the student’s perspective | √ | √ | ||
Allow students to understand their own progress towards mastering key outcomes | √ | |||
Equitable benefits to all types of courses and maintain/improve on tools in the traditional | ||||
Allow input of all types of course material | √ | √ | √ | √ |
Allow input and usage of text unique to courses | ● | ● | ||
Provide for use of external tool seamlessly within the learning environment | √ | √ | ● | ● |
Allow for integration of activity simulation where practical knowledge is to be transferred | ● | ● | ● | ● |
Align content items to objectives and goals | √ | √ | ||
Allow students to add personal course artefacts that improved their learning | √ | √ | ||
Provide for Collaboration | √ | √ | √ | √ |
Allow for student feedback outside of those pre-determined by instructor | ● | √ | √ | ● |
Allow instructor to modify course path according to students’ expectations and/or indications | ● | |||
Provide for assessment which equates to or exceeds the traditional mode but matches the style of learning | ||||
Integration of administrative information or functionalities that may aid in learning | ||||
Allow input of teaching-learning schedule of course | √ | √ | √ | √ |
Use teaching-learning schedule for notifications | √ | √ | ||
Provide for learner-centric scheduling of synchronous activities where such include online activities | ● | |||
Mitigate falsehood especially in assessment | √ | |||
Allow HEI to offer course to learners worldwide through MOOC | √ | |||
Permit customization so that HEIs can add according to holistic or long-term learner profile | √ | √ |
Key: √ – feature is fully offered
- – feature is partially offered
blank – feature is not offered
2.2. Limitations of Learning Management Systems
There are documented common complaints about Learning Management Systems. Whereas all are genuine complaints, the only complaint that is identified to be of significant interest for this research is that LMSs do not fit in the existing administration workflows [32]. A key reason to implement a LMS is to support efficient training administration. So, if this isn’t working for you, then it is a real problem [32]. We concur with [32] that when selecting a LMS, the HEI should be sure to map the existing administration and end user workflows, and use these maps to communicate your LMS implementation requirements. However, we purport that the LMS implementation requirements should be guided by best practices as well as best-case possibilities available in view of existing skills, resources, and achievements.
Blackboard’s market strategy over the years (acquisition), Moodle’s significant growth, and the arrival of Canvas (the first significant new product in this space in at least 8 years) tell interesting tales [33]. The various Learning Management Systems have delved into course management and delivery, and some have aimed for improved learning. Not many have designed or implemented a system to manage learning in view of the learner’s behavior or learning style. The emergence of MOOC platforms, which for the high-profile MOOC initiatives such as Coursera and edX are homegrown products may be the ideal platform to have self-paced managed learning where learning is tracked and attempts made during the learning process to enhance the level of learning.
It is possible that Learning Management Systems (LMS) are not equivalently suited for all types of courses [34]. Where a course’s primary aim is to transfer knowledge with respect to a practical achievement of a task, as opposed to a description or narrative view of an issue. This view could be used to support the view that LMSs may be more advantageous to certain types of courses. Among respondents who were engaged in a programming course delivered with use of a LMS, the communication and discussion aspects of the course were rated among the highest and guidance to solving problems among the lowest [34]. Although LMSs are deemed beneficial, they fall short in some aspects specific to computer science education, in particular programming. A Learning Management System lends itself to web-based learning environments by enabling the management of courses, delivery of content and lectures, assessment and tracking of student engagement with the course. The implementation of a worldwide networking course by Cisco Networking Academy which seeks to transfer the knowledge about a practical task should provide some encouragement for what is possible for other course content such as computer programming.
We are in the midst of learner-centric shifts in education, and the drivers for these changes are social and economic, not just technological [35]. A distinction is made between competency based learning (CBL) which focuses on learning outcomes rather than competency based education (CBE) which is based on time. The emphasis on the knowledge transfer to the learner being the guide for success is an essential aspect of old, existing or new Learning Management Systems.
A Delta Initiative analysis points out that most of the core Learning Management Systems were developed between 1997 and 2004, and there really has not been many new pedagogical capabilities added since that time. Instead, innovation has been driven by a horizontal expansion of business areas, e.g. interoperability with peripheral systems for portals, portfolios, ERP integration, and the ERP systems themselves [33]. As interoperability is achieved, improvements in pedagogical capabilities are achieved. The advancement of technology and the use of computing resources could assist in this way.
In referring to examinations or other forms of assessments, students have a strong tendency not to learn for life, but “to the test” [36]. A mismatch or misalignment [37] in form or content between examination tasks and learning objectives will lead to students engaging in the wrong learning activities and acquiring the wrong competences [36]. Multiple-choice tests are perhaps the most infamous example of such mismatch and the associated detrimental effects, decried for fostering surface learning and student disengagement [38]. Such a mismatch is likely to alienate students and erode their learning motivation [36]. The possibility of mismatch or misalignment suggests the need for LMSs that ensure that learning motivation is not eroded, course delivery is competence-oriented [39], as well as a LMS that mitigates the mismatch between examination tasks or other course delivery activities and learning objectives.
There are three functional areas the LMS is designed to support: Course Administration, Communication and Collaboration, and Custom Educational Content. One of the most important things an academic technologist can deliver to faculty is to show them how the LMS can be used for more than just a platform for course administration but to teach in new ways [33]. This implies that (1) there may be ways provided by the LMS to teach or improve teaching exists but are not being employed, (2) there may be new ways of teaching that may be inspired and developed with use of a LMS that have not been explored, (3) there may be new ways of teaching that are inspired by external factors to the LMS, maybe through the face-to-face environment, that may be developed as new features to the LMS.
Amidst the many Learning Management Systems and even the smaller number of those that are used predominantly by HEIs, Columbia University has used aspects of at least six of these, namely Sakai, Moodle, Angel, Canvas, eCollege and Coursera [33]. The change from one LMS to another suggests a feature, functionality, improvement or enhancement in at least one aspect of course administration, communication and collaboration, and providing educational content, which was in one system above another. Therefore as technology develops and the area of e-learning or online learning expands, newer functionalities and enhancements may become possible.
3. Consideration of Learning Styles in Learning Management Systems
Education has always had new ideas about learning and teaching. Educators are asked to use new curricula, new teaching strategies, and new assessments. They are directed to prepare students for the new state standardized test or to document and assess students’ work through portfolios and performance assessments [40]. While classroom teaching and management strategies are well documented, the online learning environment presents different challenges and benefits [41]. Teaching in an online environment requires a special set of teaching skills since many of the strategies and tactics associated with best teaching practices are somewhat constrained by the primarily text-based environment [41]. Implicitly, teaching could be taking place in the online environment yet learning is not taking place or certainly not at the level at the perception of the teacher or instructor.
3.1. Theories of Teaching and Learning
Many theories of teaching and learning have been purported and/or established. Some of these are Cognitive Load Theory of Multimedia Learning, Maslow’s Hierarchy of Needs, Cognitivism, Experiential Learning (Kolb), Constructivism, ADDIE Model, ARCS Model of Motivational Design, and Multiple Intelligences Theory [42].
Cognitive Load Theory of Multimedia Learning discusses that there are three types of cognitive load: extraneous cognitive load, intrinsic cognitive load, and germane cognitive load. The intrinsic cognitive load embodies the idea that all instruction has an inherent difficulty associated with it. This inherent difficulty may not be altered by an instructor. However many schemas may be broken into individual “subschemas” and taught in isolation, to be later brought back together and described as a combined whole [43, 44]. It is important that systems of learning provide for segmentation or combining of learning units to facilitate the intrinsic cognitive load. Extraneous cognitive load, is under the control of instructional designers. Where possible, the ideal LMS provide for the setup and automation of learner paths. Extraneous cognitive load and intrinsic cognitive load are not ideal; they result from inappropriate instructional designs and complexity of information. Germane cognitive load is coined as “effective’ cognitive load, caused by successful schema construction. Each of the cognitive loads are additive, and instructional design’s goal should be to reduce extraneous cognitive load to free up working memory [43]. The ideal LMS should model aspects of the human cognitive architecture, and provide for ease of use by course designers in their endeavor to apply sound instructional design principles.
Abraham Maslow’s theory, Hierarchy of Needs is a motivational theory in psychology that argues that while people aim to meet basic needs, they seek to meet successively higher needs in the form of a hierarchy. Maslow presented the idea that human actions are directed toward goal attainment. Any given behavior could satisfy several functions at the same time; for instance, going to a pub could satisfy one’s needs for self-esteem and for social interaction [45]. Maslow’s Hierarchy of Needs has often been represented in a hierarchical pyramid with five levels. The four levels (lower-order needs) are considered physiological needs, while the top level is considered growth needs. The lower level needs need to be satisfied before higher-order needs can influence behavior [45]. Development and implementation of a learning system or tool must consider that the body of learners as well as instructors and teachers would tend to follow Maslow’s Hierarchy of Needs.
The theory of Cognitivism says that the learner is viewed as an information processor (like a computer). Cognitivism focuses on the inner mental activities – opening the “black box” of the human mind is valuable and necessary for understanding how people learn [46]. The ideal learning system should provide for some capture of each learner’s learning mechanism/style/intelligence. In view of the theory, ensuring that stimuli exist within a Learning Management System will contribute to the system being more learner-centric.
The theory of Constructivism says that learning is an active, constructive process. The learner is an information constructor. People actively construct or create their own subjective representations of objective reality. New information is linked to prior knowledge, thus mental representations are subjective. Knowledge is constructed based on personal experiences and hypotheses of the environment [46]. This theory is used to support the proposal that the system should provide for basic information access needs such as system user-friendliness and ease of use, to allow for ease of transition in a learner using the system to increase the level of learning. The theory says that learners continuously test the hypotheses of the environment through social negotiation. Each person has a different interpretation and construction of knowledge process. The learner is not a blank slate but brings past experiences and cultural factors to a situation [46]. Past experiences and cultural factors is used to support for a diagnostic mechanism that ascertains learner preferences or other factors that may influence learning. Constructivism assumes that all knowledge is constructed from the learner’s previous knowledge, regardless of how one is taught [46]. Thus, even listening to a lecture involves active attempts to construct new knowledge.
Constructionism builds up the theory of Constructivism. Constructionism is ‘learning by making’. Constructionism shares constructivism’s connotation of the learning. It then adds the idea that this happens especially felicitously in a context where the learner is consciously engaged in constructing a public entity [47]. The theory of constructionism proposes that learning may be enhanced through kinesthesia. A Learning Management System should facilitate kinesthetic learning objects.
The theory of Multiple Intelligences identifies seven distinct intelligences. The theory says that we are all able to know the world through language, logical-mathematical analysis, spatial representation, musical thinking, the use of the body to solve problems or to make things, an understanding of other individuals, and an understanding of ourselves. Our educational system is heavily biased toward linguistic modes of instruction and assessment and, to a somewhat lesser degree, toward logical-quantitative modes as well [42]. It is argued that the broad spectrum of students would be better served if disciplines could be presented in a numbers of ways and learning could be assessed through a variety of means. It may seem impossible to teach to all learning styles [42]. However, as we move into using a mix of media or multimedia, it becomes easier. As we understand learning styles, it becomes apparent why multimedia appeals to learners and why a mix of media is more effective [48, 42]. The theory of multiple intelligences satisfies the many types of learning preferences that one person may embody or that a class embodies.
3.2. Teaching and Learning Styles
Learning styles are ways of learning presumed to allow individuals to learn best [49,50]. It is believed that most people have a preferred way in processing information [51]. There are a number of instruments developed for determining learning styles. Some of these are Witkin’s Field-Dependence/Field-Independence, Kolb’s Experiential Learning Model (ELM) and Learning Style Inventory (LSI), Hunt et al.’s Conceptual Level Model, Dunn et al.’s Learning Styles Inventory (LSI), Fleming et al.’s VARK Model, C.I.T.E Learning Styles Instrument, and Gregorc Learning Style Model [52]. Learning styles has a bad press. It seems that they are lauded and then attacked on an almost cynical basis [53]. This is probably because it is very difficult to measure learning (in part because it is difficult to define learning in useful ways), especially if one wants to know when learning happens or to what it can be ascribed. The critics of learning styles say things like ‘Knowing ones learning style does not improve learning.’ However knowing one’s learning style can be beneficial if learners take the next step, and consider how and when they learn as part of a reflective meta cognitive process, with action to follow [53].
Predominantly, it was stated that there were three learning styles, namely auditory, tactile/kinesthetic and visual [54,55]. Currently it is more accepted that there are more than three learning styles [48,31]. There are discussions whether intelligences are styles or whether they should be considered as learning styles [56]. Gardner says that intelligences are not the same as learning styles. Intelligences are mental computers of varying strength, while styles are the ways in which individuals putatively approach diverse tasks [42]. The prevalently used instruments were chosen for the research, with consideration given to the level of their accessibility. Consequently, the learning styles models discussion includes Fleming et al.’s VARK Model, Kolb’s Experiential Learning Model (ELM) and Learning Style Inventory (LSI). C.I.T.E Learning Styles Instrument was incorporated primarily due to its accessibility.
3.2.1 VARK Model of Learning Style
The VARK Model evolved from the VAK Model. The Visual, Auditory and Kinesthetic (VAK) learning style model is a common and widely-used model of learning style. According to this model, most people possess a dominant or preferred learning style; however some people have a mixed and evenly balanced blend of the three styles of visual learners, auditory learners and kinesthetic learners. In summary visual learners tend to learn through seeing and think in pictures, auditory learners tend to learn through listening and have highly developed auditory skills, and kinesthetic learners tend to learn through moving, doing and touching and expressing themselves through movement [38]. With use of an appropriate instrument, the ideal Learning Management System should provide for ascertaining the difference in styles of the learners; this should aid in the system’s provision of a learner-centric course delivery.
VARK is an acronym for Visual, Aural, Read/Write and Kinesthetic. The VAK inventories had been around for years until expanded by the inventor of the model. The inclusion was a second ‘visual’ modality for Read/Write learners. From observation some students have a distinct preference for the written word whilst others preferred symbolic information as in maps, diagrams, and charts [53]. These two preferences were not always found in the same person. He also said that there is more acceptance of this distinction today than in the 1980s [53]. Fleming’s VARK Model is suggesting that the visual and auditory/aural aspects of the previous VAK model did not capture ideally the read/write practices or preferences that were exemplified by some learners. The Learning Management System that is best suited for the learner should be able to distinguish a learner who is visual, aural or other or combinations of these styles.
3.2.2 Kolb’s Learning Style Model
The Kolb’s Experiential Learning Model and Learning Style Inventory (LSI) has been called the Kolb Learning Style Model, a 9-fold classification of learning styles with combinations of feeling, watching, thinking and doing/acting scored as a 12-item self-report questionnaire [51]. Kolb’s learning theory sets out four distinct learning styles (or preferences), which are based on a four-stage learning cycle. The model offers both a way to understand individual people’s different learning styles, and an explanation of a cycle of experiential learning that applies to us all. Kolb says that ideally (and by inference not always) this process represents a learning cycle or spiral where the learner ‘touches all the bases’, i.e., a cycle of experiencing, reflecting, thinking, and acting. Immediate or concrete experiences lead to observations and reflections. These reflections are then assimilated (absorbed and translated) into abstract concepts with implications for action, which the person can actively test and experiment with, which in turn enable the creation of new experiences [51]. The current Kolb Learning Style Model reflects an enhancement to a former version of the model [57]. As knowledge concerning learning styles increases and learning style theory, mechanism and instruments improve, there should be flexibility in a Learning Management System to facilitate ease of adaptability to the changes.
Kolb explains that different people naturally prefer a certain single different learning style. Various factors influence a person’s preferred style, notably in his experiential learning theory model (ELT). The discussion on experiential learning theory is not included as it should not affect the approach for Learning Management Systems and the use of this approach [51]. The determination of a learning style is done by the following of a protocol or rules that govern the assessment process. The ideal learning environment that provides mechanism for assessing learners should provide for the seamless creation and modification of rules per learning style and per learning style instrument that may vary with an institution’s selection of learning style approach. Knowing a person’s learning style enables learning to be orientated according to the preferred method. Everyone responds to and needs the stimulus of all types of learning styles to one extent or another – it’s a matter of using emphasis that fits best with the given situation and a person’s learning style preferences [51, 58].
Whereas there has been support for the Kolb Model, there has been some criticism. The concept of learning styles has been criticized by many and experts suggest that there is little evidence to support the existence of learning styles at all. One large scale study looked at more than 70 different learning style theories and concluded that each lacked enough valid research to support its claims [59]. In a 2008 article, educator Mark K. Smith argued that Kolb’s model is supported only by weak empirical evidence and that the learning process is actually far more complex than the theory suggests. He also noted that the theory fails to fully acknowledge how different experiences and cultures may impact the learning process [59]. Additionally, [60] says that despite the various refinements of Kolb’s inventory, the instrument still appears to possess several weaknesses which limit its use, including low reliability, questionable validity, and low predictive powers. The instrument presupposes that individuals can only possess one learning style. Their study says that although the four modes suggested by Kolb may be valid, little evidence is provided to confirm Kolb’s two dimensions. They suggest three unidimensional factors, where the resulting learning style, will be based on one’s relative position on each of the three dimensions [60].
Amidst the criticisms, [60] states that in 2005 amidst a list of 71 learning style models, Kayes chose Kolb’s model as the most influential learning style model. Kolb Model has still been in usage across the world [61]. Kolb’s Experiential Learning Model (ELM) and Learning Style Inventory (LSI) is the preferred model for inclusion in a Learning Management System. Notwithstanding, the ideal learner-centric Learning Management System should provide for setup of multiple learning style instruments and capability to select or activate the one(s) to be used per institution, per course or per period.
3.2.3 Teaching Style
By experimenting with innovative strategies intended to help students learn who formerly had not been successful, [62] found out that most methods and/or resources appeared to work with some students but not others. It was said further that in many cases, those who were successful with a particular method or set of materials had essentially similar characteristics. Repeatedly, data revealed that when taught through methods that complemented their learning characteristics, students at all levels became increasingly motivated and achieved better academically. If it is true that 90 percent of all instruction is conducted through either lecture or lecture discussion, it is no wonder that so few students achieve as well as we believe they should [62]. The research by Dunn & Dunn suggests that similar to how learners may favour a particular learning style or mechanism, a teacher or instructor may favour a particular style, mechanism or approach in providing knowledge or information to the learner.
Dunn & Dunn says that for decades, supervisors have been evaluating faculty in an effort to isolate those characteristics that produce effective instruction. Their efforts have been misdirected by weaknesses both in their assumption and their basic designs [62]. The attitude teachers hold towards various instructional programs, methods, and resources as well as kinds of youngsters they prefer working with constitute part of their “teaching style”. Teachers can assess themselves with an instrument that simultaneously identifies their teaching style and reveals the areas that need to be expanded to respond to additional characteristics [62].
In summarizing the paper, Learning Styles/Teaching Styles: Should They… Can They Be Matched? [62] answers the question “Why not just match teachers with students?”. The paper purports that the fallacy is this (a) learning style and teaching style characteristics do not always cluster into such neat packages; (b) students are not consistently one way or the other – nor are teachers; (c) neither traditional nor informal teachers are necessarily excellent, and it is possible to match a student’s learning style and a teacher’s teaching style and still not provide that youngster with an effective teacher; and (d) given the practical, “how-to” skills for teaching students through their individual learning styles, most teachers can become effective with most students and, simultaneously, provide a humanistic care, nurturing atmosphere [62].
We have concurrence with the views of [62]. However, within a Higher Education Institution, where the human teacher may be the primary source of course delivery, he/she is not necessarily provided with the resources of time to ensure that all avid learners being taught at a particular time are at their highest level of learning.
The analysis was done on a BTEC Applied Science course to determine whether student-centred learning activities improve learning outcomes [63]. The research states that deeper learning was significantly improved after instructional teaching as opposed to student-centred teaching. In the research, preferences for teaching style were also obtained from the students [63]. This research indicates that teaching style is a contributing factor to the level of learning. Where a Learning Management System is able to provide a teaching style – learning style match, the learning process may be aided. However, within a Higher Education Institution, where the human teacher may be the primary source of course delivery, he/she is not necessarily provided with the resources of compensation or training to ensure that all avid learners being taught at a particular time are at their highest level of learning.
In the discussion of teaching style, one may imagine the teacher or instructor being a computer software program or general form of Computer-Assisted Instruction (CAI) [64]. Of note to this research is the Intelligent Tutoring System (ITS) in Learning. We make note of [65] in their work for argument-making in higher education. The best result of 81.74% in classification correct rate was obtained when all grade classes were used [65]. It was said that while CAI may be somewhat effective in helping learners, they do not provide the same kind of personalised attention that a student would receive from a human tutor. One-to-one tutoring allows learning to be highly individualized and consistently yields better outcomes than other methods of teaching. Unlike previous CAI systems, ITS represents some of the knowledge and reasoning of good one-to-one human tutors, and consequently can coach in a much more detailed way than CAI systems. ITS does not just provide feedback such as correct or wrong answers for the student’s mathematics solution but it also provides details of the misconception that the student face. The Student Model assesses student’s knowledge and makes a hypothesis on the conceptions and reasoning strategies employed by the student to achieve his/her knowledge state [66]. Findings suggest participants’ learning capacity of the introductory statistics could be improved successfully when CAI used as a supplement to regular lecture in teaching introductory statistics course [64].
The mechanism without the rules of Kolb’s Model was attainable without a cost. For the C.I.T.E. Learning Styles Instrument, both the mechanism and detailed rules for implementation were available.
3.3. Current Status of LMSs incorporating Learning Styles and Sequencing
The Advanced Distributed Learning (ADL) Initiative was established in 1997 to standardize and modernize training and education management and delivery. To meet its mission, the ADL Initiative developed the Sharable Content Object Reference Model (SCORM) and the ADL Registry. The release in 2009 is SCORM 2004 4th Edition. ADL supplies resources for SCORM 1.2, SCORM 2004 3rd Edition, and SCORM 2004 4th Edition. ADL recommends use of SCORM 2004 4th Edition [67]. Some consider the Experience API (Tin Can API) released in 2013 with currently in excess of 170 adopters, to be the successor to SCORM [68].
Further, the IMS Global Learning Consortium (IMS GLC) is a global, nonprofit, member organization that strives to enable the growth and impact of learning technology in the education and corporate learning sectors worldwide. Consequently, IMS develops Learning Tools Interoperability (LTI) to allow remote tools and content to be integrated into a Learning Management System (LMS). In addition to LTI, IMS GLC developed another standard, Common Cartridge. IMS Common Cartridge (IMS CC) provides a standard way to represent digital course materials for use in online learning systems so that such content can be developed in one format and used across a wide variety of learning systems, as well as enable new publishing models for online course materials and digital books that are modular, web-distributed, interactive, and customizable.
The three standards described, namely Sharable Content Object Reference Model (SCORM), Learning Tools Interoperability (LTI) and Common Cartridge have been employed in learning management systems in varied levels. The main systems in use which provide such functionality are Blackboard which is proprietary, and Moodle, Canvas, Sakai which are open source.
SCORM 2004 is not supported in any version of Moodle. Parts of the API have been implemented, but sections such as Navigation and Sequencing, which will assist in learner progress and assessment detailed tracking, have not been implemented. Moodle has announced that development on native SCORM 2004 support in the system has stopped. They further suggest that where a fully certified SCORM 2004 Player in Moodle is desired, Rustici Software have a Moodle plugin which connects to their commercial SCORM Cloud service turning Moodle into a fully compliant SCORM 2004 LMS [42].
Where there is a human teacher as the primary source of course delivery, he/she is not necessarily provided with the requisite resources to ensure that all avid learners being taught at a particular time are at their optimal level of learning. From the concept of levels of learning by [10] and the discussion of optimal learning by [11], the optimal level of learning is the highest level of learning achievable in a given time and nature of the uptake function; we consider the nature of the uptake function to include the learner, learning environment and learning style [69]. Learners are expected to comprehend the material within the mode made available by the teacher. All learners in a particular batch tend to be governed by the path of learning carved out by the teacher in his/her expertise and experience. Whereas the teacher has the knowledge to be transferred and is better able to determine the path to be taken based on prior batches of students, the flexibility does not exist at the point of learning for a change of path or delivery mode. There is also often disparity between the mode employed for course delivery and assessment, such as oral versus written or electronic versus hardcopy. Hence, as the number of learning styles may increase as the population within a batch of learners increases, there is an increasing tendency away from the optimal level of learning, amidst the finite or a limited number of course delivery paths and human teachers, and the current design of learning management systems.
4. OleCenT – The Online Learner-Centric Tool
4.1. The OLeCenT Approach to Course Delivery
In the interest of a positive effect on the level of learning, we propose OLeCenT, a tool for learner-centric course delivery in the online environment. OLeCenT may be integrated with a Learning Management System for enhanced course administration. We embrace the integration of learning styles to achieve a maximal matching [70] with the teaching styles. Teaching-learning in higher education institutions is examined with an analysis being done on course delivery in view of learning styles. We suggest how instructional design may be applied amidst a standard and specification for web-based e-learning with emphasis on how learning takes place.
4.1.1 The Underlying Theories of the OLeCenT Approach
We are influenced by [71] that computing impacts the three necessities of teaching-learning, namely basic skills instruction, advanced skills instruction and assessment of student progress, as well as the types of learning. We discuss the learner-centric tool by examining teaching and learning styles in a graph theory context.
4.1.1.1 OLeCenT and Graph Theory
By examining teaching and learning styles in a graph theory context, we propose an approach for course delivery, and describing elements and standards of the learner-centric model. The learner-centric tool may be designed for any of the sets of learning styles. We propose [51] Learning Styles Inventory 9-fold classification of concrete experience (feeling), reflective observation (watching), abstract conceptualization (thinking), active experimentation (doing), diverging (feel and watch), assimilating (think and watch), converging (think and do), accommodating (feel and do) and perception continuum (feel, watch, think, do). This model provides flexibility in terms of basic learning styles and diverse combinations of these styles. It is believed that this 9-fold classification engulfs others such as the seven stated in the classification by [48].
We propose that in the context of all teaching and learning styles, the relation for teaching-learning styles is a bijective function [70]. A teaching-learning style is a teaching style that provides greatest learning for (matches) a particular learning style, and vice versa. Considering a teaching-learning unit such as a course or a session of learning where knowledge transfer is the aim, there is a set of teachers and a set of learners. Correspondingly, there are sets of teaching styles, learning styles, and teaching-learning styles (the matching between a teaching style and a learning style).
Let G be a directed, bipartite graph with disjoint vertices T (the set of teaching styles, t1, t2, …) and L (the set of learning styles, l1, l2, …) in which the edges are directed from T to L (Figure 1). We propose that in any teaching- learning unit, optimal learning occurs where there is a maximal matching [70] for G with all elements of L having a pre-mapping (maximal matching with surjectivity) (Figure 1a, Figure 1b.) even where a complete matching does not exist (Figure 1b). Optimal learning may exist even where there is no surjective (onto) mapping as the same may exist where a teaching style exists in a teaching learning unit which has no mapping to a learning style in that unit (Figure 1b). Where there is a complete matching, that is with all teaching styles having a matching (Figure 1a, Figure 1c), non-optimal learning may still occur (Figure 1c) as all learning styles may not be catered to. For large learning groups with the number of learning styles tending towards maximum, invariably there are learning styles not mapped and far from optimal learning (Figure 1d). For learner-centric course delivery in e-learning, we propose that maximal matching with surjectivity for the teaching-learning styles is attainable with use of the learner-centric tool and how the LMS is implemented.
4.1.1.2 OLeCenT and the Push-Based Model
The push-based (build-to-stock) model of supply chains is built on the basis that the supplier or provider of goods or service knows best as to what goods or service are needed [72]. This build-to-stock model schedules production based on best guesses of demand and the availability of raw material in inventory. Considering the teachers’ “raw material” or availability of teaching styles, some HEIs operate in a push-based model where the course content is provided in a mode as a best guess of demand. We propose that the pull-based approach (demand-driven) be used in course delivery where the customer orders trigger events in the supply chain [72]. The desire for learning should not be what triggers what is taught (course content) but rather the learning style (for the course content) should trigger events for the material to be provided in a particular style.
The pull-based model is similar to the self-examination mentioned by [71] in the PRECALC project or may be likened to diagnostic and formative assessments with benefits for the current year or summative assessments with benefits for the ensuing year. Research provided for the three initially proposed as the existing learning styles, namely visual, tactile, and kinesthetic [71]. The learner-centric tool also provides for learning styles which are combination of these as well as other forms of learning styles [51]. Further, formative and summative assessments primarily tend to be used to assess for knowledge gaps or knowledge acquisition respectively, rather than for an assessment of teaching style(s) to be employed. Even where these are used for this purpose, it must be noted that benefits can only accrue subsequent to the time of assessment.
4.1.1.3 OLeCenT and Supply-Demand Theory
The learner-centric tool should cause a lessening of the gap between teaching and learning styles within a batch of learners. Some learners should be more comfortable with the course material as it is received in a manner closer to the type of learning to which they are adept. The avid learner is not first required to “translate” course material from another teaching-learning style to ensure his/her optimal learning. Learners within the same batch benefit during the teaching-learning process as opposed to the trend where changes are put in place for subsequent batches

Figure 1: The Teaching Styles (T) and Learning Styles (L) Matching reflecting when optimal learning (7a and 7b) or non-optimal learning (7c and 7d) is achieved. The figure shows various combinations of complete matching, maximal matching and surjectivity
of learners. This is because the tool implements discovered trends or learning patterns of the current learners. The provision of learning objects specific to an individual’s learning style should minimize the need to complement or supplement their learning with non-LMS sources. There is therefore a seamless integration required for fluidity of learning and a mitigating of the teachers’ concerns for the use of material without approval.
In examining market mechanism, [73] states that the supply curve tells us how much producers are willing to sell at various prices, and the demand curve tells us how much consumers are willing to buy at these prices. The two curves intersect at the equilibrium point [73] where quantity demanded is equal to quantity supplied. In our study the aim is for optimal learning collectively and individually. Applying the supply and demand curve principle within a course and considering how much (teaching styles) teachers are willing, able or allowed to provide, and how much (learning styles) learners are willing to adopt, we propose that there exists a teaching-learning equilibrium (A) where teaching-learning tends to operate. With the learner-centric tool the level and speed of learning should increase and there should be an upward movement of the teaching-learning equilibrium point (A à B) (Figure 2). Figure 2 is constructed based on the following assumptions: (1) as the number of teaching styles increases, ceteris paribus, the level of learning increases because there is a higher likelihood that a learner will find a matching teaching style (T1), and (2) similarly as the number of learning styles increases,
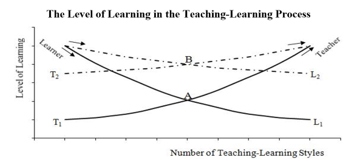
Figure 2: Relation between the Level of Learning and the Number of Teaching-Learning Styles for the human Teacher and the Learner in the Teaching-Learning process
ceteris paribus, the level of learning decreases (L1). The tool provides a shift in both teaching (T2) and learning (L2) curves.
The graph shows expectations before (solid) and after (dashed) the use of a learner-centric course delivery tool. The teaching-learning styles axis represents the teaching styles for the teacher graphs (T1 and T2), and the learning styles for the learner graphs (L1 and L2).
4.1.2 Standards of the Learner-Centric Tool
One of the essential elements of the learner-centric tool is the emphasis placed on the learners and the determined learning style(s). We propose that in instructional design, learning style should drive course delivery and correspondingly course assessment (Learning Style à Course Delivery à Assessment). We apply Bloom’s taxonomy and suggest examples of measurable learner outcomes to assess the learner-centric tool. The Instructional System Development model is adopted with specifics stated for the tool. We then state not only that the learner-centric tool is SCORM compliant but also how the SCORM categories should be used with the learner-centric tool.
4.1.2.1 Educational Objectives
Bloom’s Taxonomy of Educational Objectives is widely used for organizing levels of expertise. Reference [10] states the three overlapping domains of educational objectives as cognitive, psychomotor and affective and based on the expected knowledge transfer within a course, the specific domain is identified. We propose the application of Bloom’s Taxonomy to determine the learner’s expertise in using the learner-centric tool. The learners’ values, attitudes or interests are affected by the course delivery technique embracing teaching-learning styles, therefore the domain of affective goals is the most relevant to assess the learners’ expertise and the effectiveness of the learner-centric tool. We determine each learner’s level of expertise by assessing which measurable learner outcomes have been attained. The levels of expertise are listed in the order of complexity (Table 2). The collective summary of the learners’ expertise suggests the effectiveness of the tool.
4.1.2.2 Instructional Design
Instructional Design models are used to define instructional systems. There are more than one hundred instructional design models, but almost all including the Instructional System Development (ISD) Model are based on the generic ADDIE model [74]. The ISD model is the most well-developed [71, 75] and contains similar phases as the ADDIE model, namely Analysis, Design, Development, Implementation and Evaluation. The phases are sequential sets of activities where the output of each serves as input to the subsequent phase. The learner-centric tool is defined below using the Instructional System Development model. The activities in the various phases for the learner-centric tool do not replace the actual activities for the instructional system but rather complement same. Consequently, good instructional design is expected of the system and these are only superseded where there is a conflict with the activities of the learner-centric tool.
Table 2: Measurable Learner Outcomes for Assessing Learners’ Levels of Expertise in Using the Learner-Centric Tool, based on Bloom’s Taxonomy for Affective Goals [10]
Level of Expertise | Measurable Learner Outcome |
Receiving | Given an option, the learner chooses to use the learner-centric tool. |
Responding | The learner uses optional features of the learner-centric tool such as some of the formative assessments. |
Valuing | The learner seeks out in other learning environments, similar techniques employed by the learner-centric tool. |
Organization | The learner finds differences with the method of learning (such as with the learning outcome) within the learner-centric tool, and the normal face-to-face mode of learning or the LMS without the learner-centric tool. The learner attempts to resolve the mental conflict(s). |
Characterization by a value or value complex | The learner decides to encourage other learners to make use of the learner-centric tool. |
4.1.2.2.1 The Analysis Phase
For the analysis phase the aim is to identify existing deficiencies before the teaching-learning exercise between what is desired and what is existing. The analysis is needed not only for the first delivery of the course content but for each delivery of the same. In addition to the analysis concerning desired outcome and existing knowledge and skills, the tool aids in the discovery of gaps between teaching skills and learning skills. Both teacher and learner are tested to determine teaching-learning skills matching. The human teachers for the course are tested and the teaching styles noted for each teacher as being preferred and/or practiced. Learners are tested with the aim of determining the prevalent learning style and the list of all learning styles in the set of learners. These are grouped into categories of dominant and existing learning styles. The teaching and learning styles resulting from the tests are put in a matrix and used to extract the levels of matching. An analysis document and file are produced with a listing of levels of each teaching-learning style match, levels of each unmatched learning style or combination of learning styles, and unmatched teaching style(s). The results from the analysis may be used by the HEI without the tool but these are generated specifically for and form the basis of the next phase for the design of the tool.
4.1.2.2.2 The Design Phase
In the design phase, the design document is produced to describe the learning objects to be created based on the results from the analysis phase. For each teaching-learning unit (such as material relating to a particular topic of a course), there are specific learning objectives including assessment(s) and corresponding learning objects. For each learning objective, it is determined which teaching-learning style learning objects are to be created to compensate for each teaching-learning style match and unmatched learning style or combination of learning styles. Some learning styles may require more learning objects than others for optimal learning. It is noted which learning objects per learning objective and learning style, already exist in the repository. Priorities are stated for all non-existent learning objects with greater values being given to those for the unmatched learning styles combinations. In LMSs, course designers indicate the workflow of teaching-learning units and the learning objects which relate to the teaching-learning units as well as the direction and timing for display. This exercise continues for the learner-centric tool. Additionally, the designer indicates these workflows per learning style; the actual order of teaching-learning units may vary based on the learning style. The course designer also documents rules as to which learning objects should be omitted based on other options chosen. The rules also indicate the conditions on which to allow the learner-centric tool, based on its learning, to assist in the workflow of the teaching-learning process.
4.1.2.2.3 The Development Phase
The creation of the learning objects is done in the development phase. Within this phase, based on the priorities indicated in the design document, learning objects are created. HEIs may choose not to create learning objects for teaching-learning style matches based on the lack of time before delivery and/or the lack of compensatory resources. However, this decreases the effectiveness of the learner-centric tool. For unmatched learning styles, where the levels indicate that the majority of the learners fall in this grouping, the learning objects are loaded into memory cache. The memory cache is a specialized memory storage used to optimize data transfers resulting in faster and more efficient data retrieval. Based on the workflow, direction and timing of the teaching-learning units and the learner’s current place in the garnering of the course material, the loading of objects into cache is done at run-time as the learner accesses the course environment. The loading of objects into cache is important primarily where learning objects are large or may significantly increase retrieval time. The setting up of the rules given in the design document in the learner-centric tool is done at this juncture. The developer also ensures that the tool has the facility for the learner to set the timing of their learning sessions with the possibility for teacher override. The learning objects created are ensured to be SCORM 2004 Compliant.
4.1.2.2.4 The Implementation and Evaluation Phase
As it relates to instructional design, the implementation phase speaks to the delivery of the course material to the learner. All developments are put into operation and are expected to follow whatever rules were setup. Within the evaluation phase, one ensures greater efficiency and effectiveness based on the results garnered from implementation. The usage of the learning objects per learning objective per learning style is compared to the expected levels indicated in the analysis document. Where the learner-centric tool was allowed to assist in the workflow based on its learning, a determination is made whether the tool appears consistent with the learners’ desires. This information influences decisions in subsequent analyses. Some of the other control mechanisms are the usage pattern of the objects loaded into memory cache, and the level of reuse of the objects.
4.1.2.3 Standards for Web-based e-Learning
The learner-centric tool is SCORM 2004 4th Ed. Compliant but may be developed with less effectiveness to be compliant with other editions of SCORM 2004. The Sharable Courseware Object Reference Model (SCORM) is a collection of standards and specifications for web-based e-learning [76]. The parts of SCORM 2004 are the Run-Time Environment, the Content Aggregation Model (CAM) and the Sequencing and Navigation (SN). The CAM has three parts: the Content Model, the Metadata and Content Packaging. The Content Model describes the relationships between course modules and provides for Sharable Content Objects (SCOs) and assets; the Metadata is used to describe the content into some common vocabulary. The SN governs how navigation between SCOs is handled by the LMS through the definition of rules known as the Sequencing Definition Model [67].
We state only the aspects for which there is uniqueness for the learner-centric tool. We recommend that the learner-centric course delivery tool uses SCORM 2004 with the following:
- The teaching-learning style learning objects (the learning objects per learning objective and learning style) are represented as the (SCORM) assets. [Content Aggregation Model – Content Model]
The SCO is the combination of these assets (learning objects) for a learning objective. A recursive definition would be more appropriate for accomplishing the needs of the learner-centric tool but to achieve reuse, SCORM defines that a SCO is not allowed to reference other SCOs.
- In a grouping of learning objects per learning objective, one or more of these learning objects may or may not be used in an instance of accomplishing the learning objective, based on the learning style(s) of the learners. [Content Aggregation Model – Content Model]
- The Meta-metadata category stores the teaching-learning styles relevant to the object. Its usage is therefore mandatory. [Content Aggregation Model – Metadata]
The usage of the General, Lifecycle, Relation, and Classification categories are optional but recommended. [Content Aggregation Model – Metadata]
- The Sequencing Rules category outlines the workflow between the teaching-learning style learning objects. [Sequencing and Navigation Specification – Sequencing Definition Model]
- The following categories are also mandatory: Rollup Rules, Rollup Controls, Objectives, Delivery Controls and Navigational Controls. Completion Threshold Controls is mandatory for SCORM 2004 4th Edition only. [Sequencing and Navigation Specification – Sequencing Definition Model]
The categories of Sequencing Control Modes, Constraint Choice Controls and Limit Conditions are not mandatory but their usage are advantageous based on the implementation and application of the learner-centric tool.
All other aspects of or necessities for SCORM which tend to be common to all SCORM compliant tools are applied: the LMS launches all content in a web browser and is required to implement an API, all of the relevant course data is stored in a XML file, and all content is to be transferred in a folder or a ZIP file called a package interchange file (PIF) [76, 67].
The Online Learner-Centric Tool (OLeCenT) is designed to interface with a Learning Management System (LMS) (Figure 3), thereby course delivery and administration is still managed by the LMS, but enhanced by the learner-centric delivery provided through OLeCenT.
4.2. Components of OLeCenT
The tool has four components, namely Diagnostic Analysis, Repository and Workflow Setup, Learning Administration, and Learner-Centric Assessment and Evaluation (Figure 4). Diagnostic Analysis allows for the setup of one or more Learning Style Inventories as well as the diagnostic assessments of the teacher(s) and learner(s). Repository and Workflow Setup receives and updates learning objects of different types for a single unit of learning as well as setup a designated workflow of how the learning objects are ordered for delivery of the course content per learning style. Learning Administration generates learner course paths and Assessment and Evaluation provides for formative and summative assessments in consideration of each student’s teaching-learning style.
4.2.1 Diagnostic Analysis
The Diagnostic Analysis component incorporates two (2) major processes, namely Setup Learning Style Inventory (LSI)
and Perform Diagnostic Assessment (Figure 5). The tool is designed to allow for any learning style mechanism that has measurable notations. OLeCenT uses learning style indexes throughout its implementation and therefore translates the terms, codes and notations of specific learning style instruments to the OLeCenT learning style indexes.
The tool provides each teacher and learner with a diagnostic assessment to ascertain the teaching-learning style(s) and preferences; it uses this information to inform the human teacher (where existent) of the learning style make-up of the class and teaching-learning style match or gaps as displayed in Table 3.
The learner-centric tool suggests a learning style or combination of learning styles for each student. The learner is allowed to choose another teaching-learning style in which to provide the course content but the system maintains a record of the determined learning style. In Higher Education Institutions (HEIs) there are group assessments, and learning by collaboration is encouraged. By analyzing the collection of student learning styles within the batch of learners, the tool determines for each student or teacher, suggested lists of group members based on the computation of a compatibility factor with a maximum of 100. The suggested lists are based on similar or dissimilar learning style and preferred group make-up. Figure 6 details the 4-part algorithm for determining teacher or learner compatibility factor. The four (4) parts are: Determine Main User Compatibility Factor, Initialize Selection Arrays per Similarity, Determine Other User Compatibility Factors, and Generate List as per Compatibility Ratios.
Table 3: OLeCenT Diagnostic Teaching-Learning Style Analysis
No. | Learning Style | Dominant | Existing | Teaching Match | |||
Course: COMP1005 | |||||||
1 | Visual Numerical | 14 | 37% | 17 | 22% | Preferred | 1 |
2 | Social Individual | 8 | 21% | 13 | 17% | Practiced | 1 |
3 | Visual Language | 7 | 18% | 4 | 5% | Preferred | 1 |
4 | Auditory Numerical | 3 | 8% | 13 | 17% | Practiced | 1 |
5 | Kinesthetic – Tactile | 3 | 8% | 6 | 8% | ||
6 | Oral Expressiveness | 2 | 5% | 5 | 6% | Practiced | 2 |
7 | Written Expressiveness | 0 | 0% | 6 | 8% | ||
8 | Social Group | 0 | 0% | 6 | 8% | ||
9 | Auditory Language | 0 | 0% | 4 | 5% |
4.2.1.1 Determine Main User Compatibility Factor
The main user compatibility factor is first determined as a benchmark for assessing the other users. Dominant and existing learning styles, and learning assessment scores for each style is obtained ensuring that the dominant learning style is procured with highest priority (1). Three (MAX_LS_PRI) learning style records are procured. The calculated factor is a summation of a maximum score per learning style with a higher weighting (MAX_LS_PRI/i) being allocated for the dominant learning style. The factor of 3 is used as the other users will be compared based on the 3VAK elements.
4.2.1.2 Initialize Selection Arrays per Similarity
A compatibility factor holder will maintain the compatibility factors of all other users. Based on whether the grouping was desired by Similarities or Dissimilarities, the calculated value would be tending away from zero or not respectively. The initialization values ensure that the calculated user values have higher precedence.
4.2.1.3 Determine Other User Compatibility Factors
The dominant and existing learning styles, and learning assessment scores for each style is obtained for each user, ensuring that the dominant learning style is procured with highest priority (1). Three (MAX_LS_PRI) learning style records are procured. The calculated factor is a summation of a maximum score per learning style with a higher weighting (MAX_LS_PRI/i) being allocated for the dominant learning style. A factor of 1 to 3 is used to multiply the learning style score based on the level of similarities of the 3VAK elements of the main user’s learning style and that of the user being determined. As record 3 (MAX_LS_PRI) of each user is ascertained, an insertion sort is applied only maintaining the NOOFDISPLAYNEEDED.
4.2.1.4 Generate List as per Compatibility Ratios
A compatibility factor ratio is determined by considering the variance of the user factor to the main factor, as a ratio of the main factor. The NOOFDISPLAYNEEDED users are shown with compatibility factors in comparison to 100.
Both teacher and learner are tested to determine teaching-learning skills matching. The human teachers for the course are tested and the teaching styles noted for each teacher as being preferred and/or practiced. Learners are tested with the aim of determining the prevalent learning style and the list of all learning styles in the set of learners. These are grouped into categories of dominant and existing learning styles. The teaching and learning styles resulting from the tests are put in a matrix and used to extract the levels of matching.
The learner-centric tool may be designed for any of the sets of learning styles with any Learning Style instrument (LSI). We propose Kolb & Kolb’s Learning Styles Inventory 9-fold classification [51] based on its widespread usage, flexibility and a classification that seems to engulf other LSI. The learning style units are classified based on the Visual- Auditory-Kinesthetic (VAK) content thereby providing ease of relation to learning objects. OLeCenT allows rules for learning style determination to be codifed. Learning Style rules are entered as text; the text is parsed on input to determine a formula for the specific learning style calculation. The parsed calculation is stored. An example of the text inputs is (1):
(Q5+Q13+Q21+Q29+Q37)2 (1)
4.2.2 Repository and Workflow Setup
The tool is able to (1) receive and update learning objects of different types for a single unit of learning as well as (2) setup a designated workflow of how the learning objects are ordered for delivery of the course content. Instructors are allowed to setup a single course learning path per learning style or for all learning styles. Figure 7 reflects these two processes as Maintain Learning Object Learning Style Index(es) and Setup Learning Style Course Path(s) respectively. Within the latter process, the tool also provides an option for setting learning sessions based on input of date, time or date-time ranges.
In view of Learning Object Repositories which exist [77], the Maintain Learning Object Learning Style Index(es) process is not designed as a priority to create new learning objects. In the final implementation, the Repository and Workflow Setup component may work with any SCORM 2004 4th Edition Learning Object Repository. The component ensures that pre-existing learning objects may be viewed, the learning style indexes may be updated, and the user may be informed of the state of each learning object. The state of the learning object includes its SCORM compliance, its OLeCenT readiness in view of learning styles, and other general learning object parameters such as id, title, and objectives. Learning objects (LOs) are tagged for general VAK features and therefore provide the flexibility of being used with multiple LSI without having these tagged to various instruments. Consequently, an institution is provided with the ease of switching between LSIs without having to again setup learning paths or retagging objects.
A design document is produced from the Maintain Learning Object Learning Style Index(es) process to describe the learning objects to be created based on the results from the analysis phase. For each teaching-learning unit (such as material relating to a particular topic of a course), there are specific learning objectives including assessment(s) and corresponding learning objects. For each learning objective, it is determined which teaching-learning style learning objects are to be created to compensate for each teaching-learning style match and unmatched learning style or combination of learning styles. Some
learning styles may require more learning objects than others for optimal learning. It is noted which learning objects per learning objective and learning style, already exist in the repository. Priorities are stated for all non-existent learning objects with greater values being given to those for the unmatched learning styles combinations.
Each type of learning object is specific to a basic teaching-learning style. LMSs have the mechanism for teachers to provide learning content in reusable learning objects. The course designer is able to state the specific unit of course content and the related objects specific to the teaching-learning styles. There is a one-to-many relationship between each unit of course content and the teaching-learning style learning object. The teaching-learning style learning object forms part of the learning process and is specifically designed to support one or more learning styles. The objects are placed in a repository for retrieval at course compilation subject primarily to the learning style course path that has been setup.
The Course Learning Object Workflow is initially setup by the course designer or teacher /instructor but may be updated as the system learns the norms of various users per learning style. Consequently, in the Setup Learning Style Course Path(s) process, the user is provided with an option to enter/modify the Course Path Update Factor and Course Path Automatic Update. The Course Path Update Factor is the numeric ratio where during Learning Administration (OLeCenT Component 3) if a different learner workflow for a particular learning style is followed by the learners that many times when compared to the teacher-entered course learning object workflow, the teacher should be notified/alerted concerning updating the Course Learning Object Workflow for the course for the particular learning style. When alerted, the teacher may manually update the workflow. The Course Path Automatic Update may be set during Setup Learning Style Course Path. If the preferred option is to have automatic update, this may be done and an alert/notification sent to the instructor; the instructor is not provided with the option not to receive notifications in the automatic configuration instance.
4.2.3 Learning Administration
For each learner the tool generates a learner course path (the set of teaching-learning style learning objects designated by the workflow for delivery of the course content), learns which other teaching-learning styles are favoured by specific types of learners and uses this acquired knowledge to enhance the formulated learner course paths (Figure 8).
The learner course path is determined from the learning style for the learner determined from Diagnostic Analysis, as well as the course learning object workflow for each learning style setup by the course designer or teacher during Repository and Workflow Setup. Learning administration is barred from access to the student user without a determined learning style. Having determined the learner course path, the tool provides the teaching-learning style learning objects based on the teacher-designed/approved and/or system-determined workflow and where the learner is at in the scope of learning the course content.
OLeCent attempts to provide a best-fit learning object based on course learning path setup by the instructor and learner’s chosen learning style or determined dominant leaning style. The learner may however choose to view or undertake another path or other relevant but not best fit objects on the assigned course learner path. For fluidity of learning, the tool provides the objects in hypermedia with more than one learning object related to the determined or chosen learning style. It is where the learner chooses to undertake other path(s) or other learning objects on the same path, that the tool learns which other learning styles or path are favoured, preferred or taken by specific types of learners in the delivery of certain sections of the course.
For unmatched learning styles, where the levels indicate that the majority of the learners fall in this grouping, the learning objects are loaded into memory cache. The memory cache is used to optimize data transfers resulting in faster and more efficient data retrieval. Based on the workflow, direction and timing of the teaching-learning units and the learner’s current place in the garnering of the course material, the loading of objects into cache is done at run-time as the learner accesses the course environment. The loading of objects into cache is important primarily where learning objects are large or may significantly increase retrieval time.
The Learning Administration component maintains the path of learning objects, duration and completion percentage for each learner. This is stored within the Learner Learning Object Workflow and is used as the source of data to determine whether an alert should be generated for the course designer or teacher. The Course Learning Object Workflow setup by the course designer or teacher may be updated as the system learns the norms of various users per learning style. Where the Course Path Update Factor and/or Course Path Automatic Update was entered/modified during Repository and Workflow Setup, and where a new learner path was undertaken by the learner, the Update Factor is used to determine whether the new path is used that many times by learners with similar learning style when compared to the teacher-entered course learning object workflow. If these conditions are met, the teacher is notified/alerted concerning updating the Course Learning Object Workflow for the course for the particular learning style.
When alerted, the teacher may manually update the workflow. Where the Course Path Automatic Update is set to the affirmative, the system performs the update automatically. For the update, the teacher-entered course learning object workflow is put in a dormant state, and the new course learning object workflow for the particular learning style is added to Course Learning Object Workflow and made active.
The alerts to enhance the Course Learning Object Workflow, shown in Figure 9 as Learning Administration Exception may be in report form, web page, email or another form of notification. Alert Notifications and Automatic Configuration is determined based on calculated alert, active learning style and change enrolment ratios. The formulae in Figure 9 reflect that the instructor is alerted once the alert percentage exceeds the course update factor. However, when automatic update is on, only where the alert ratio exceeds the average of the active learning style ratio and the change enrolment ratio, is the automatic configuration done. This mitigates the system flopping between decisions where the magnitude of the variance is not significant.
4.2.4 Assessment and Evaluation
The tool will provide for formative and summative assessments in consideration of each student’s teaching-learning style. LMSs have the mechanism for ongoing assessments and some allow the teacher to indicate the level of difficulty for each assessment unit. This feature represents only a modification based on the teaching-learning style; the learner undertakes an assessment based on the determined teaching-learning style.
The Assessment and Evaluation Model (Figure 10) diagrammatically shows that similar to Learning Administration, the learner assessment path is determined from the learning style for the learner determined from Diagnostic Analysis, as well as the course learning object workflow for each learning style setup by the course designer or teacher during Repository and Workflow Setup.
Having determined the learner assessment path, the tool provides the teaching-learning style learning objects based on the workflow and where the learner is at in the scope of learning the course content. The path of learning (assessment) objects, duration and completion percentage for each learner is stored. Similar to Learning Administration, this is stored within the Learner Learning Object Workflow and is used as the source of data to determine whether an Assessment Exception (alert) should be generated for the course designer or teacher. The Assessment and Evaluation component uses the Course Path Update Factor to determine whether the assessment grades is that many times by any learning style when compared to the other learning styles.
5. Implementation and Results
5.1. Development of Tool
OLeCenT is developed using HTML 5, XML, CSS and Javascript for the graphical user interface (client) and PHP for all backend (server) transactions. The database employed is MySQL. All components of OLeCenT use fields of SCORM 2004 4th Edition. Additionally, the Diagnostic Analysis component was developed and tested to be fully SCORM 2004 4th edition compliant.
5.2. Implementation Instance
OLeCenT provides for the setup of a Learning Style Inventory (LSI) or Model by learning style indexes. OLeCenT captures information about each learner and teacher and suggests the dominant and other learning/teaching styles. There is a matching which assigns the learners’ learning styles and the teachers’ teaching styles to a number of the learning styles indexes which matches to the learning styles of the LSI in use. Learning objects are stored in the repository and also given a learning style index or a number of indexes as determined by the course administrator / deliverer / teacher / lecturer. The learners are provided with learning objects in the form of course paths which vary based on the course administrator’s projected path, the learners’ learning styles, and other assigned or chosen course paths of similar learners.
The current instance of OLeCenT allows a user to be defined as one of five possible user types. The user types are Student, Instructor/Lecturer, Course Designer, Administrator and System Administrator. Each user is assigned optionally to a specific department and/or institution. Where a user of type Student or Instructor is not provided with a department or institution, that user is available to be assigned to any course as in the use of OLeCenT for Massive Open Online Courses (MOOCs).
The first three components of OLeCenT were tested with an instantiation including The C.I.T.E. Learning Styles Inventory (http://www.d11.org/Transition/Assessments/ Documents/C.I.T.E.%20Learning%20Styles%20Instrument.pdf) as the Learning Style Instrument (LSI). The C.I.T.E. instrument diagnosed one or more of nine (9) learning styles by use of forty-five questions. For testing purposes only, access to OLeCenT was successfully provided via the Moodle environment, but implementation was not done for full integration which allows for the ease of demographic and course data between the two systems.
Learning Objects were setup as types V – Visual, A – Auditory, K – Kinesthetic, R – Reading/None Specified, and O – Other. Video Files (WMV, MP4, AVI) and Powerpoint Presentation (PPT, PPTX) and Show (PPSX, PPS) without Audio were setup as Visual. Audio Files (MP3,WAV,M4A) and Powerpoint Presentation (PPT, PPTX) and Show (PPSX, PPS) with Audio and Timing were setup as Visual. Articulate Storyline v. 2 Web-enabled Files (HTML) and Powerpoint Presentation (PPT, PPTX) and Show (PPSX, PPS) with Audio and Transition but without Timing were setup as Kinesthetic. PDF, TXT,RTF files were set as Reading. Where learning objects of an auditory type were not readily available, the Windows version of Natural Reader v. 14 was employed for creation of such objects. Natural Reader is a free text to speech software with naturally sounding male and female voices. For the Computing or Math-based courses, Natural Reader was deemed useful primarily for course outlines, rather than actual course content or assessments. Subsequent to the Learning Objects Setup, the Learning path (per learning style or for all learning styles) is also setup by the course designer/instructor.
5.3. Implementation Results
OLeCenT was used in four (4) instances including a focus group of ten (10) students of a private institution administered a MOOC of secondary-level Information Technology, and three computing qualitative and quantitative courses at a tertiary-level institution administered at first year, second year and third year. The first year course COMP1005 Software Engineering Essentials was qualitative and contained over thirty-five (35+) students; the second year course COMP2005 Discrete Mathematics was quantitative and included five (5) students; the third year course INFO3005 Information Systems was qualitative and was administered to 3 students.
5.3.1 Diagnostic – Teaching-Learning Style Match Analysis
The teaching style (TS) – learning style (LS) match may be determined based on the learners’ dominant or practiced styles and the instructors’ teaching approaches. The teaching-learning style matches determined by OleCenT were:
For the first year qualitative course COMP1005
- 38% match – preferred TS and dominant LS with main instructor (Figure 11)
- 57% match – preferred TS and dominant LS with all instructors (Figure 11)
- 90% match – total of preferred and other TS and group’s dominant LS
For the small qualitative course INFO3005
- 33% match – preferred teaching style (TS) and group’s dominant learning style (LS)
- 66% match – total of preferred and other TS and group’s dominant LS
The results also showed in the instance of the quantitative course COMP2005 that the preferred teaching style was matched by only 30% of the group’s dominant learning style. In view of the total of preferred teaching style and other teaching styles, there was a 49% match with the group’s dominant learning style.
The data for all courses proved useful both to the face-to-face and online instructors who were favoured with the information as to how to possibly better reach the batch of learners in teaching.
5.3.1 Diagnostic – Teacher and Learner Group Member Possibilities
Similar and dissimilar learning methods of students may be determined based on a student’s dominant or existing styles, and a comparative review with other dominant and existing styles and scores from the learning style instrument. In view of teaching approaches, similar and dissimilar approaches may also be determined; these are based on a teacher’s preferred or practiced approaches and other similar approaches and comparison of scores from the learning style instrument.Figure 12 displays a student with id number 620099999 with his/her top 5 students with similar styles. OLeCent may also display a teacher with his/her top teacher with dissimilar styles. Top teacher dissimilarity possibilities may be used to ensure that there is greater coverage of the learning styles that are reached in a batch of learners. The similarity check is not restricted to learners/teachers of a similar learning-teaching style. Figure 12 highlights that student 620099999 of dominant learning style Social Individual and other styles of Visual Numerical and Auditory Numerical was deemed to have the fourth highest compatibility factor 80 (of 100) with student 415001546 of dominant style Oral Expressiveness and other styles of Visual Numerical and Auditory Numerical.The similarity and dissimilarity comparisons are based on the relative difference between the main student/teacher being
evaluated and each other student/teacher in the batch of learners/teachers. A compatibility factor for each learner is computed from the relative differences noted among the dominant learning styles, existing styles, and learning styles instrument scores 1, 2 and 3 (Figure 13). For the teacher, compatibility factors are computed from the relative differences noted among the dominant preferred styles/approaches, practiced styles or approaches, and learning styles instrument scores 1, 2 and 3.
The teacher-learner group member possibilities determined by OLeCenT were:
For a student of a particular learning style
- Similar and dissimilar styles
- 70% or greater similarity was found even where the individuals were of different dominant learning styles
For a teacher of a particular teaching approach
A 39% dissimilarity was identified with other teachers/instructors.
5.3.3 Repository – Learning Object Learning Style Gaps
Learning Object Learning Style gaps may be identified per course and per VARK containment. VARK containment describes whether a learning object is deemed to be appealing to the Visual, Auditory, Reading or Kinesthetic learner [53]. The instructor and/or course designer may be immediately notified where learners of a particular style may be required to access the course material in the mode not deemed for his/her highest level of learning.
5.3.4 Learning Path Consistency and Disparity
Chosen learning paths per student per learning style may be identified. The learning path setup by the teacher / course designer may be setup uniquely per leaning style. Subject to the flexibility setup with use of pre-requisites, OLeCenT determines the level of compatibility or disparity between the teacher designed path and the student chosen path. Additionally, the favoured learning VARK objects per learning style are ascertained. For each unit within the learning path, a number of learning objects per visual, auditory, reading and kinesthetic containment may be available. OLeCenT determines the most suitable VARK learning object per unit of the learning path to be displayed dominantly with all other available learning objects for the same unit (of the learning path) subtly displayed.
OLeCenT may generate a learning path consistency and disparity analysis for each course in view of one or more teaching-learning styles, activities (including A-Assessment, B-Lab, F-Field work, G-General, L-Lecture, O-Other, S-Seminar, T-Tutorial), disparity allowance time (length of time the learning object was accessed) for 0 or more minutes, the diagnosed or chosen learning style, and consistency check criterion of learning path or learning object. Figure 14 is the learning path consistency and disparity analysis for COMP1005 for all teaching-learning styles, all activities, where learning objects were accessed for 0 or more minutes, for the diagnosed learning style, and using consistency check criterion being the learning object.
Figure 15 is the learning path consistency and disparity analysis for COMP1005 for all teaching-learning styles, activity type lecture, where learning objects were accessed for 0 or more minutes, for the diagnosed learning style, and using consistency check criterion being the learning object. This diagram may
be used to easily identify the level of consistency and disparity for the various learning styles.
New student learning paths may be auto-configured subject to a minimum threshold and the instructor’s approval. Where there is auto configuration and the minimum threshold met for a particular style, subsequent students of the learning style(s) reconfigured are administered the course content according to the newly learnt path. The minimum threshold is met where the threshold percentage/factor of the students of a particular style chooses a consistent path (contrary to the teacher designed path). For the analysis including all activities, chosen learning path variances met the minimum threshold criterion of 75% (of students within similar learning style) for updates in learner course path; this was evident for Visual Language, Auditory Numerical, Social Individual, Oral Expressiveness (Figure 14). Where the minimum threshold is not met but there is significant disparity for a particular learning style, the notifications to the teacher(s)/instructor(s) were ideal as they could more easily manage the process in view of what learning objects were favoured. There were instances where the assigned teacher paths were followed, especially for students that were of a Kinesthetic-Tactile learning style.
Patterns of path selection per learning style were identified for the larger courses (COMP1005, COMP2005). In the instance for reviewing OLeCenT in which the users were selected similar to that of a focus group, the data showed more inconsistency with unidentified patterns. The patterns were more identifiable where OLeCent was used in the actual class environments.
5.3.5 Usage of OLeCent
Comparing COMP1005 which provides course delivery to 39 students through OLeCenT, and a Moodle instance of INFO3005 which provides similar delivery to 44 students, each environment was setup by the same teacher and course designer with a similar number of learning objects subject to the limitation of the Moodle environment in terms of managing VARK learning objects. OLeCenT reflected 1934 views in the course or 50 views per student, whilst Moodle reflected 3492 views in its course or 70 views per student.
Across two (2) campuses, in view of all students and excluding the maximum and minimum length of time spent accessing OLeCenT, the usage over eleven (11) weeks reflected an average of between twenty-two (22) and fifty-seven (57) hours. The findings are significant as students from the same campus as the main course teacher did not reflect lower usage figures where the main teacher was more easily physically accessible. Considering all students in the course, the average usage for 11 weeks per student at the campus without lecturer was 3,449 minutes or 57 hours. This was comparatively more than the average for students on the same campus as the main lecturer of 2,255 minutes or 38 hours. Where students with the maximum and minimum averages were excluded, the pattern remained the same with average usage of 2,923 minutes or 49 hours versus 1,313 minutes or 22 hours respectively.
A survey of students of the tertiary computing first year course COMP1005 was administered to a class of 39 students. Of the 31 responses (or 79% of all responses) received, 41% of students have used 1 other learning management tool, e.g. Moodle or Blackboard and 55% have used 2 or more other LM tools.
The summary of the survey reflecting OLeCenT usage are included as strengths, weaknesses and other points of concern:
Strengths of OLeCenT
- 93% used OLeCent at least once per week. Internationally: 56% (of students in a course) use LMS daily
- 93% recognized the learning object of different formats and used opportunity to view other formats
- 87% felt that OLeCent was effective for course delivery
- Only 9% felt that OLeCent did not improve learning performance
- Only 3% felt that OLeCent was inefficient
- 58% chose the VARK Learning Object assigned by system
Weaknesses of OLeCenT
- 36% undecided or agreed that OLeCenT was frustrating to use
- 29% said that OLeCenT was not easy to use
Other Points of Concern with respect to OLeCent usage
- 52% undecided whether a system should modify path based on learning (45% agreed to system recalculation of learners’ paths)
29% of respondents were undecided whether OLeCenT could be considered higher in course delivery methodology (48% agreed that OLeCenT was above other learning management tools).
5.4. Benefits and Drawbacks
OLeCenT determines learning course paths based on the determined learning styles. The learning styles are determined through the diagnostic assessment in its first module, Diagnostic Analysis. OLeCenT uses the input of any accepted learning style assessment or inventory model. Whereas this may be a benefit to the flexibility of OLeCenT, the drawback is that two of the other three modules, namely Learning Administration, and Learner-Centric Assessment and Evaluation are dependent on the correct determination of the learners’ learning styles. Course developers may develop only some of the teaching-learning style learning objects recommended by the learner-centric tool and still increase the level of learning. For this to happen, developing learning objects for the teaching-learning style matches is omitted but learners make full use of the teaching provided through the human teacher(s).
Other benefits include:
- Allow codifying of multiple Learning Style instruments
- Allow for learning objects to be tagged for single or multiple learning types and can be used across multiple Learning Style instruments
- Identify disparity in teaching and learning and aids face-to-face teacher in knowing percentage of students with greatest benefit in course delivery
- Allow learner flexibility of receiving course content deemed to be best fit as first priority
- Reshape course path per learning style as it learns of learning preference
- Should cause a skew primarily of the teaching curve and a shifting of the equilibrium point [70] of the teaching-learning process if the knowledge gained is used appropriately.
Amidst these benefits, the learner-centric course delivery tool has a number of drawbacks. These include the extensive possibly additional work for the course developer in providing learning objects for each learning style for each course objective to meet the Visual-Auditory-Kinesthetic (VAK) standard. Providing an electronic solution sometimes requires more resources in terms of time, energy, or skill. There is also always the concern of determining the level of granularity for the teaching-learning style learning objects to ensure maximum usability [77]. Another drawback is that eventually all components of OLeCenT will be SCORM 2004 compliant. Many LMSs and tools especially in some international regions are compliant with SCORM 1.2 [16] and there would be decreased efficiency of the tool if designed to be compliant with SCORM 1.2.
Other drawbacks include:
- Learning and auto-configuration takes place during course delivery and therefore provides greater benefit to latter users
- Where a user’s learning style does not change, as a precautionary measure, that user has to periodically (once per term, per year or greater as deemed fit by the administrator) re-diagnosed for learning style.
6. Conclusion
Higher Education Institutions use Learning Management Systems (LMSs) worldwide by to assist with improvements in course delivery or management. Moodle and Blackboard are predominantly used throughout Higher Education Institutions. The Learning Management System of greater usage has limitations and do not foresee immediate usage of features including sequencing and navigation. Learning styles have been purported by some to have relevance and usage in assisting learning. Researches [10-11] suggest that there may be an optimal level of learning in a given time and nature of the uptake function; we consider the nature of the uptake function to include the learner, learning environment and learning style.
The OLECENT approach considers drawbacks of some of the Learning Management Systems (LMSs). OLeCenT, a proof of concept of the OLECENT approach, is developed to be integrated within these LMSs, thereby enhancing functionalities that were not obtained. OLeCent incorporates teaching approaches / styles and learning mechanism / styles with an aim of ensuring that learner-centric course delivery may be administered thereby increasing the level of learning. Instantiations of OLeCenT have shown benefits to teachers and learners including identifying disparity in teaching-learning and providing a mechanism towards improving online learner-centric course delivery, and allowing the input of one or more course learning paths with real-time learning and automatic reconfiguration of the course path where a new trend or pattern is identified. The OLECENT approach may be adopted within Learning Management Systems or the tool incorporated to enhance learning where there may be physical disabilities.
Conflict of Interest
The authors declare no conflict of interest.
Acknowledgment
Thanks to the University of the West Indies which made the resources possible for this research.
- C. Beckford, E. Mugisa, “OLeCenT, ATool towards Improving Online Learner-Centric Course Delivery” Proceedings of Future Technologies Conference 2016. IEEE (FTC 2016), San Francisco, USA, 2016.
- D. Laurillard, “Rethinking university teaching: A conversational framework for the effective use of learning technologies” Routledge, 2013.
- I. Forsyth, “Teaching and learning materials and the Internet” Routledge, 2014.
- B. Asheim, L. Coenen, J. Vang, “Face-to-face, buzz, and knowledge bases: sociospatial implications for learning, innovation, and innovation policy” Environment and Planning C, 25(5), 655, 2007.
- S. R. Aragon, S. D. Johnson, N. Shaik, “The influence of learning style preferences on student success in online versus face-to-face environments” The American Journal of Distance Education, 16(4), 227-243, 2002.
- I. E. Allen, L. Seaman, “Changing Course: Ten Years of Tracking Online Education in the United States” Sloan Consortium. PO Box 1238, Newburyport, MA 01950, 2013.
- M. Bar-Yam, K. Rhoades, L. Booth Sweeney, J. Kaput, Y. Bar-Yam, “Changes in the Teaching and Learning Process in a Complex Education System” Complex Systems Perspectives on Education and the Education System, New England Complex Systems Institute, 2002.
- S. Kara, “Learning Styles And Teaching Styles: A Case Study In Foreign Language Classroom” Conference of the International Journal of Arts and Sciences 1(20): 77 – 82, 2009.
- G. Sabeh, R. Bahou, N. N. Bacha, M. Nabhani, “A Match or a Mismatch between Student and Teacher Learning Style Preferences” International Journal of English Linguistics, Vol. 1,No., 2011.
- D. R. Krathwohl, B. S. Bloom, B. B. Masia, “Taxonomy of Educational Objectives, the Classification of Educational Goals. Handbook II: Affective Domain” New York: David McKay Co., Inc, 1973.
- L. K. Son, R. Sethi, “Metacognitive control and optimal learning” Cognitive Science, 30(4), 759-774, 2006.
- H. Coates, R. James, G. Baldwin, “A critical examination of the effects of Learning Management Systems on University Teaching and Learning” Tertiary Education and Management 11: 19-36,2005, Springer, 2005.
- A. S. Sife, E. T. Lwoga, C. Sanga, “New technologies for teaching and learning: Challenges for higher learning institutions indeveloping countries” International Journal of Education and Development using ICT > Vol.3, No. 2, 2007.
- D. A. Falvo, B. F. Johnson, “The Use of Learning Management Systems in the United States” TechTrends – March/April 2007, Volume 51, Number 2. 2007.
- P. M. Drinkwater, C. M., Adeline, S. French, K. N. Papamichail, T. Rickards, “Adopting a web-based collaborative tool to support the Manchester method approach to learning” Electronic Journal on e-Learning,2(1), 61-68, 2004.
- C. Beckford, E. Mugisa, “Learning Management Systems – The Current Picture” Proceedings of World Conference on E-Learning in Corporate, Government, Healthcare, and Higher Education, AACE (E-Learn 2014), New Orleans, USA, 2014.
- Hamline University, “Blackboard at Hamline” www.hamline.edu/blackboardhelp/, 2004. Retrieved 2016-01-10.
- Princeton University, “Blackboard Overview” The Trustees of Princeton University. www.princeton.edu/bb/, 2016. Retrieved 2016-06-10.
- S. Overly, “The Download: Blackboard makes first acquisition under CEO Jay Bhatt” The Washington Post. www.washingtonpost.com/business/capitalbusiness/the-download-blackboard-makes-first-acquisition-under-ceo-jay-bhatt/2014/01/16/35e7dc1e-7d5d-11e3-9556-4a4bf7bcbd84_story.html, 2014. Retrieved 2014-09-09.
- Saudi Gazette “Mideast adapts to e-learning technologies” Saudi Gazette. www.saudigazette.com.sa/index.cfm?method=home.regcon&contentid=20130429163468, 2013. Retrieved 2014-09-09.
- Blackboard Inc., “Blackboard Learn Platform – There’s More to Learn” www.blackboard.com/about-us/index.aspx, 2016. Retrieved 2016-06-14.
- R. Riddell, “12 Leaning Management System providers and What They Bring to Classrooms” EducationDIVE. www.educationdive.com/news/12-learning-management-system-providers-and-what-they-bring-to-classrooms/97613/, 2013. Retrieved 2014-09-07.
- Moodle, “Moodle Features” docs.moodle.org, 2016. Retrieved 2016-06-14.
- D. Nagel, “Instructure Creates Free ‘Canvas Network’ for Online Courses” campustechnology.com/articles/2012/11/01/instructure-creates-free-canvas-network-for-online-courses.aspx, 2012. Retrieved 2014-09-10.
- Harvard University, “New School Year, New Course Platform. The President and Fellows of Harvard College” tlt.harvard.edu/news/new-school-year, 2016. Retrieved 2016-06-12.
- Instructure, “Canvas Features” www.instructure.com, 2016. Retrieved 2016-06-01.
- IBL Studios Education “Is Canvas The Next Dominant LMS?” IBL Studios Education, http://iblstudios.com/is-canvas-the-next-dominant-lms/, 2014. Retrieved 2016-06-15.
- K. Winkler, “Instructure Canvas – The Next Dominant Education Platform?” Edukwest, 2014.
- Columbia University, “The Sakai and Canvas Comparison” https://canvas.gsb.columbia.edu/courses/361092/pages/lms-selection-process, 2012. Retrieved 2014-09-09.
- Sakai “SAKAI: Collaboration and Learning Environment For Education” sakaiproject.org, 2016. Retrieved 2016-03-07.
- E. Cheng, R. Kleinberg, S. Kruk, W. Lindsay, D. Steffy, “A Strictly Combinatorial Approach To A University Exam Scheduling Problem” Congress, Numerantium, Vol 167. Winnipeg; Utilitas Mathematica, 2004.
- A. Bickford, “12 Common Complaints about Learning Management Systems” Connect Thinking Pty Ltd., 2013.
- P. F. Bergen, “The State of the Learning Management at The University of Chicago and Beyond” The University of Chicago, https://itservices.uchicago.edu/connect/article/state-learning-management-system-university-chicago-and-beyond, 2013. Retrieved 2014-09-11.
- C. Govender, D. W. Govender, “An Exploratory Study: The effectiveness of a Learning Management System (LMS) in the delivery of a face-to-face programming course” Academia.edu 2014.
- D. Everhart, “Competency Based Learning and Learner-Centric Shifts in Education” Blackboard Inc., 2014.
- T. Halbherr, K. Reuter, D. Schneider, C. Schlienger, T. Piendl, “Making Examinations More Valid, Meaningful And Motivating: The Online Exams Service At Eth Zurich” European University Information Systems (EUNIS), 2014.
- J. Biggs, C. Tang, “Teaching for quality learning at university” McGraw-Hill International, 2011.
- K. Struyven, F. Dochy, S. Janssens, “Students’ perceptions about evaluation and assessment in higher education: A review 1. Assessment & Evaluation in Higher Education” 30(4), 325-341, 2005.
- J. T. Gulikers, T. J. Bastiaens, P. A. Kirschner, “A five-dimensional framework for authentic assessment” Educational Technology Research and Development, 52(3), 67-86, 2004.
- S. M. Wilson, P. L. Peterson, “Theories of learning and teaching: what do they mean for educators?” Washington, DC: National Education Association, 2006.
- J. Savery, “BE VOCAL: Characteristics of Successful Online Instructors” Journal of Interactive Online Learning, Volume 4, Number 2, 2005.
- H. Gardner, “The Unschooled Mind: How Children Think and How Schools Should Teach” Basic Books, 2013.
- J. Sweller, J. Van Merriënboer, F. Paas, “Cognitive load during problem solving: Effects on learning” Cognitive Science (2): 257–285, 1998.
- S. D. Sorden, “The cognitive theory of multimedia learning” Handbook of educational theories, Charlotte, NC: Information Age Publishing, 2012.
- A. H. Maslow, “Motivation and Personality” 2nd. Ed., New York, Harper & Row, 1970.
- D. Hung, “Theories of learning and computer-mediated instructional technologies” Educational Media International, 38(4), 281-287, 2001.
- S. Papert, I. Harel, “Constructionism” Ablex Publishing Corporation, 1991.
- H. Gardner, “Frames of Mind” NY: Basic Books, 1983.
- S. Kara, “Learning Styles And Teaching Styles: A Case Study In Foreign Language Classroom” Conference of the International Journal of Arts and Sciences 1(20): 77 – 82, 2009.
- A. P. Gilakjani, “A Match or Mismatch Between Learning Styles of the Learners and Teaching Styles of the Teachers” I. J. Modern Education and Computer Science, 11, 51-60, 2012.
- A. Kolb, D. Kolb, “Learning styles and learning spaces: Enhancing experiential learning in higher education” Academy of Management Learning and Education, 4(2):193–212, 2005.
- S. Cassidy, “Learning Styles: An overview of theories, models, and measures” Educational Psychology Vol. 24, No. 4, Carfax Publishing, 2004.
- N. Fleming, D. Baume, “Learning Styles Again: VARKing up the right tree!”, Educational Developments, SEDA Ltd, Issue 7.4, 4-7, 2006.
- A. Gilakjani, A. S. Masoumeh, “The Effect of Visual, Auditory, and Kinaesthetic Learning Styles on Language Teaching” International Conference on Social Science and Humanity IPEDR vol.5, IACSIT Press, Singapore, 2011.
- N. Chick, “Learning Styles” Vanderbilt University, Centre for Teaching, cft.vanderbilt.edu/guides-sub-pages/learning-styles-preferences/, 2011. Retrieved 2016-06-04.
- H. F. Silver, R. W. Strong, M. J. Perini, “So each may learn: Integrating learning styles and multiple intelligences” Association for Supervision and Curriculum Development, 1703 North Beauregard Street, Alexandria, VA 22311-1714, 2000.
- D. A. Kolb, R. E. Boyatzis, C. Mainemelis, “Experiential learning theory: Previous research and new directions” Perspectives on thinking, learning, and cognitive styles, 1, 227-247, 2001.
- J. J. Koob, J. Funk, “Kolb’s Learning Style Inventory: Issues of Reliability and Validity”, Sage Publications, 2011.
- K. Cherry, “Kolb’s Learning Styles” About Education, About, 2014.
- C. Manolis, D. Burns, J. David J, R. Assudani, R. Chinta, “Assessing experiential learning styles: A methodological reconstruction and validation of the Kolb Learning Style Inventory” Learning and Individual Differences, Volume 23. Elsevier, 2013.
- E. das Chagas Lemos, L. A. M. do Amaral, L. R. M. Oliveira, “Using Virtual Environments Adapted to Individual Capacities of Build Knowledge: an Approach to Distance Learning Courses” In Conference Proceedings. The Future of Education (p. 97), libreriauniversitaria.it Edizioni, 2016.
- R. Dunn, K. J. Dunn, “Learning Styles/Teaching Styles: Should They… Can They Be Matched?” Association for Supervision and Curriculum Development Journal, 1979.
- D. V. Dear, “Do student-centred learning activities improve learning outcomes on a BTEC Applied Science course in FE?” Journal of Further and Higher Education, 1-10, 2016.
- R. Basturk, “The Effectiveness of Computer-Assisted Instruction in Teaching Introductory Statistics” Educational Technology & Society, 8 (2), 170-178, 2005.
- C. H. Chuan, D. Dinsmore, J. Schmuller, T. Morris, “ An Intelligent Tutoring System for Argument-Making in Higher Education: A Pilot Study” 13th International Conference on Machine Learning and Applications (ICMLA), 2014.
- T. C. Chen, A. S. MdYunus, W. W. Ali, A. Bakar, “Utilization of Intelligent Tutoring Systems (ITS) in mathematics learning” International Journal of Education and Development using ICT, Vol. 4, No. 4, 2008.
- Advanced Distributed Learning (ADL), “SCORM Users Guide for Instructional Designers. Sharable Content Object Reference Model (SCORM®)” 2004 4th Ed. Version 8, Advanced Distributed Learning (ADL), 2011.
- Rustici Software, “Tin Can API: Current Adopters” tincanapi.com/adopters/, 2016. Retrieved 2016-06-15.
- C. Beckford, E. Mugisa, “A Tool for Learner-Centric Course Delivery in e-Learning” In J. Sanchez & K. Zhang (Eds.), Proceedings of World Conference on E-Learning in Corporate, Government, Healthcare, and Higher Education (pp. 2475-2484), Chesapeake, VA: AACE (E-Learn 2010), Orlando, USA, 2010.
- R. Johnsonbaugh, “Discrete Mathematics” Upper Saddle River, NJ: Pearson Prentice Hall, 2009.
- J. C. de Lemos, A. Pelin, J. Quiroz, “The Relationship between Learning Styles and the Computer-based Instruction System PRECALC” Florida International University, 1995.
- K. Laudon, J. Laudon, “Management Information Systems, Managing The Digital Firm” Upper Saddle River, NJ: Pearson Prentice Hall, 2014.
- R. S. Pindyck, D. L. Rubinfeld, “Microeconomics” Needham Heights, MA: Simon & Schuster Custom Publishing, 2009.
- Herridge Group, “Use of traditional Instructional Systems Design Models for eLearning” Herridge Group Inc., 2004.
- R. M. Gagne, L. J. Briggs, “Principles of instructional design” NY: Holt, Rinchart and Winston, Inc., 1974.
- S. Xu, S. Li, “The SCORM Standard and its Application in Web-based Education Resources Building” IEEE International Symposium on IT in Medicine and Education, 2008.
- C. Innis-Allen, E. Mugisa, “A flexible taxonomy of learning objects based on content and media centric approaches to granularity” Seventh IASTED International Conference on Web-based Education, Austria. 275, 2008.